An Unsupervised Machine-Learning Approach to Understanding Seismicity at an Alpine Glacier
JOURNAL OF GEOPHYSICAL RESEARCH-EARTH SURFACE(2022)
Abstract
It is critical to understand the dynamic conditions of Earth's cryosphere, yet the subglacial and englacial environments that control many aspects of ice behavior are inherently difficult to observe. The study of seismicity in glaciers and ice sheets has provided valuable insights about the cryosphere for decades, more recently aided by tools from machine learning. Here, we present an unsupervised machine-learning approach to discovering and interpreting cryoseismic patterns using 5 weeks of seismic data recorded at Gornergletscher, Switzerland. Our algorithm utilizes non-negative matrix factorization and hidden Markov modeling to reduce spectrograms into characteristic, low-dimensional "fingerprints," which we reduce further using principal component analysis, then cluster using k-means clustering. We investigate the timing, locations, and statistical properties of the clusters in relation to temperature, GPS and lake-level measurements, and find that signals associated with lake flooding tend to occupy one cluster, whereas signals associated with afternoon and evening melt-water flow reside in others. We suggest that the one cluster contains signals that include the true initiation of the flood's englacial and subglacial drainage components. This work demonstrates an unsupervised machine-learning approach to exploring both continuous and event-based glacial seismic data. Plain Language Summary Earth's climate change poses extreme risks for the stability of glaciers and ice sheets. One such glacier, Gornergletscher in the Swiss Alps, has been monitored for decades, along with a lake on the glacier which from the 1950s until recently filled and drainedevery summer, releasing huge amounts of water under, through, and on top of the glacier. To study this glacier, which is hundreds of meters thick, and its lake, scientists employ "cryoseismology," the study of seismic excitations in the ice. In our study, we use unsupervised machine-learning, a type of artificial intelligence that identifies patterns in large data sets, to organize 5 weeks of cryoseismic records into clusters composed of similar types of signals. Although the machine-learning algorithm has no knowledge of glacial hydrology nor its evolution over time, the output places the signals associated with the beginning of lake flooding in one cluster, and signals associated with afternoon and evening melt-water flow in others. We also find that "icequakes" are clustered based on extremely subtle, similar, vibrations. From these findings, we provide an improved chronology for the glacial lake flood, and a better understanding of the cryoseismic signals caused by water flowing underneath and through glaciers.
MoreTranslated text
Key words
cryoseismology,cryosphere,machine learning,GLOF,icequakes
AI Read Science
Must-Reading Tree
Example
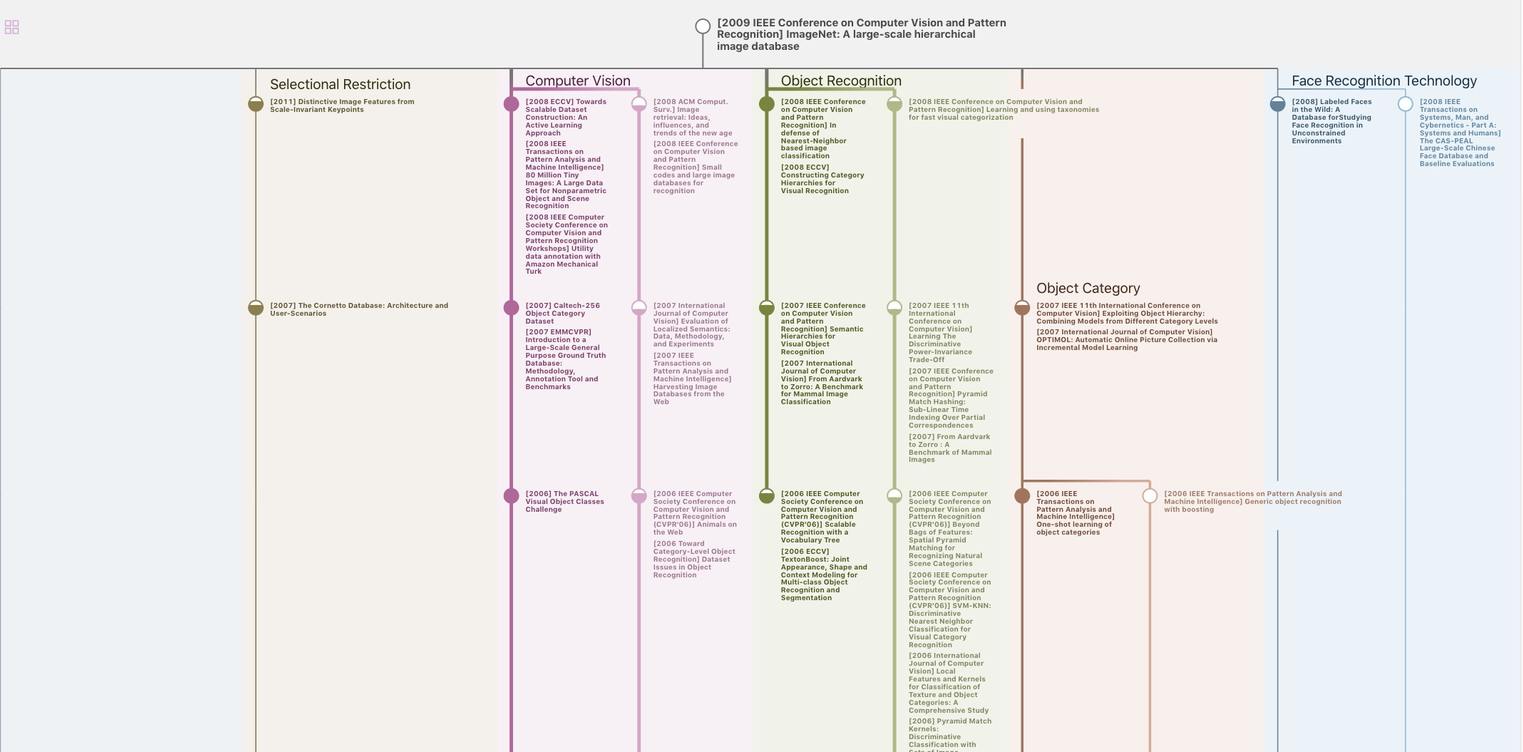
Generate MRT to find the research sequence of this paper
Chat Paper
Summary is being generated by the instructions you defined