Scaling strategies for on-device low-complexity source separation with Conv-Tasnet
arxiv(2023)
摘要
Recently, several very effective neural approaches for single-channel speech separation have been presented in the literature. However, due to the size and complexity of these models, their use on low-resource devices, e.g. for hearing aids, and earphones, is still a challenge and established solutions are not available yet. Although approaches based on either pruning or compressing neural models have been proposed, the design of a model architecture suitable for a certain application domain often requires heuristic procedures not easily portable to different low-resource platforms. Given the modular nature of the well-known Conv-Tasnet speech separation architecture, in this paper we consider three parameters that directly control the overall size of the model, namely: the number of residual blocks, the number of repetitions of the separation blocks and the number of channels in the depth-wise convolutions, and experimentally evaluate how they affect the speech separation performance. In particular, experiments carried out on the Libri2Mix show that the number of dilated 1D-Conv blocks is the most critical parameter and that the usage of extra-dilation in the residual blocks allows reducing the performance drop.
更多查看译文
关键词
separation,on-device,low-complexity,conv-tasnet
AI 理解论文
溯源树
样例
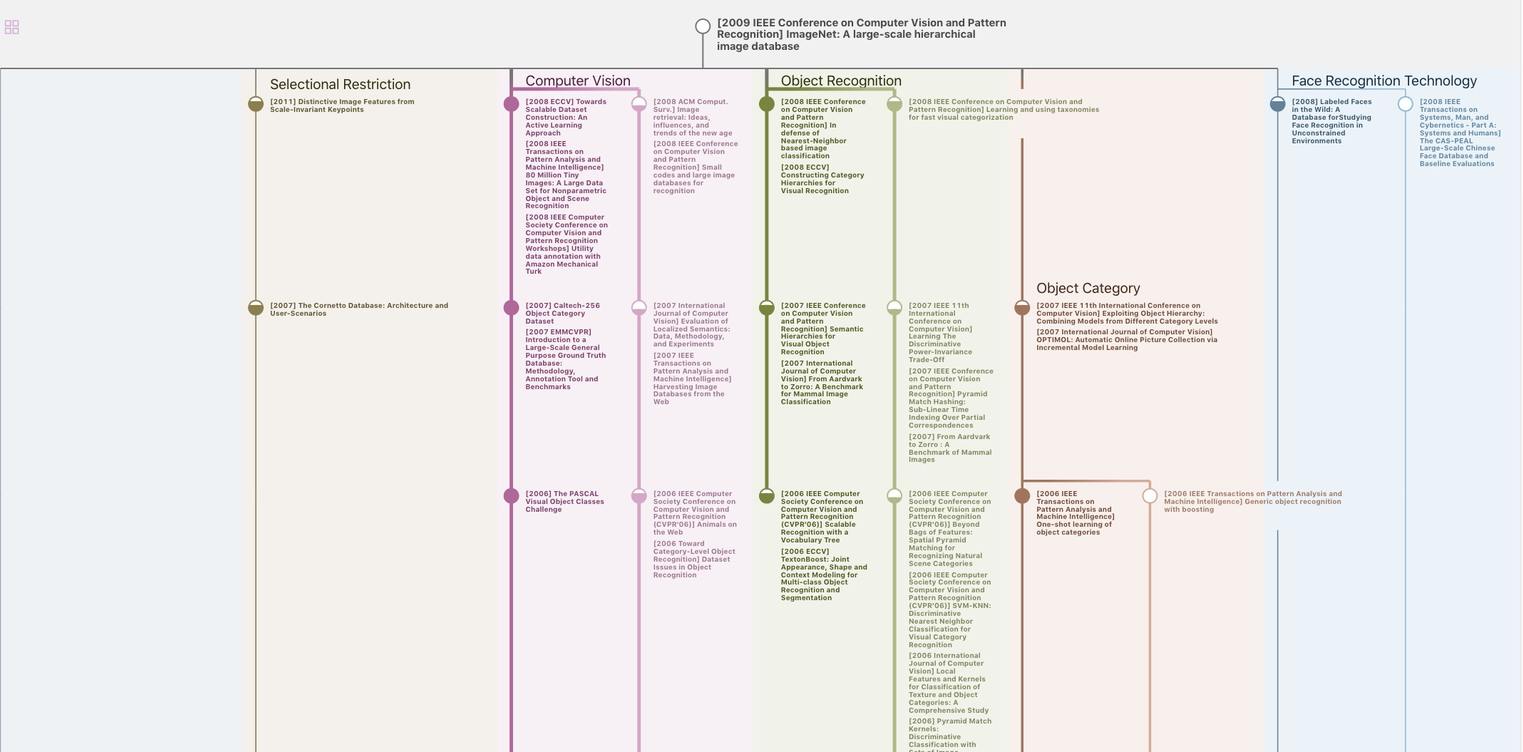
生成溯源树,研究论文发展脉络
Chat Paper
正在生成论文摘要