Multiclass Classification of GvHD and Relapse: A Comparative Analysis Among Machine Learning and ANN-based Algorithms
2022 25th International Conference on Computer and Information Technology (ICCIT)(2022)
摘要
Hematopoietic Stem Cell Transplantation (HSCT) is the most effective therapeutic option or the only chance for a full recovery when the immune system or bone marrow is damaged. Many different malignant and nonmalignant disorders, including leukemia, lymphoma, hematologic malignancies, lymphoid tumors, and many more, have been treated with this technique. But the major drawback of this HSCT is Graft versus Host Disease (GvHD) and sometimes the recurrence of the blood disease, which is popularly known as relapse. In addition, a patient may undergo relapse coupled with acute graft versus host disease (aGvHD) or chronic graft versus host disease (cGvHD), which are the two possible kinds of GvHD, and things get more challenging when a patient experiences a relapse along with any types of GvHD. To effectively treat patients and create follow-up care plans, predicting relapse and GvHD is essential before HSCT. In this study, a multiclass target feature is created to predict potential GvHD and relapse pairings using only three and six crucial attributes from the original dataset, and a comparative analysis is performed between them. For the multiclass classification, this study utilizes several Machine learning algorithms, including the Multilayer Perceptron (MLP), a prevalent classical form of artificial neural network. This study demonstrates that the Extra Trees Classifier algorithm achieves the highest accuracy of 94.45% and 95.34%, and its area under the ROC curve (AUC) score is approximately 98.06% and 99.21% using three and six features, respectively. This model is more useful as a diagnostic tool since it predicts multiclass target features and has lower feature dependence with excellent accuracy.
更多查看译文
关键词
GvHD,Relapse,Multiclass,Feature Engineering,Machine Learning
AI 理解论文
溯源树
样例
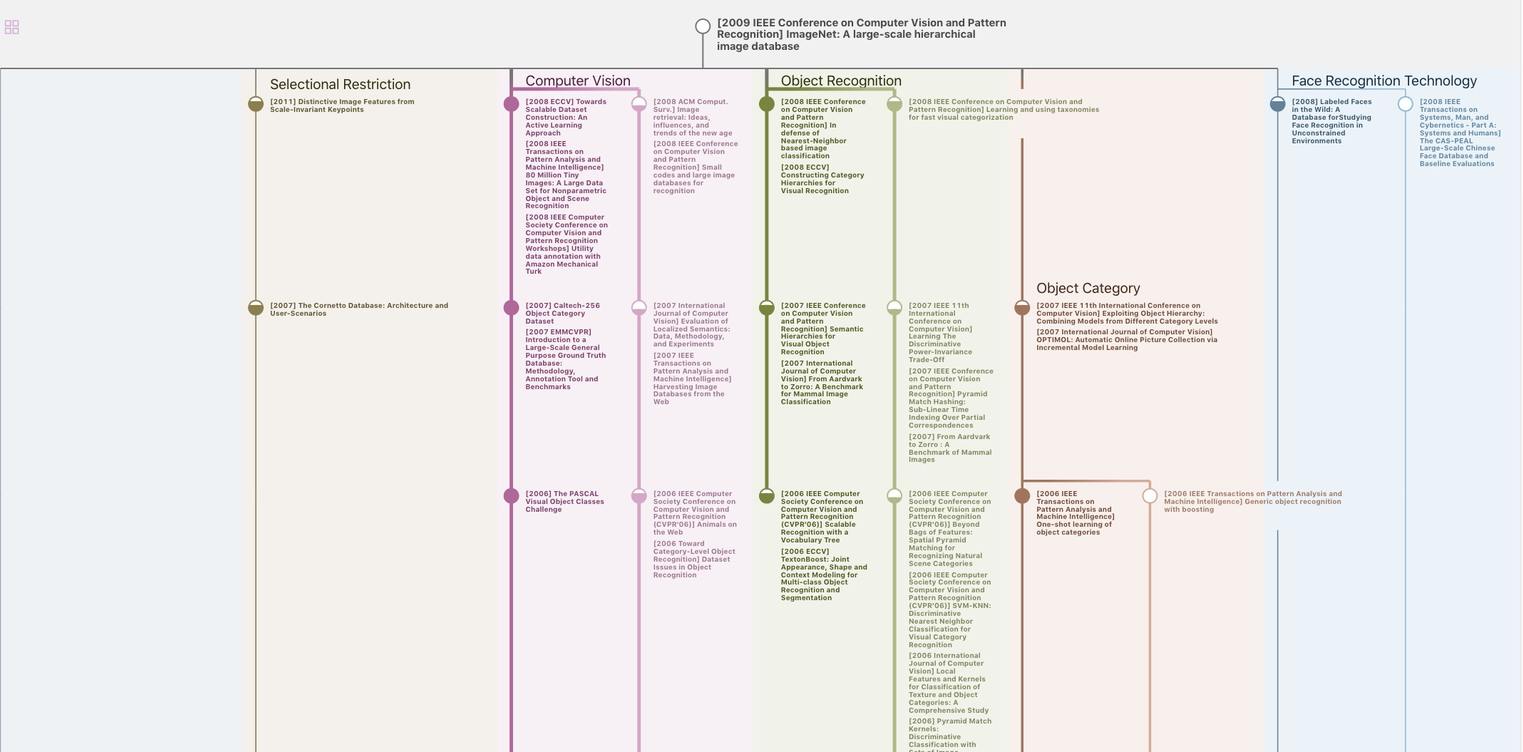
生成溯源树,研究论文发展脉络
Chat Paper
正在生成论文摘要