Physics-informed machine learning combining experiment and simulation for the design of neodymium-iron-boron permanent magnets with reduced critical-elements content
FRONTIERS IN MATERIALS(2023)
摘要
Rare-earth elements like neodymium, terbium and dysprosium are crucial to the performance of permanent magnets used in various green-energy technologies like hybrid or electric cars. To address the supply risk of those elements, we applied machine-learning techniques to design magnetic materials with reduced neodymium content and without terbium and dysprosium. However, the performance of the magnet intended to be used in electric motors should be preserved. We developed machine-learning methods that assist materials design by integrating physical models to bridge the gap between length scales, from atomistic to the micrometer-sized granular microstructure of neodymium-iron-boron permanent magnets. Through data assimilation, we combined data from experiments and simulations to build machine-learning models which we used to optimize the chemical composition and the microstructure of the magnet. We applied techniques that help to understand and interpret the results of machine learning predictions. The variables importance shows how the main design variables influence the magnetic properties. High-throughput measurements on compositionally graded sputtered films are a systematic way to generate data for machine data analysis. Using the machine learning models we show how high-performance, Nd-lean magnets can be realized.
更多查看译文
关键词
machine learning-ML,materials design,NdFeB permanent magnet,combinatorial sputtering,rare-earth element (REE),optimization,ab-initio simulation,micromagnetic simulation
AI 理解论文
溯源树
样例
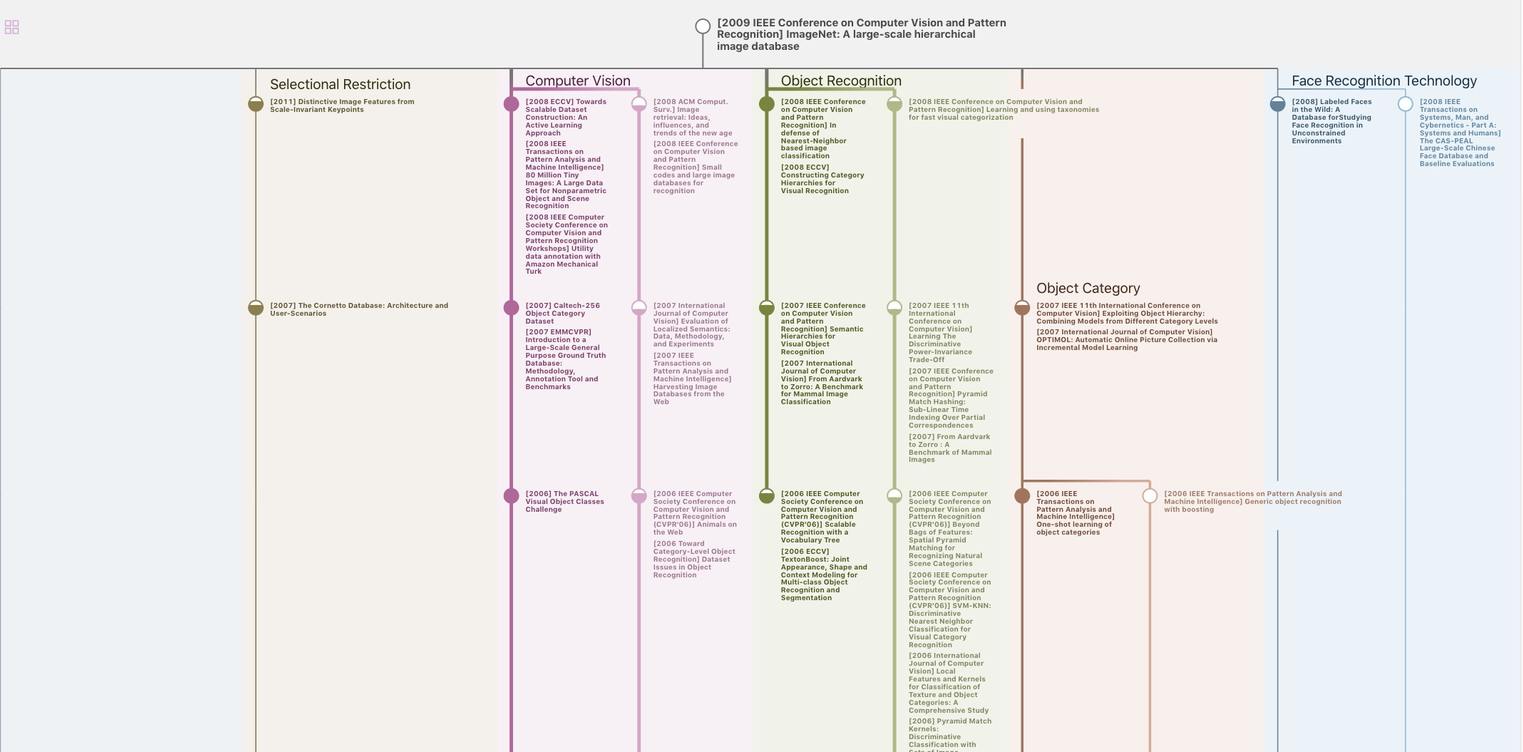
生成溯源树,研究论文发展脉络
Chat Paper
正在生成论文摘要