Deep Learning at the Physical Layer for Adaptive Terahertz Communications
IEEE Transactions on Terahertz Science and Technology(2023)
摘要
Wireless communications in the terahertz (THz) band will become a cornerstone of sixth-generation (6G) networks. The THz channel, however, presents several challenges, such as distance-dependent absorption coefficients that can change the bandwidth significantly in case of mobility. Thus, future THz transmitters will have to switch modulation and bandwidth almost continuously. Moreover, using the same transmission scheme can enable adversaries to leverage smart interfering to inflict more damage with less energy expense. To help enable adaptive and secure THz communications, this article presents the first ever experimental study of modulation and bandwidth classification (MBC) at THz frequencies through deep learning (DL) techniques. We have performed an extensive experimental data collection campaign at 120 GHz with different modulation schemes, signal bandwidth (up to 20 GHz), and different signal-to-noise ratio (SNR) levels. We prove for the first time the feasibility and effectiveness of MBC at THz frequencies, with our DL models reaching accuracy up to 78% and 90% in low- and high-SNR conditions. Furthermore, we investigate the memory and latency constraints that need to be satisfied as a function of the signal bandwidth, and propose a boosting technique to improve the inference quality by trading off latency for accuracy. Finally, we experimentally evaluate the latency of our CNN models through FPGA implementation.
更多查看译文
关键词
Deep learning (DL),experiments,modulation and bandwidth classification (MBC),sixth generation (6G) terahertz (THz) communications,wireless
AI 理解论文
溯源树
样例
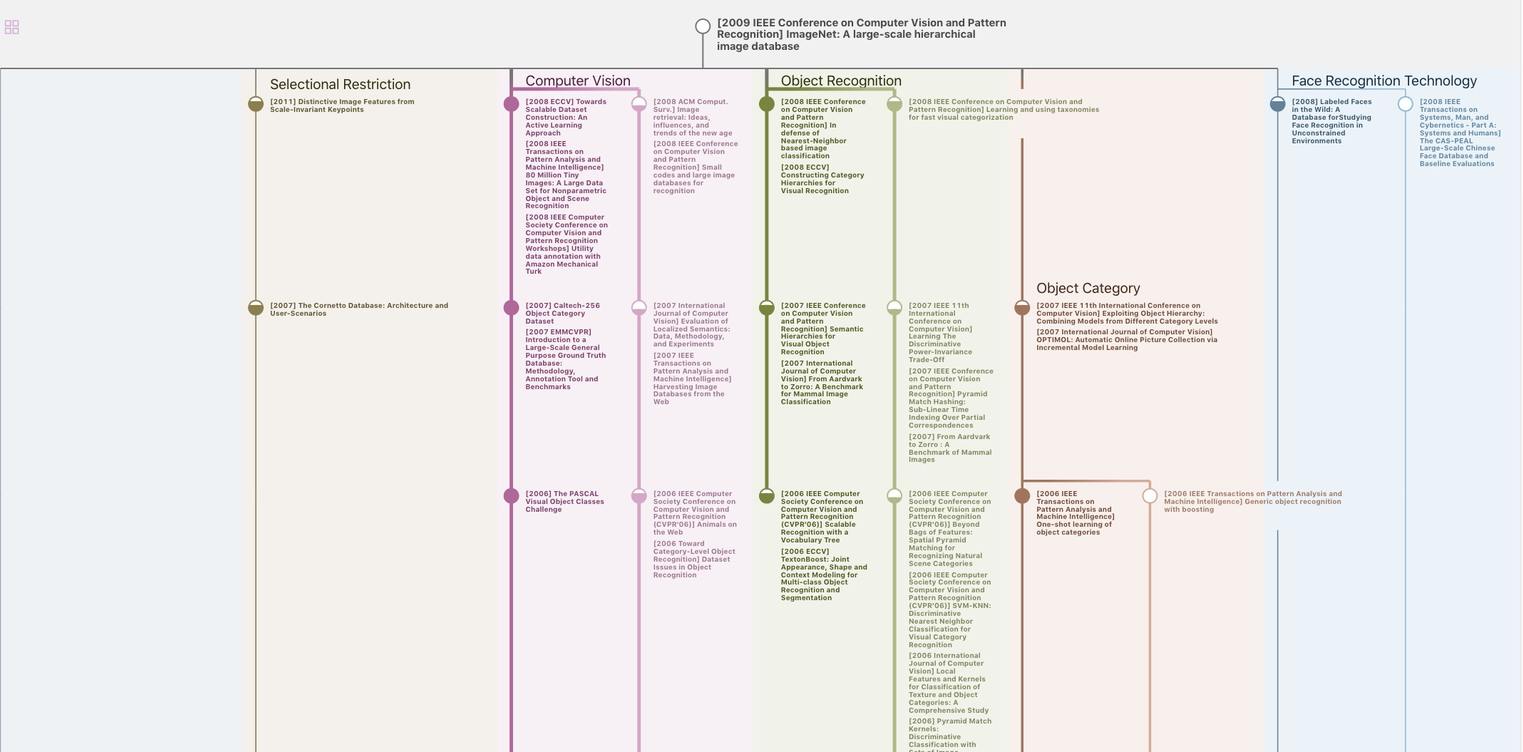
生成溯源树,研究论文发展脉络
Chat Paper
正在生成论文摘要