Remaining useful life prediction combining temporal convolutional network with nonlinear target function
MEASUREMENT SCIENCE AND TECHNOLOGY(2023)
Abstract
Remaining useful life prediction based on degraded data is the premise of intelligent maintenance of equipment. Since the degradation process is usually complex and changeable, the general deep learning prediction method brings a large prediction deviation since it uses linear target function. In this paper, temporal convolutional network combined with a nonlinear target function (NT-TCN) is proposed to improve the prediction accuracy. The nonlinear target function is constructed using a piecewise function to label degraded data. By means of dilated causal convolution and Mish activation function, the NT-TCN learns the time series characteristics of degeneration. Thus, high precision of life prediction is achieved. By analyzing NASA's C-MAPSS data set, the prediction results show that the NT-TCN model has lower root mean squared error and evaluation score than the neural network model with the linear target function, which is also superior to some existing prediction methods.
MoreTranslated text
Key words
remaining useful life prediction,temporal convolutional network,nonlinear target function,dilated causal convolution,Mish activation function
AI Read Science
Must-Reading Tree
Example
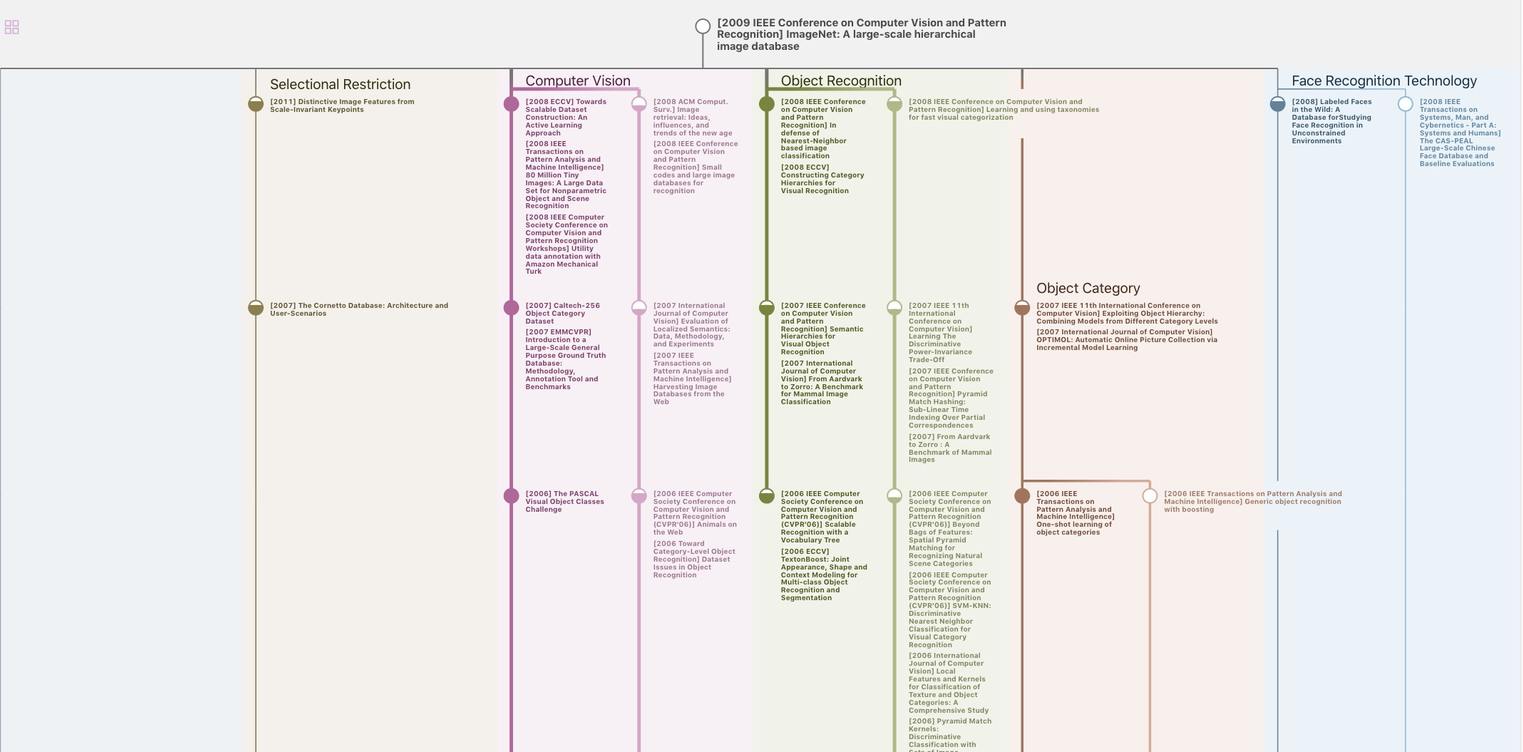
Generate MRT to find the research sequence of this paper
Chat Paper
Summary is being generated by the instructions you defined