Privacy-Preserving Fair Item Ranking
arxiv(2023)
摘要
Users worldwide access massive amounts of curated data in the form of rankings on a daily basis. The societal impact of this ease of access has been studied and work has been done to propose and enforce various notions of fairness in rankings. Current computational methods for fair item ranking rely on disclosing user data to a centralized server, which gives rise to privacy concerns for the users. This work is the first to advance research at the conjunction of producer (item) fairness and consumer (user) privacy in rankings by exploring the incorporation of privacy-preserving techniques; specifically, differential privacy and secure multi-party computation. Our work extends the equity of amortized attention ranking mechanism to be privacy-preserving, and we evaluate its effects with respect to privacy, fairness, and ranking quality. Our results using real-world datasets show that we are able to effectively preserve the privacy of users and mitigate unfairness of items without making additional sacrifices to the quality of rankings in comparison to the ranking mechanism in the clear.
更多查看译文
关键词
Ranking, Privacy, Fairness
AI 理解论文
溯源树
样例
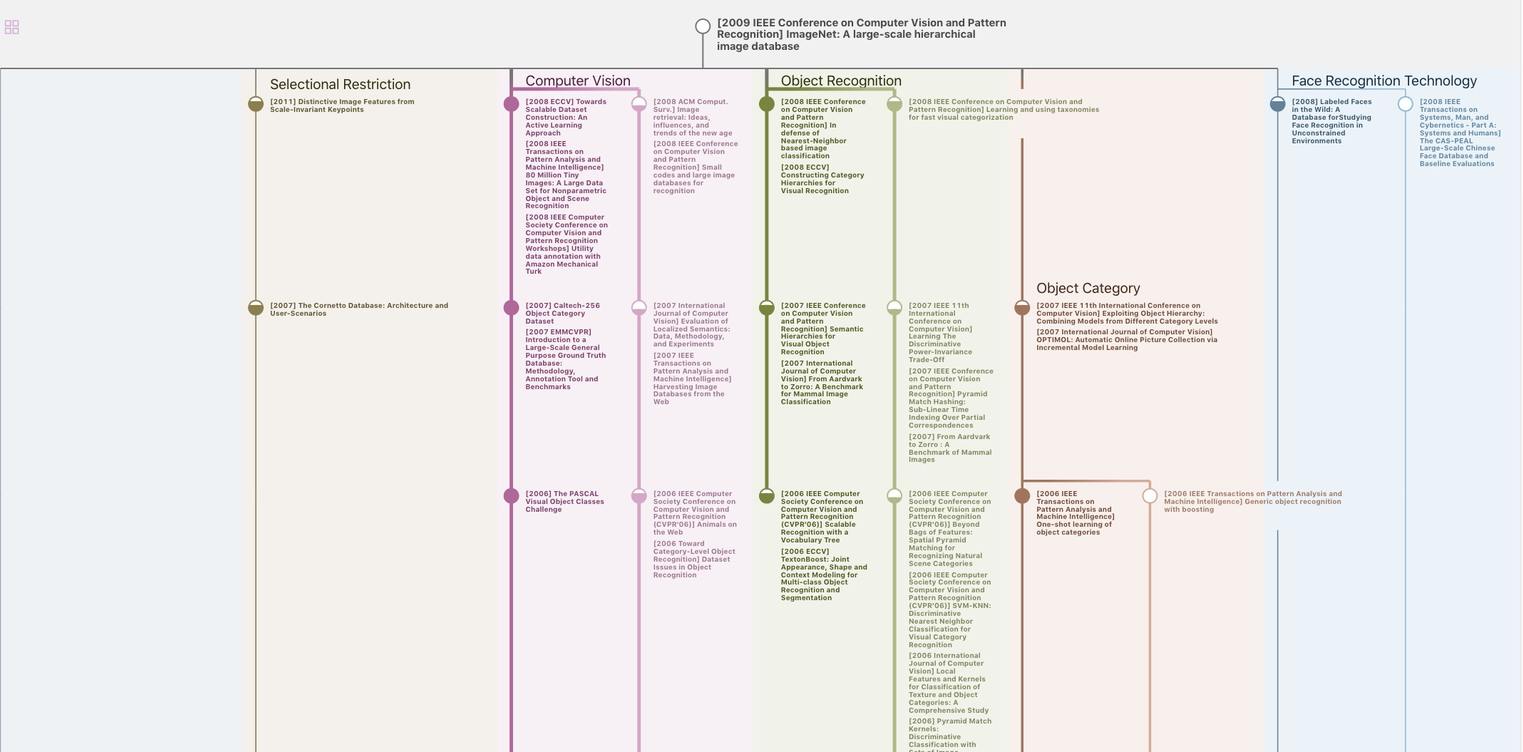
生成溯源树,研究论文发展脉络
Chat Paper
正在生成论文摘要