DEDGAT: Dual Embedding of Directed Graph Attention Networks for Detecting Financial Risk
arxiv(2023)
摘要
Graph representation plays an important role in the field of financial risk control, where the relationship among users can be constructed in a graph manner. In practical scenarios, the relationships between nodes in risk control tasks are bidirectional, e.g., merchants having both revenue and expense behaviors. Graph neural networks designed for undirected graphs usually aggregate discriminative node or edge representations with an attention strategy, but cannot fully exploit the out-degree information when used for the tasks built on directed graph, which leads to the problem of a directional bias. To tackle this problem, we propose a Directed Graph ATtention network called DGAT, which explicitly takes out-degree into attention calculation. In addition to having directional requirements, the same node might have different representations of its input and output, and thus we further propose a dual embedding of DGAT, referred to as DEDGAT. Specifically, DEDGAT assigns in-degree and out-degree representations to each node and uses these two embeddings to calculate the attention weights of in-degree and out-degree nodes, respectively. Experiments performed on the benchmark datasets show that DGAT and DEDGAT obtain better classification performance compared to undirected GAT. Also,the visualization results demonstrate that our methods can fully use both in-degree and out-degree information.
更多查看译文
关键词
directed graph attention networks,risk,financial,dual embedding
AI 理解论文
溯源树
样例
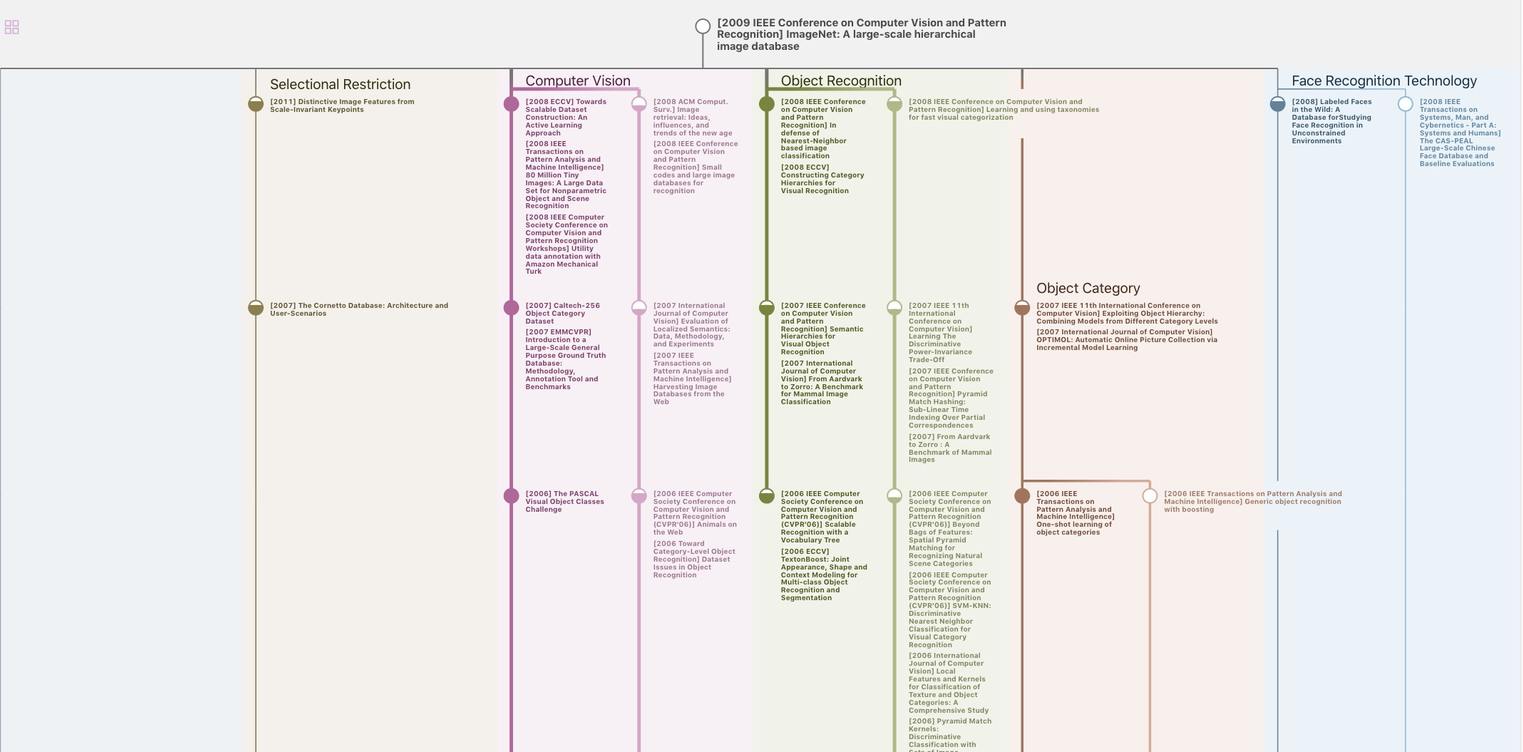
生成溯源树,研究论文发展脉络
Chat Paper
正在生成论文摘要