Multi-view multi-objective clustering-based framework for scientific document summarization using citation context
APPLIED INTELLIGENCE(2023)
摘要
Due to the expanding rate of scientific publications, it has become a necessity to summarize scientific documents to allow researchers to keep track of recent developments. In this paper, we formulate the scientific document summarization problem in a multi-view clustering (MVC) framework. Two views of the scientific documents, semantic and syntactic, are considered jointly in MVC framework. To obtain an improved partitioning corresponding to different views, a differential evolution algorithm is utilized as the underlying optimization strategy. After obtaining the final optimal partitioning, various sentence-level features like length, position of the sentences, among others, are investigated to extract high scoring sentences and these extracted sentences are used to form the summary. We have also investigated the use of (a) two embedding spaces to represent the sentences of the documents in semantic space; and (b) use of citation contexts to incorporate their importance in summarizing a given scientific document. Our proposed multi-view based clustering approach is purely unsupervised in nature and, does not utilize any labelled data for the generation of the summary. To show the potentiality of multi-view clustering, a single-view based clustering framework is also developed for the purpose of comparison. The performance of the multi-view based system in comparison to a single-view is first tested on some random articles selected from the recently released SciSummNet 2019 dataset using ROUGE measure. Then, two more datasets namely, CL-SciSumm 2016 and CL-SciSumm 2017 are considered for evaluation. Our investigations prove that the proposed approach yields better results than the existing supervised and unsupervised methods including transformer-based deep learning model, with statistically significant improvements.
更多查看译文
关键词
Multi-view learning, Clustering, Citation context, Scientific document summarization (SDS), Evolutionary algorithm (EA), Multi-objective optimization (MOO)
AI 理解论文
溯源树
样例
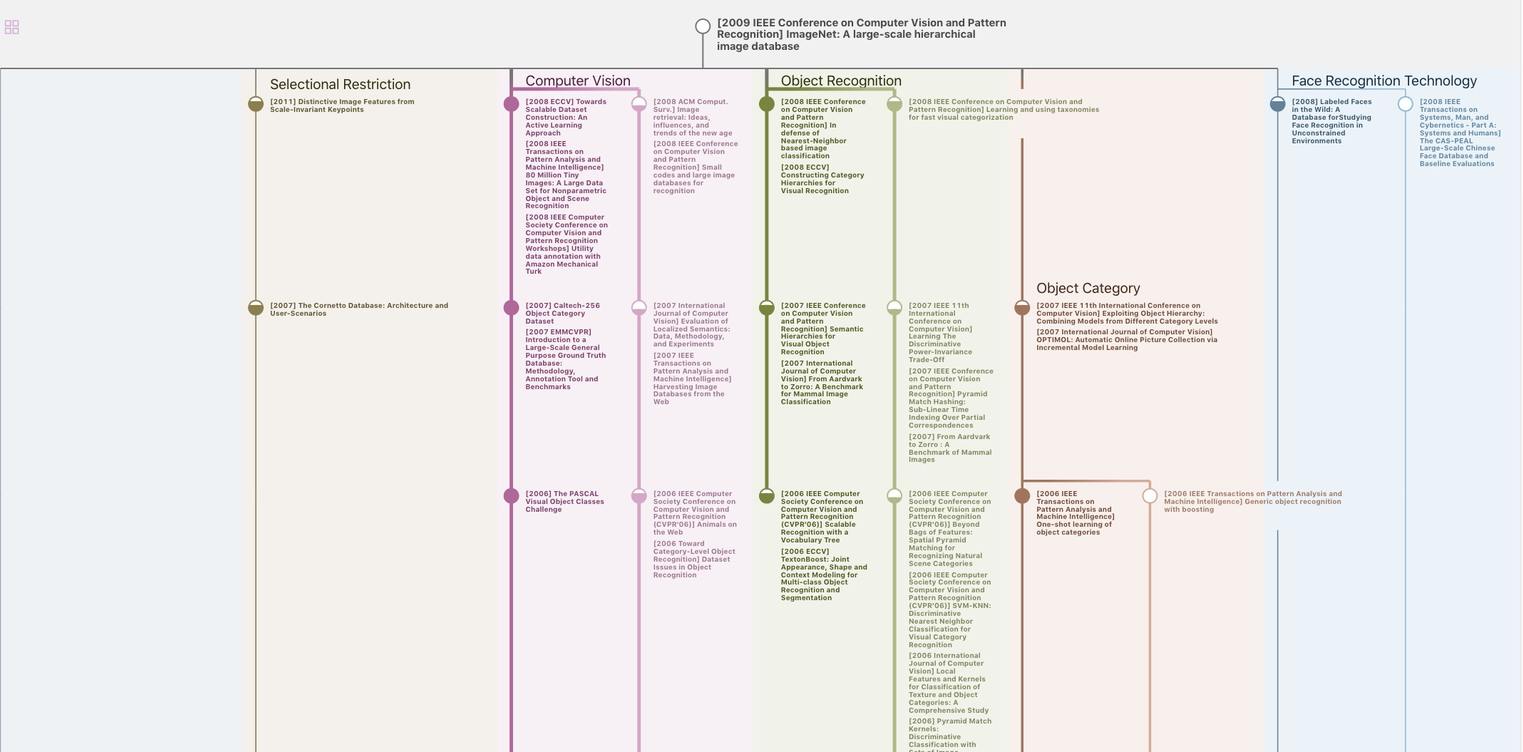
生成溯源树,研究论文发展脉络
Chat Paper
正在生成论文摘要