Spatiotemporal prediction of landslide displacement using deep learning approaches based on monitored time-series displacement data: a case in the Huanglianshu landslide
GEORISK-ASSESSMENT AND MANAGEMENT OF RISK FOR ENGINEERED SYSTEMS AND GEOHAZARDS(2023)
摘要
The use of deep learning approaches to predict landslide displacement based on monitored time-series data is an effective method for the early-warning of landslides. Currently, most prediction models focus on the temporal correlation of displacements from a single monitoring point, ignoring the spatial influence of other monitoring points. To fully consider the spatiotemporal features of the displacement data, this paper develops three deep learning models based on graph convolution networks to spatiotemporally predict the landslide displacements of the Huanglianshu landslide. Specifically, we first establish a fully connected graph to represent the spatial relationships of all the deployed monitoring points. Second, we develop a temporal graph convolutional network-long short term memory (TGCN-LSTM) model and an Attention-TGCN model based on the temporal graph convolutional network-gate recurrent unit (TGCN-GRU) deep learning model and employ the three models to spatiotemporally predict displacements of the Huanglianshu landslide. The proposed spatiotemporal prediction models accurately predict the displacements at seven monitoring points, with a maximum R-2 of 0.85 at the individual monitoring points. The comparative results show that the proposed Attention-TGCN model achieves the highest spatiotemporal prediction accuracy, and the accuracy of the Attention-TGCN model can further improve after considering the movement of the monitoring points.
更多查看译文
关键词
Landslide,time-Series displacement data,spatiotemporal prediction,deep learning,attention mechanism
AI 理解论文
溯源树
样例
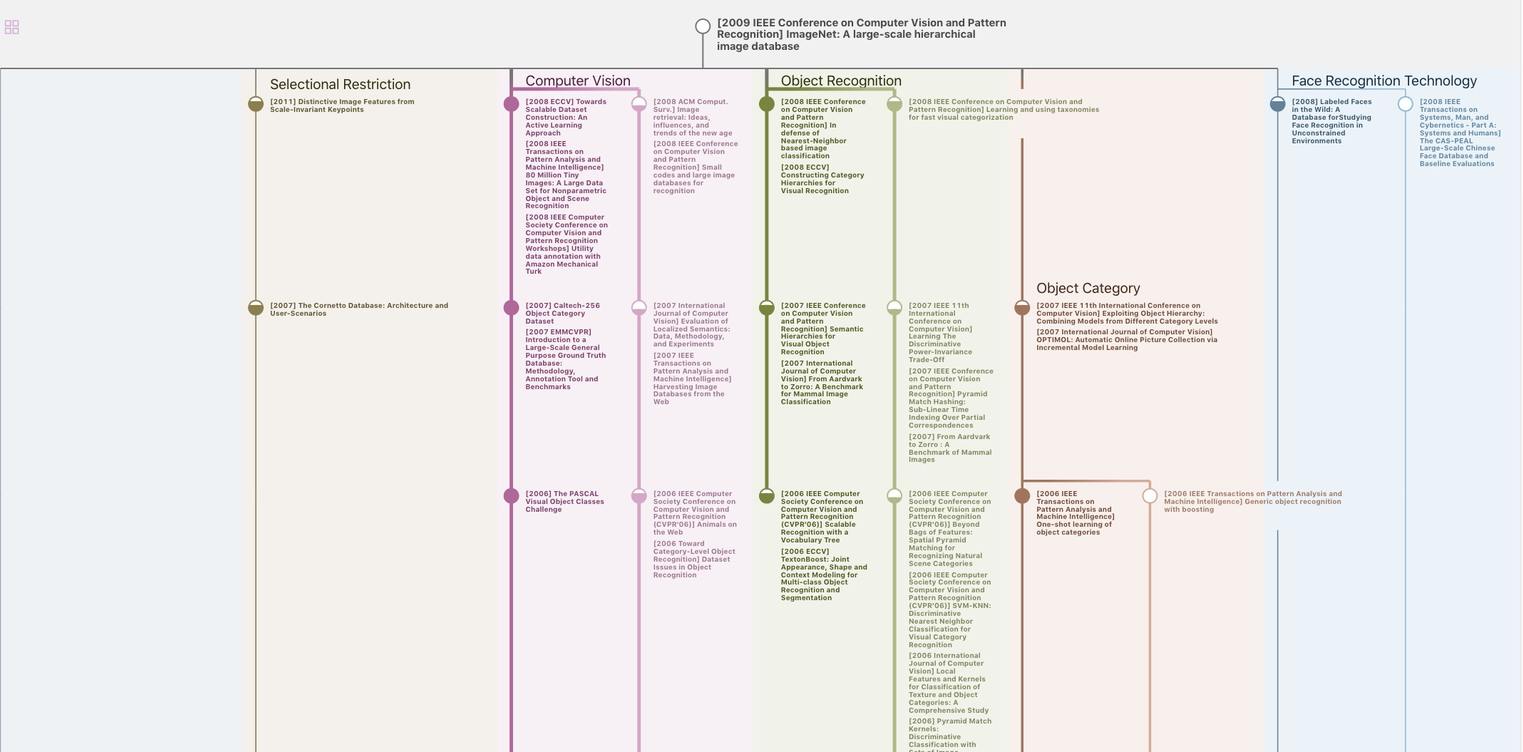
生成溯源树,研究论文发展脉络
Chat Paper
正在生成论文摘要