A fast magnitude estimation method based on deep convolutional neural networks
CHINESE JOURNAL OF GEOPHYSICS-CHINESE EDITION(2023)
Abstract
The magnitude estimation method in earthquake early warning is traditionally realized by the empirical relationship between the characteristic parameters based on the initial few seconds of arriving seismic waves and the magnitude. These characteristic parameters depend on human experience and subjective judgment, and do not make full use of the information related to magnitude in the initial seismic waves, which restricts the effect of magnitude estimation. To address this issue, this paper uses a deep convolutional neural network (CNN) to directly extract features from initial seismic waves to achieve end-to-end magnitude estimation. CNN uses the vertical component of initial seismic records from a single station as the main input, epicentral distance, focal depth, and 1753, as auxiliary inputs, and magnitude as its output. The proposed CNN was trained (98,257 records), verified (31, 429 records) and tested (40, 638 records) by using a large number of surface accelerograms from Japan and Chile, and the generalization performance of the proposed CNN was tested by accelerograms (583 records) from the United States and New Zealand, and the results were compared with the most widely used peak displacement Pd method. The comparison shows that when the duration of initial P wave is 3 s, the accuracy of magnitude estimation by the CNN is 1.5 times that of the Pd method for magnitude range of 4 similar to 6.4, and 1.2 times that of Pd method in the magnitude range of 6.5 similar to 9. When the duration of initial seismic wave increases from 3 s to 10 s, the CNN continuously improves the accuracy of magnitude estimation with the increasing duration of initial seismic waves, and it is always better than the Pd method. It should be noted that for the magnitude range of 4 similar to 6.4, the accuracy of magnitude estimation by the CNN using the initial 3 s seismic wave is 1.2 times that of the Pd method using the initial 10 s seismic wave. With the increase of seismic wave duration, CNN performs better than Pd in addressing the magnitude saturation issue. Compared with manually defined characteristic parameters, CNN automatically learns features that are closely related to magnitude from the initial seismic waves, and these features greatly improve the accuracy and timeliness for the magnitude estimation. The proposed approach can provide faster and more accurate magnitude estimation for earthquake early warning systems.
MoreTranslated text
Key words
Earthquake early warning,Magnitude estimation,Convolutional neural networks,Deep learning
AI Read Science
Must-Reading Tree
Example
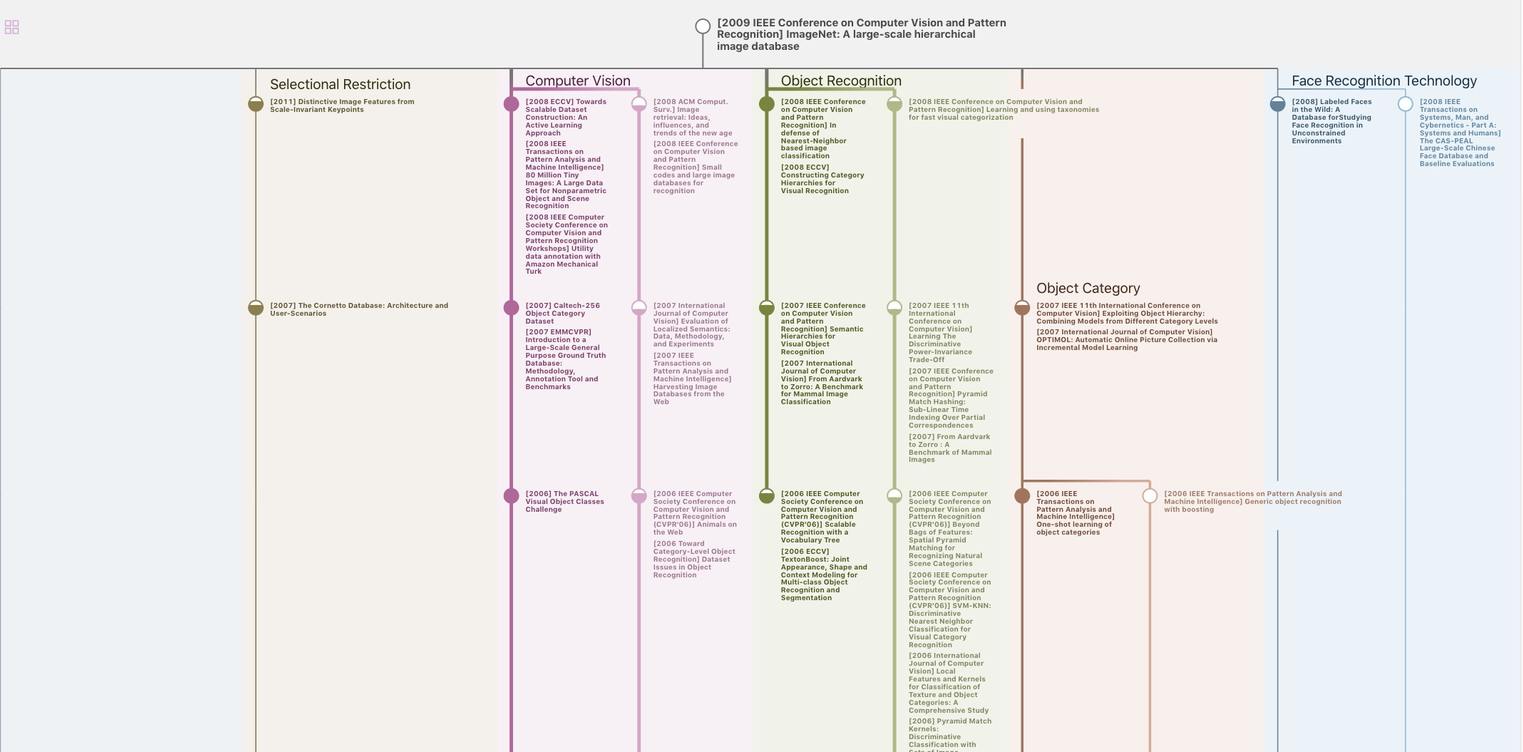
Generate MRT to find the research sequence of this paper
Chat Paper
Summary is being generated by the instructions you defined