Pre-trained Model Representations and their Robustness against Noise for Speech Emotion Analysis
arxiv(2023)
摘要
Pre-trained model representations have demonstrated state-of-the-art performance in speech recognition, natural language processing, and other applications. Speech models, such as Bidirectional Encoder Representations from Transformers (BERT) and Hidden units BERT (HuBERT), have enabled generating lexical and acoustic representations to benefit speech recognition applications. We investigated the use of pre-trained model representations for estimating dimensional emotions, such as activation, valence, and dominance, from speech. We observed that while valence may rely heavily on lexical representations, activation and dominance rely mostly on acoustic information. In this work, we used multi-modal fusion representations from pre-trained models to generate state-of-the-art speech emotion estimation, and we showed a 100% and 30% relative improvement in concordance correlation coefficient (CCC) on valence estimation compared to standard acoustic and lexical baselines. Finally, we investigated the robustness of pre-trained model representations against noise and reverberation degradation and noticed that lexical and acoustic representations are impacted differently. We discovered that lexical representations are more robust to distortions compared to acoustic representations, and demonstrated that knowledge distillation from a multi-modal model helps to improve the noise-robustness of acoustic-based models.
更多查看译文
关键词
model representations,emotion,noise,speech,pre-trained
AI 理解论文
溯源树
样例
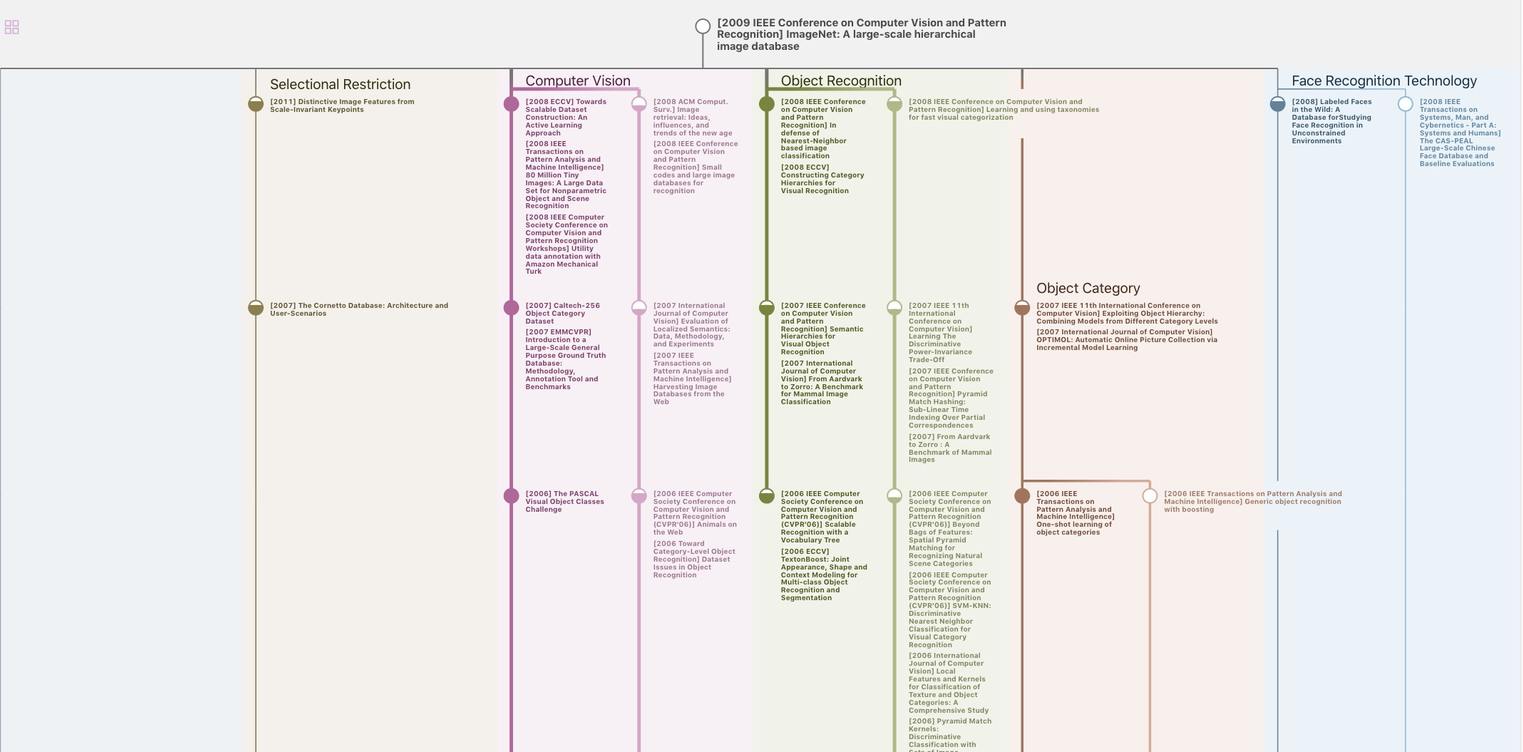
生成溯源树,研究论文发展脉络
Chat Paper
正在生成论文摘要