Critical Points and Convergence Analysis of Generative Deep Linear Networks Trained with Bures-Wasserstein Loss
ICML(2023)
摘要
We consider a deep matrix factorization model of covariance matrices trained with the Bures-Wasserstein distance. While recent works have made important advances in the study of the optimization problem for overparametrized low-rank matrix approximation, much emphasis has been placed on discriminative settings and the square loss. In contrast, our model considers another interesting type of loss and connects with the generative setting. We characterize the critical points and minimizers of the Bures-Wasserstein distance over the space of rank-bounded matrices. For low-rank matrices the Hessian of this loss can theoretically blow up, which creates challenges to analyze convergence of optimizaton methods. We establish convergence results for gradient flow using a smooth perturbative version of the loss and convergence results for finite step size gradient descent under certain assumptions on the initial weights.
更多查看译文
关键词
generative deep linear,networks,critical points,loss,bures-wasserstein
AI 理解论文
溯源树
样例
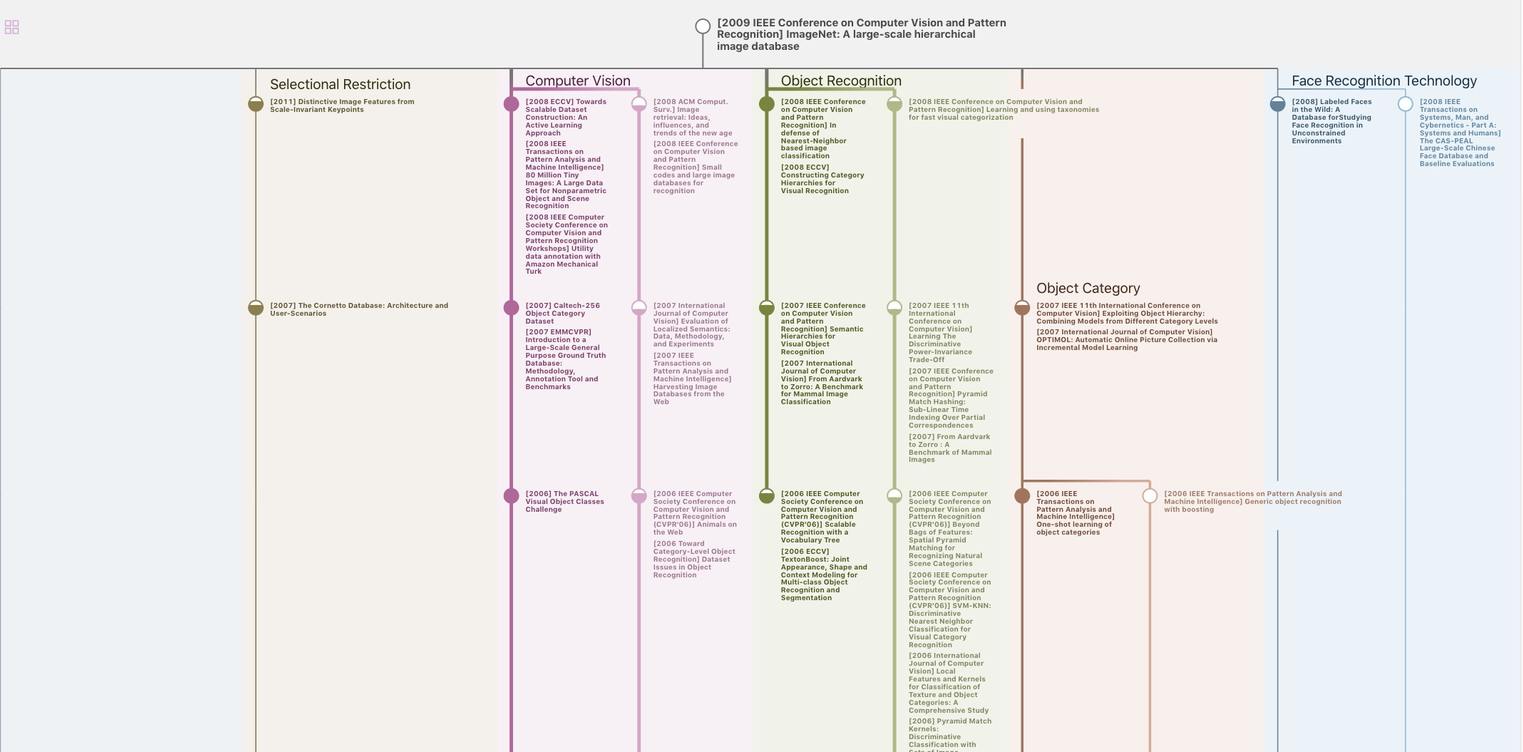
生成溯源树,研究论文发展脉络
Chat Paper
正在生成论文摘要