Diffusion Models Generate Images Like Painters: an Analytical Theory of Outline First, Details Later
arxiv(2023)
Abstract
How do diffusion generative models convert pure noise into meaningful images?
In a variety of pretrained diffusion models (including conditional latent space
models like Stable Diffusion), we observe that the reverse diffusion process
that underlies image generation has the following properties: (i) individual
trajectories tend to be low-dimensional and resemble 2D `rotations'; (ii)
high-variance scene features like layout tend to emerge earlier, while
low-variance details tend to emerge later; and (iii) early perturbations tend
to have a greater impact on image content than later perturbations. To
understand these phenomena, we derive and study a closed-form solution to the
probability flow ODE for a Gaussian distribution, which shows that the reverse
diffusion state rotates towards a gradually-specified target on the image
manifold. It also shows that generation involves first committing to an
outline, and then to finer and finer details. We find that this solution
accurately describes the initial phase of image generation for pretrained
models, and can in principle be used to make image generation more efficient by
skipping reverse diffusion steps. Finally, we use our solution to characterize
the image manifold in Stable Diffusion. Our viewpoint reveals an unexpected
similarity between generation by GANs and diffusion and provides a conceptual
link between diffusion and image retrieval.
MoreTranslated text
AI Read Science
Must-Reading Tree
Example
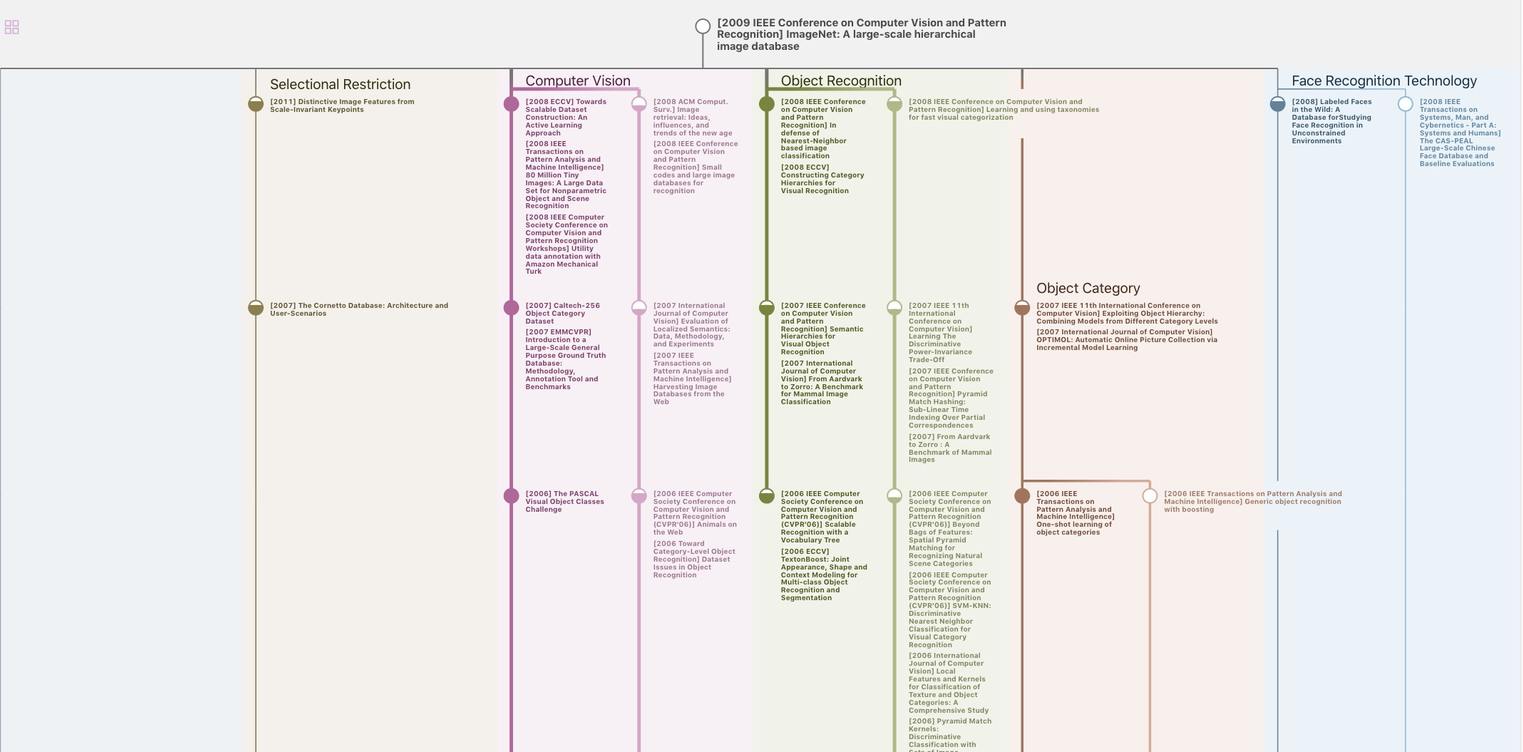
Generate MRT to find the research sequence of this paper
Chat Paper
Summary is being generated by the instructions you defined