Development and External Validation of a Machine Learning Model for Prediction of Lymph Node Metastasis in Patients with Prostate Cancer
EUROPEAN UROLOGY ONCOLOGY(2023)
摘要
Background: Pelvic lymph node dissection (PLND) is the gold standard for diagnosis of lymph node involvement (LNI) in patients with prostate cancer. The Roach formula, Memorial Sloan Kettering Cancer Center (MSKCC) calculator, and Briganti 2012 nomogram are elegant and simple traditional tools used to estimate the risk of LNI and select patients for PLND.Objective: To determine whether machine learning (ML) can improve patient selection and outperform currently available tools for predicting LNI using similar readily available clinicopathologic variables. Design, setting, and participants: Retrospective data for patients treated with surgery and PLND between 1990 and 2020 in two academic institutions were used.Outcome measurements and statistical analysis: We trained three models (two logistic regression models and one gradient-boosted trees-based model [XGBoost]) on data provided from one institution (n = 20 267) with age, prostate-specific antigen (PSA) levels, clinical T stage, percentage positive cores, and Gleason scores as inputs. We externally validated these models using data from another institution (n = 1322) and compared their performance to that of the traditional models using the area under the receiver operating characteristic curve (AUC), calibration, and decision curve analysis (DCA).Results and limitations: LNI was present in 2563 patients (11.9%) overall, and in 119 patients (9%) in the validation data set. XGBoost had the best performance among all the models. On external validation, its AUC outperformed that of the Roach formula by 0.08 (95% confidence interval [CI] 0.042-0.12), the MSKCC nomogram by 0.05 (95% CI 0.016-0.070), and the Briganti nomogram by 0.03 (95% CI 0.0092-0.051; all p < 0.05). It also had better calibration and clinical utility in terms of net benefit on DCA across relevant clinical thresholds. The main limitation of the study is its retrospec-tive design. Conclusions: Taking all measures of performance together, ML using standard clinico-pathologic variables outperforms traditional tools in predicting LNI.Patient summary: Determining the risk of cancer spread to the lymph nodes in patients with prostate cancer allows surgeons to perform lymph node dissection only in patients who need it and avoid the side effects of the procedure in those who do not. In this study, we used machine learning to develop a new calculator to predict the risk of lymph node involvement that outperformed traditional tools currently used by oncologists.(c) 2023 European Association of Urology. Published by Elsevier B.V. All rights reserved.
更多查看译文
关键词
Prostate cancer,Lymph node metastasis,Machine leaning
AI 理解论文
溯源树
样例
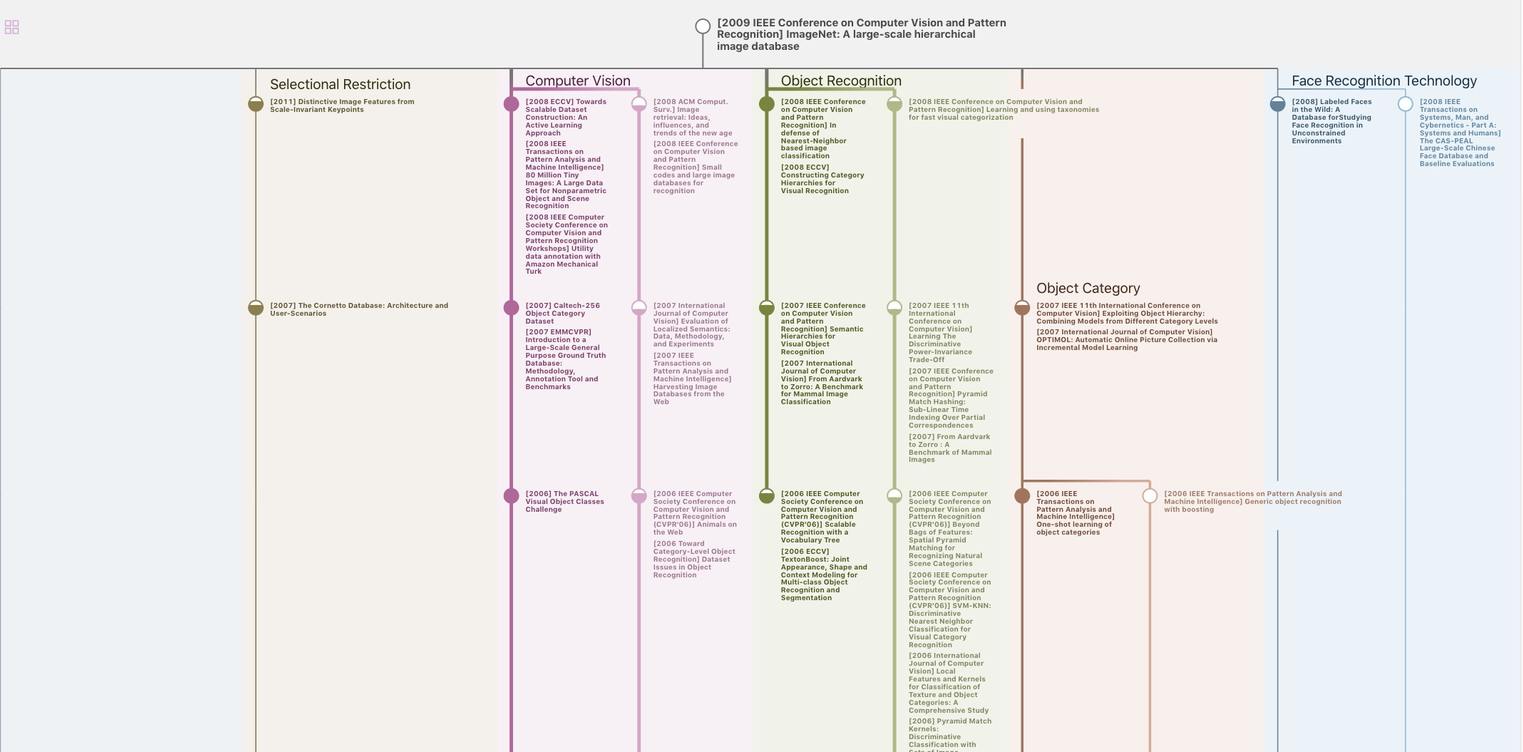
生成溯源树,研究论文发展脉络
Chat Paper
正在生成论文摘要