Remaining useful life prediction of bearings based on self-attention mechanism, multi-scale dilated causal convolution, and temporal convolution network
MEASUREMENT SCIENCE AND TECHNOLOGY(2023)
摘要
Effective remaining useful life (RUL) prediction of bearings is essential for the predictive maintenance of rotating machinery. However, the effectiveness of many existing RUL prediction methods depends on expert experience and signal processing algorithms, which limiting the application of these methods in real-life scenarios. This study proposes a novel end-to-end deep learning framework consisting of a multi-scale attention-based dilated causal convolutional (MADCC) module and a multi-layer temporal convolutional network (MTCN) to predict the RUL of bearings using raw vibration data. First, the MADCC module extracts multi-scale temporal features of the bearing degradation process (BDP) and provides fused feature vectors (FFVs) containing comprehensive BDP information for the MTCN module. Subsequently, the MTCN module mines deep temporal dependencies hidden in the FFV to predict the RUL of bearings. Ablation experiments are conducted to analyze the contribution of the framework's components. Three evaluation metrics (root mean square error (RMSE), mean absolute error (MAE), and coefficient of determination (R (2))) are used to verify the effectiveness of the proposed framework and other state-of-the-art methods on two public bearing datasets. The experimental results show that the proposed framework achieves the lowest RMSE and MAE and the highest R (2), demonstrating excellent performance and potential for RUL prediction of bearings.
更多查看译文
关键词
bearing,rotating machinery,remaining useful life prediction,deep learning,temporal convolution network
AI 理解论文
溯源树
样例
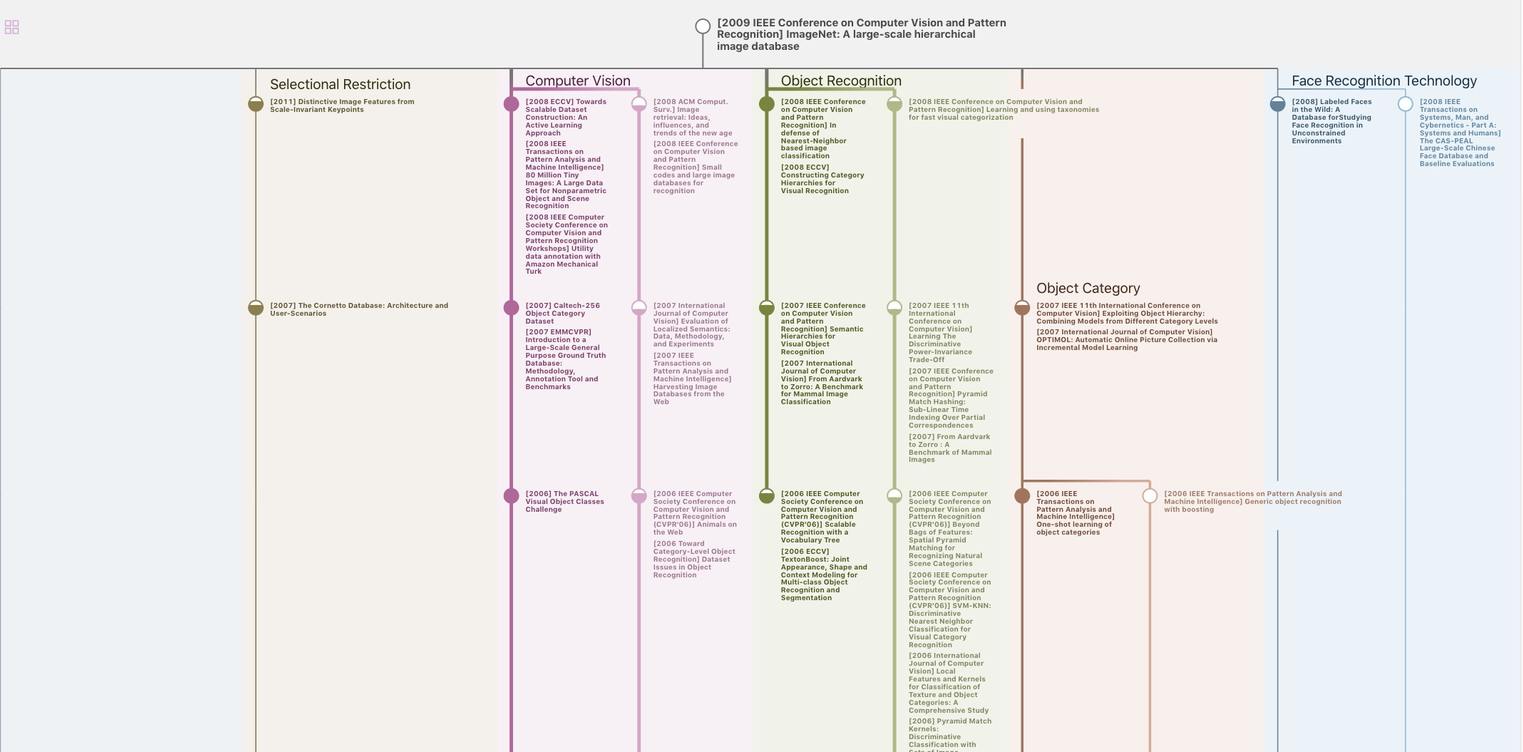
生成溯源树,研究论文发展脉络
Chat Paper
正在生成论文摘要