The Wasserstein Believer: Learning Belief Updates for Partially Observable Environments through Reliable Latent Space Models
arXiv (Cornell University)(2023)
摘要
Partially Observable Markov Decision Processes (POMDPs) are useful tools to model environments where the full state cannot be perceived by an agent. As such the agent needs to reason taking into account the past observations and actions. However, simply remembering the full history is generally intractable due to the exponential growth in the history space. Keeping a probability distribution that models the belief over what the true state is can be used as a sufficient statistic of the history, but its computation requires access to the model of the environment and is also intractable. Current state-of-the-art algorithms use Recurrent Neural Networks (RNNs) to compress the observation-action history aiming to learn a sufficient statistic, but they lack guarantees of success and can lead to suboptimal policies. To overcome this, we propose the Wasserstein-Belief-Updater (WBU), an RL algorithm that learns a latent model of the POMDP and an approximation of the belief update. Our approach comes with theoretical guarantees on the quality of our approximation ensuring that our outputted beliefs allow for learning the optimal value function.
更多查看译文
关键词
belief updates,observable environments,wasserstein believer,learning,models
AI 理解论文
溯源树
样例
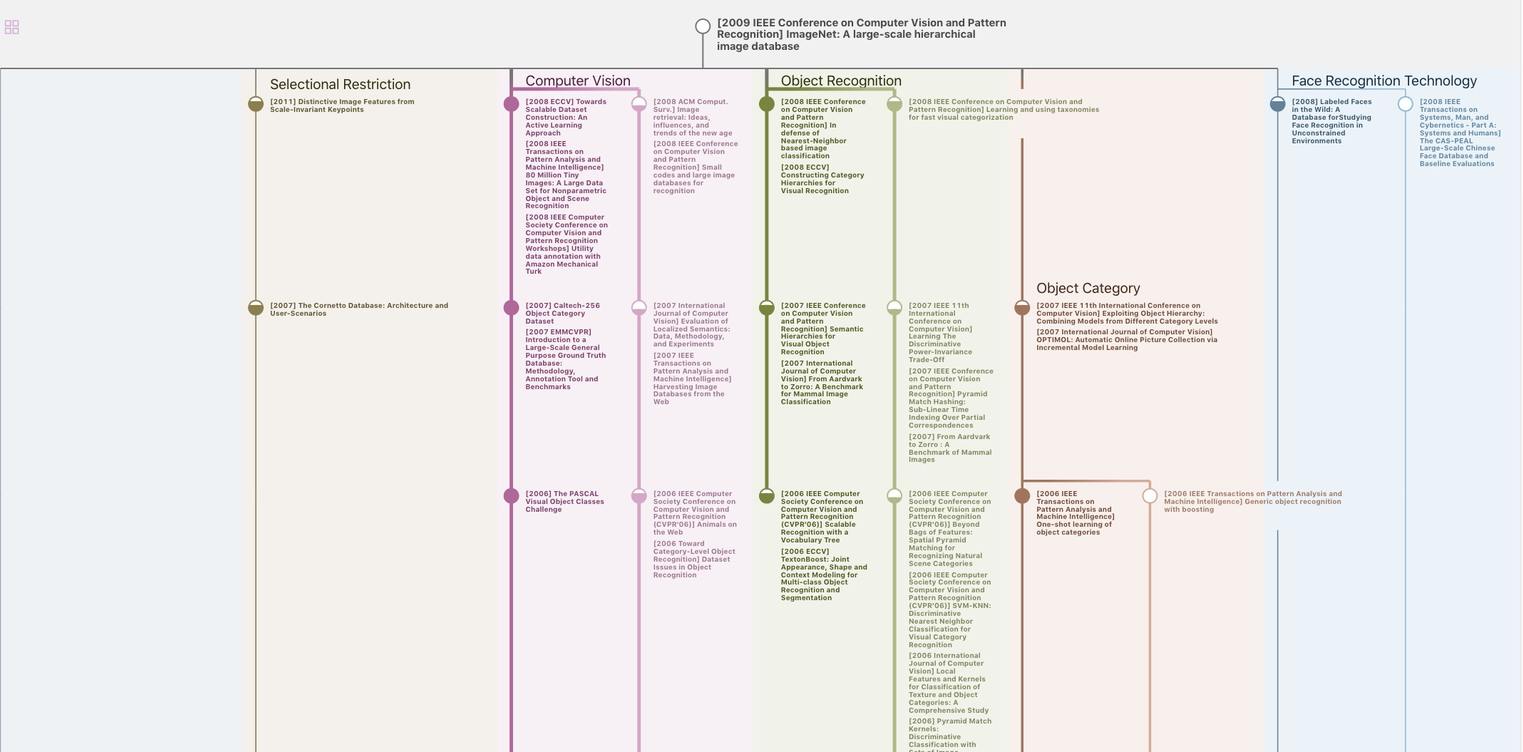
生成溯源树,研究论文发展脉络
Chat Paper
正在生成论文摘要