Physics-Informed Deep Learning for Traffic State Estimation: A Survey and the Outlook
arxiv(2023)
摘要
For its robust predictive power (compared to pure physics-based models) and sample-efficient training (compared to pure deep learning models), physics-informed deep learning (PIDL), a paradigm hybridizing physics-based models and deep neural networks (DNNs), has been booming in science and engineering fields. One key challenge of applying PIDL to various domains and problems lies in the design of a computational graph that integrates physics and DNNs. In other words, how the physics is encoded into DNNs and how the physics and data components are represented. In this paper, we offer an overview of a variety of architecture designs of PIDL computational graphs and how these structures are customized to traffic state estimation (TSE), a central problem in transportation engineering. When observation data, problem type, and goal vary, we demonstrate potential architectures of PIDL computational graphs and compare these variants using the same real-world dataset.
更多查看译文
关键词
physics-informed deep learning (PIDL),computational graph,uncertainty quantification
AI 理解论文
溯源树
样例
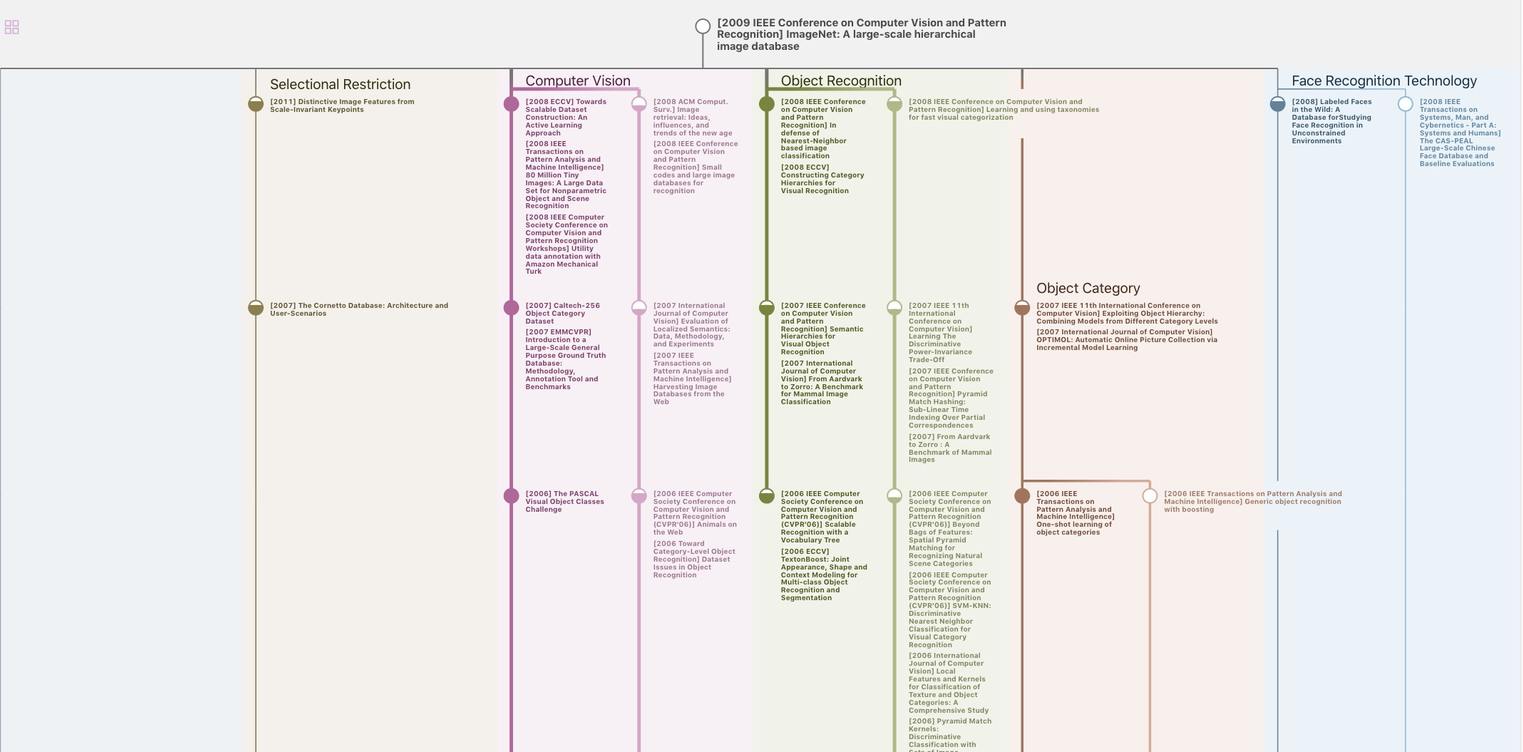
生成溯源树,研究论文发展脉络
Chat Paper
正在生成论文摘要