Hierarchical Graph Neural Networks for Particle Track Reconstruction
CoRR(2023)
Abstract
We introduce a novel variant of GNN for particle tracking called Hierarchical Graph Neural Network (HGNN). The architecture creates a set of higher-level representations which correspond to tracks and assigns spacepoints to these tracks, allowing disconnected spacepoints to be assigned to the same track, as well as multiple tracks to share the same spacepoint. We propose a novel learnable pooling algorithm called GMPool to generate these higher-level representations called "super-nodes", as well as a new loss function designed for tracking problems and HGNN specifically. On a standard tracking problem, we show that, compared with previous ML-based tracking algorithms, the HGNN has better tracking efficiency performance, better robustness against inefficient input graphs, and better convergence compared with traditional GNNs.
MoreTranslated text
Key words
hierarchical graph neural networks,particle
AI Read Science
Must-Reading Tree
Example
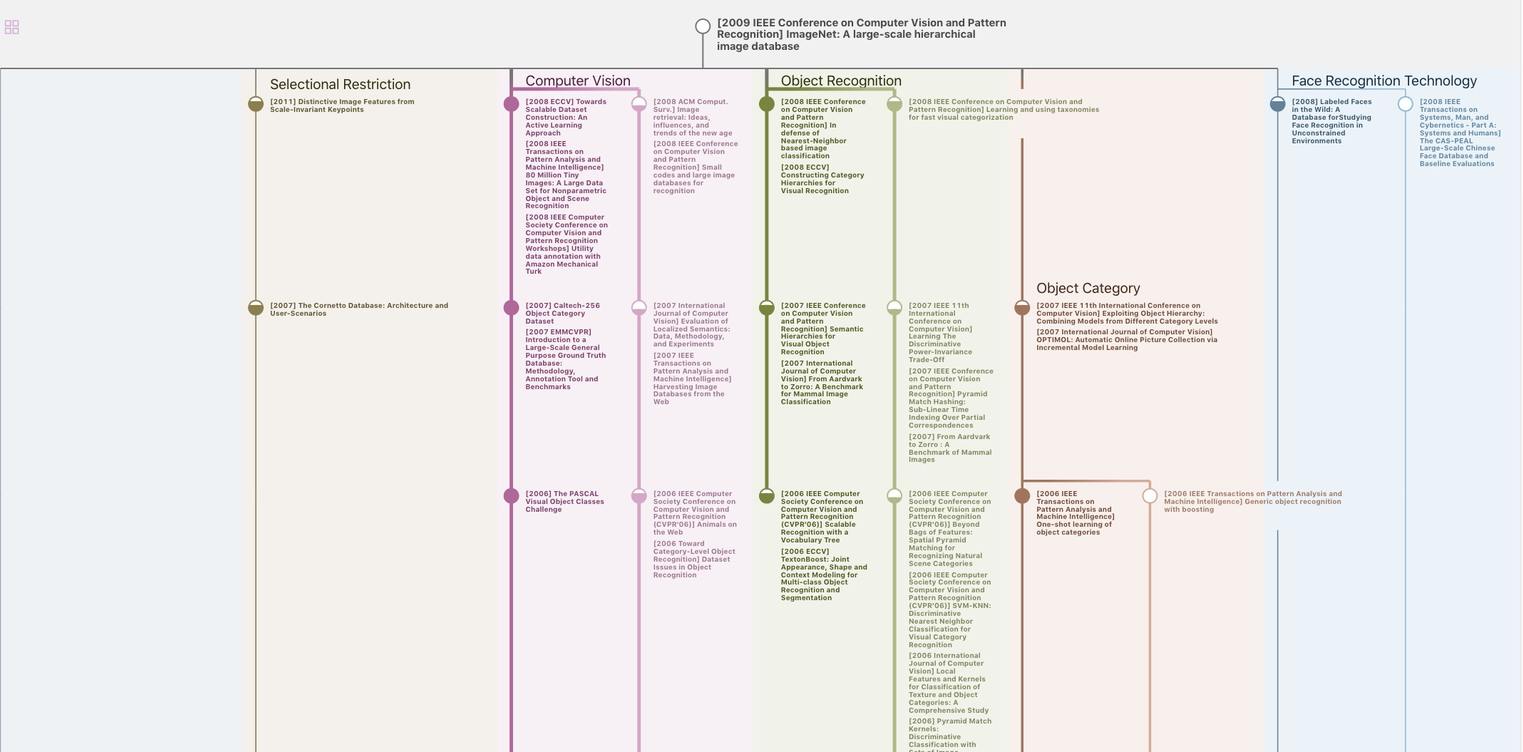
Generate MRT to find the research sequence of this paper
Chat Paper
Summary is being generated by the instructions you defined