Machine learning model to predict the efficiency of thermal conductivity of oxide nanofluids
WAVES IN RANDOM AND COMPLEX MEDIA(2023)
Abstract
This article gathers a comprehensive experimental dataset to obtain the efficiency of the thermal conductivity of oxide nanoparticles with the variations in particle size, particle volume concentration, and suspension temperature using Machine learning approach. ML algorithms can be explored to design and develop trans-disciplinary predictive or forecasting models to enhance the existing system performances and efficiencies. Because of the efficiency of the proposed model, it provides several applications in various areas in industries, biomedical, etc. The present investigation is expected to be a Gradient Boosting Regression (GBR), and a new approach that overrides the 'Artificial Neural Network' (ANN). It is presented by considering a data set of 240 using the Koo-Kleinsture-Li (KKL) model conductivity of Al2O3 and CuO-water-based nanofluids. Further, the computation of 'Mean Absolute Error' (MAE), 'Root Mean Squared Error' (RMSE), and the 'Coefficient of Determination' (COD) is obtained and compared with five different approaches that validate the performances of the present technique. It is concluded that the GBR Model is proven as one of the powerful promising approaches over the other approaches used for the predictive enhancements in the performances of several inputs.
MoreTranslated text
Key words
Machine learning,oxide nanofluids,thermal efficiency of KKL model,gradient boosting regression,error analysis
AI Read Science
Must-Reading Tree
Example
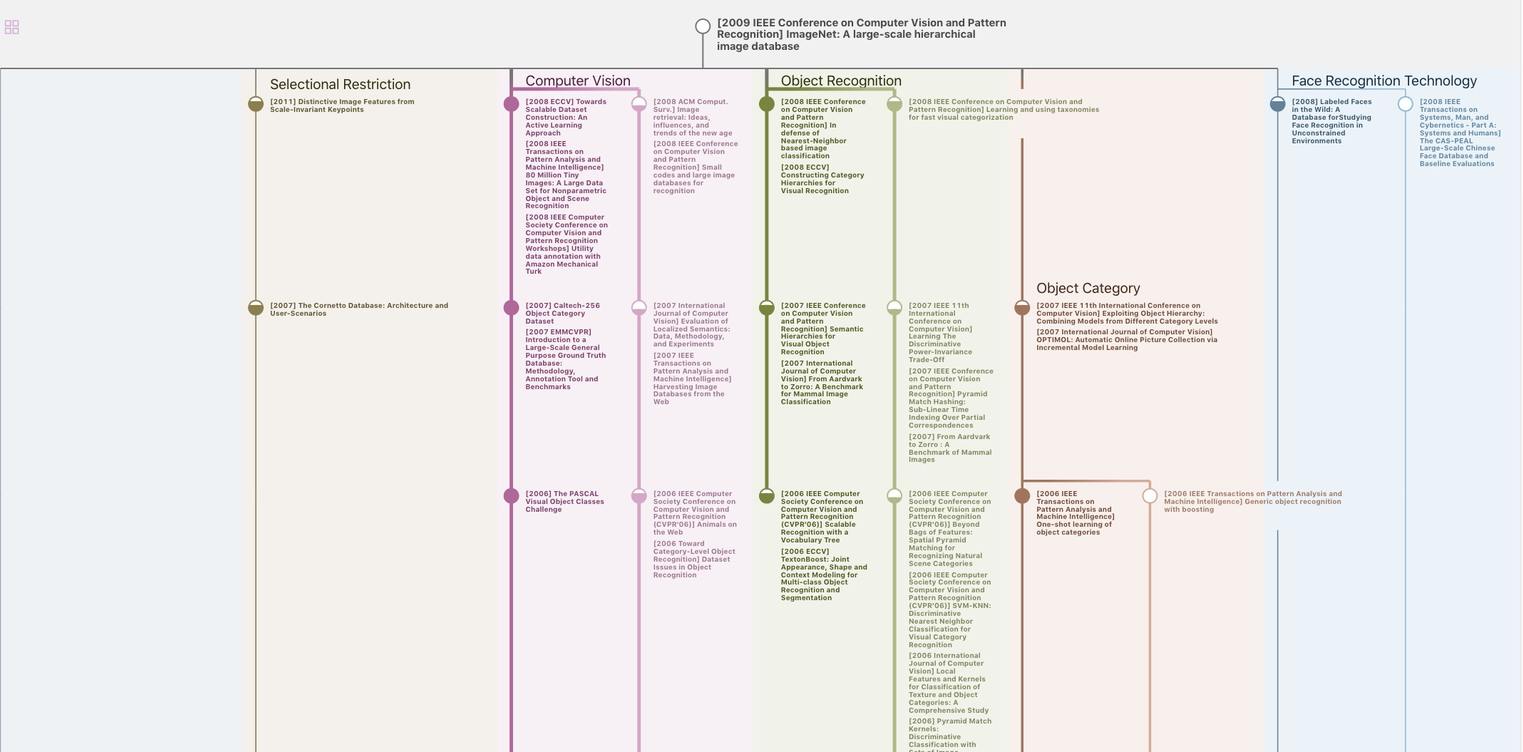
Generate MRT to find the research sequence of this paper
Chat Paper
Summary is being generated by the instructions you defined