Scapegoat generation for privacy protection from deepfake
2023 IEEE INTERNATIONAL CONFERENCE ON IMAGE PROCESSING, ICIP(2023)
摘要
To protect privacy and prevent malicious use of deepfake, current studies propose methods that interfere with the generation process, such as detection and destruction approaches. However, these methods suffer from sub-optimal generalization performance to unseen models and add undesirable noise to the original image. To address these problems, we propose a new problem formulation for deepfake prevention: generating a "scapegoat image" by modifying the style of the original input in a way that is recognizable as an avatar by the user, but impossible to reconstruct the real face. Even in the case of malicious deepfake, the privacy of the users is still protected. To achieve this, we introduce an optimization-based editing method that utilizes GAN inversion to discourage deepfake models from generating similar scapegoats. We validate the effectiveness of our proposed method through quantitative and user studies.
更多查看译文
关键词
Deepfake,Adversarial Examples,GAN,Privacy
AI 理解论文
溯源树
样例
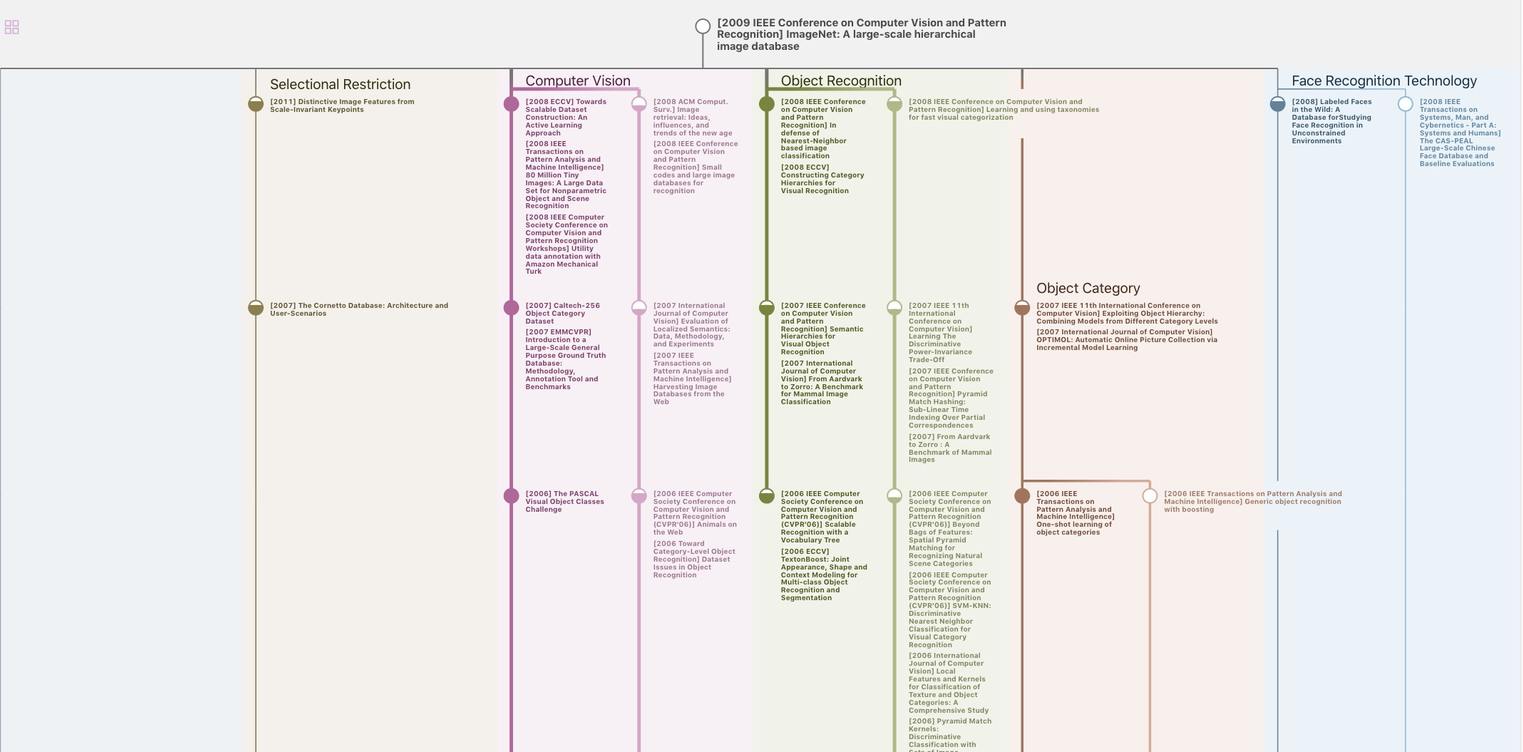
生成溯源树,研究论文发展脉络
Chat Paper
正在生成论文摘要