Uncertainty Estimation by Fisher Information-based Evidential Deep Learning
arxiv(2023)
摘要
Uncertainty estimation is a key factor that makes deep learning reliable in practical applications. Recently proposed evidential neural networks explicitly account for different uncertainties by treating the network's outputs as evidence to parameterize the Dirichlet distribution, and achieve impressive performance in uncertainty estimation. However, for high data uncertainty samples but annotated with the one-hot label, the evidence-learning process for those mislabeled classes is over-penalized and remains hindered. To address this problem, we propose a novel method, Fisher Information-based Evidential Deep Learning ($\mathcal{I}$-EDL). In particular, we introduce Fisher Information Matrix (FIM) to measure the informativeness of evidence carried by each sample, according to which we can dynamically reweight the objective loss terms to make the network more focused on the representation learning of uncertain classes. The generalization ability of our network is further improved by optimizing the PAC-Bayesian bound. As demonstrated empirically, our proposed method consistently outperforms traditional EDL-related algorithms in multiple uncertainty estimation tasks, especially in the more challenging few-shot classification settings.
更多查看译文
关键词
evidential deep
AI 理解论文
溯源树
样例
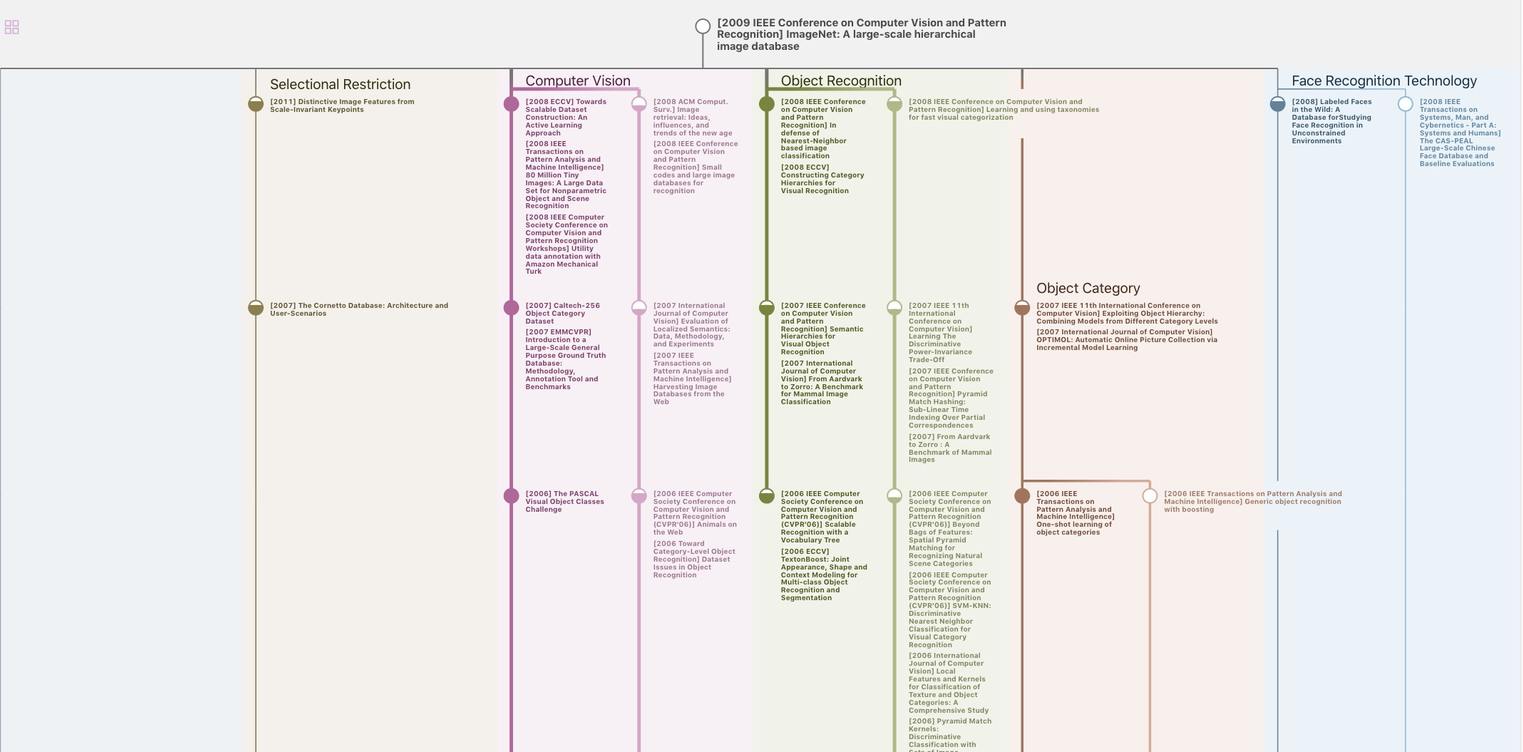
生成溯源树,研究论文发展脉络
Chat Paper
正在生成论文摘要