Efficient and Accurate Range Counting on Privacy-preserving Spatial Data Federation [Technical Report]
arxiv(2023)
摘要
A spatial data federation is a collection of data owners (e.g., a consortium of taxi companies), and collectively it could provide better location-based services (LBS). For example, car-hailing services over a spatial data federation allow end users to easily pick the best offers. We focus on the range counting queries, which are primitive operations in spatial databases but received little attention in related research, especially considering the privacy requirements from data owners, who are reluctant to disclose their proprietary data. We propose a grouping-based technical framework named FedGroup, which groups data owners without compromising privacy, and achieves superior query accuracy (up to 50% improvement) as compared to directly applying existing privacy mechanisms achieving Differential Privacy (DP). Our experimental results also demonstrate that FedGroup runs orders-of-magnitude faster than traditional Secure Multiparty Computation (MPC) based method, and FedGroup even scales to millions of data owners, which is a common setting in the era of ubiquitous mobile devices.
更多查看译文
关键词
accurate range counting,federation,privacy-preserving
AI 理解论文
溯源树
样例
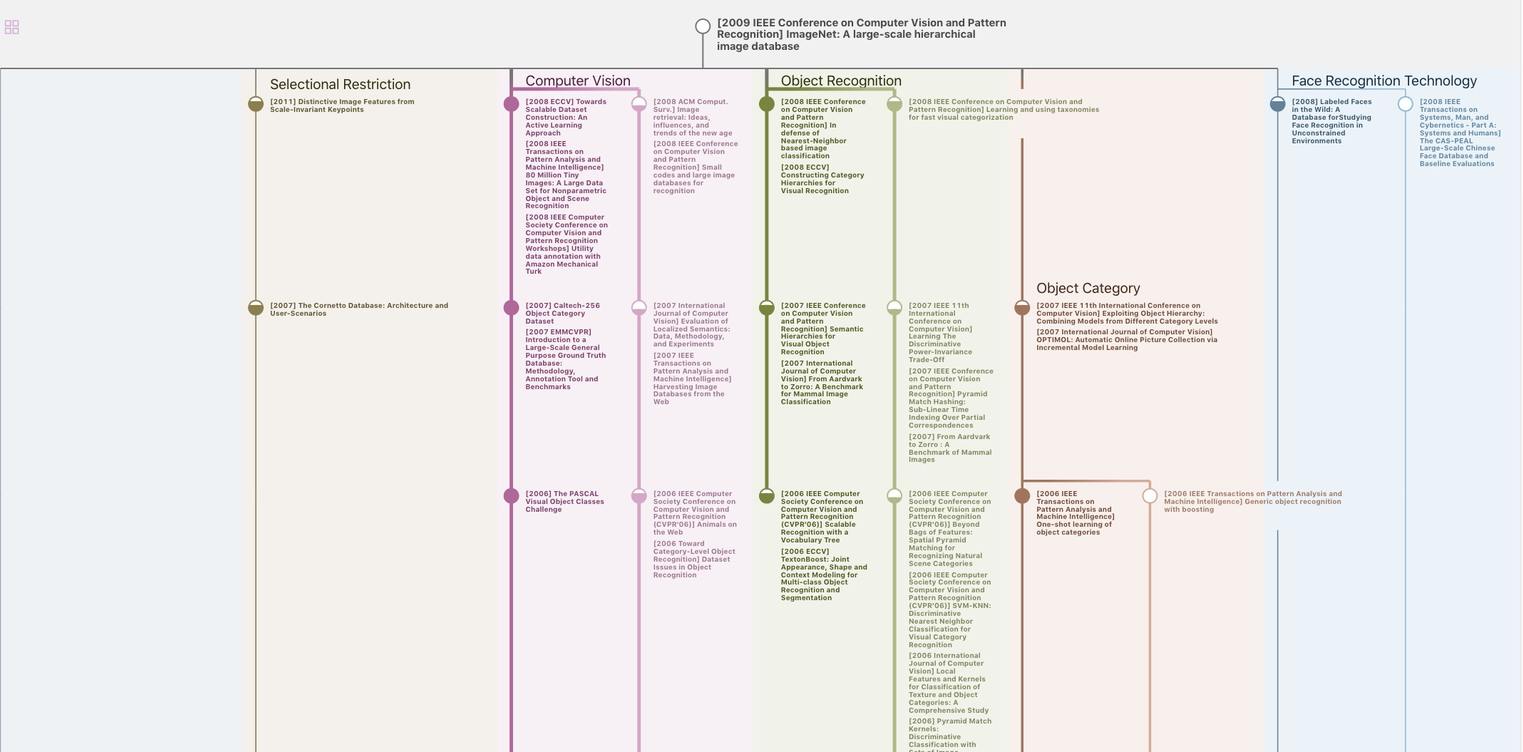
生成溯源树,研究论文发展脉络
Chat Paper
正在生成论文摘要