resVAE ensemble: Unsupervised identification of gene sets in multi-modal single-cell sequencing data using deep ensembles.
Frontiers in cell and developmental biology(2023)
摘要
Feature identification and manual inspection is currently still an integral part of biological data analysis in single-cell sequencing. Features such as expressed genes and open chromatin status are selectively studied in specific contexts, cell states or experimental conditions. While conventional analysis methods construct a relatively static view on gene candidates, artificial neural networks have been used to model their interactions after hierarchical gene regulatory networks. However, it is challenging to identify consistent features in this modeling process due to the inherently stochastic nature of these methods. Therefore, we propose using ensembles of autoencoders and subsequent rank aggregation to extract consensus features in a less biased manner. Here, we performed sequencing data analyses of different modalities either independently or simultaneously as well as with other analysis tools. Our resVAE ensemble method can successfully complement and find additional unbiased biological insights with minimal data processing or feature selection steps while giving a measurement of confidence, especially for models using stochastic or approximation algorithms. In addition, our method can also work with overlapping clustering identity assignment suitable for transitionary cell types or cell fates in comparison to most conventional tools.
更多查看译文
关键词
bioinformatics,deep learning,ensemble,gene set analysis,rank aggregation,single-cell sequencing
AI 理解论文
溯源树
样例
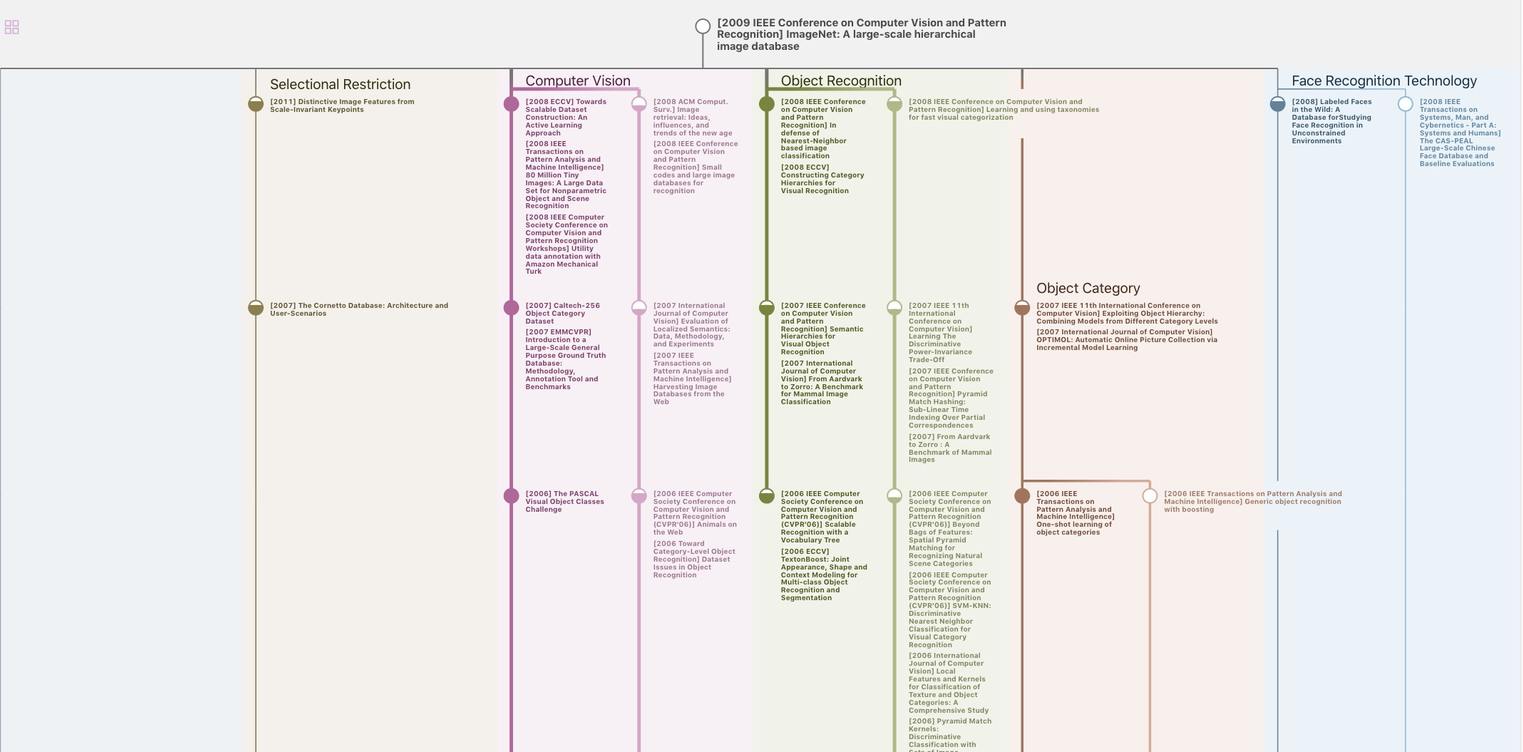
生成溯源树,研究论文发展脉络
Chat Paper
正在生成论文摘要