Adaptive Texture Filtering for Single-Domain Generalized Segmentation
arxiv(2023)
摘要
Domain generalization in semantic segmentation aims to alleviate the performance degradation on unseen domains through learning domain-invariant features. Existing methods diversify images in the source domain by adding complex or even abnormal textures to reduce the sensitivity to domain specific features. However, these approaches depend heavily on the richness of the texture bank, and training them can be time-consuming. In contrast to importing textures arbitrarily or augmenting styles randomly, we focus on the single source domain itself to achieve generalization. In this paper, we present a novel adaptive texture filtering mechanism to suppress the influence of texture without using augmentation, thus eliminating the interference of domain-specific features. Further, we design a hierarchical guidance generalization network equipped with structure-guided enhancement modules, which purpose is to learn the domain-invariant generalized knowledge. Extensive experiments together with ablation studies on widely-used datasets are conducted to verify the effectiveness of the proposed model, and reveal its superiority over other state-of-the-art alternatives.
更多查看译文
关键词
segmentation,filtering,single-domain
AI 理解论文
溯源树
样例
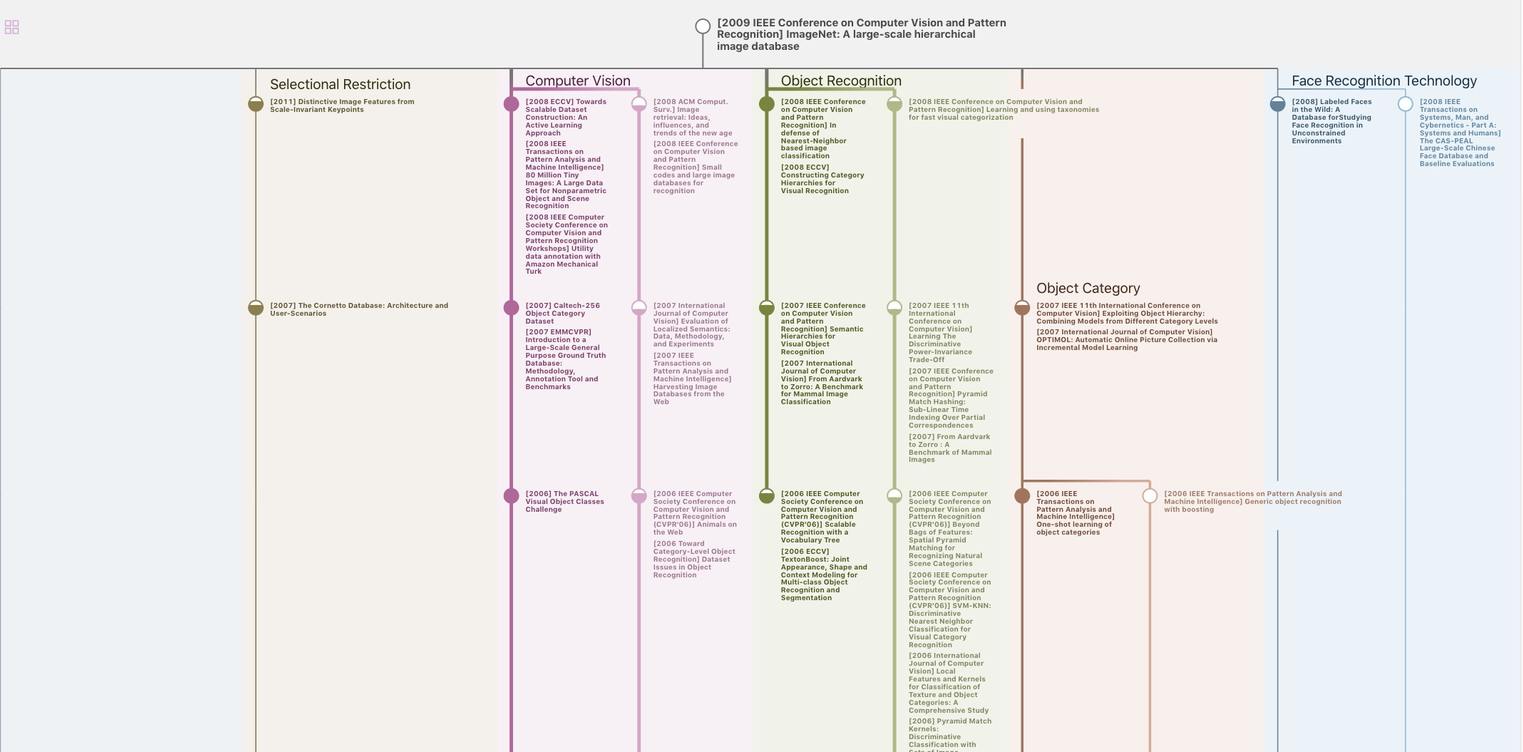
生成溯源树,研究论文发展脉络
Chat Paper
正在生成论文摘要