Propensity Score Matching with Cross-Classified Data Structures: A Comparison of Methods
JOURNAL OF EXPERIMENTAL EDUCATION(2024)
摘要
In the current study, we compare propensity score (PS) matching methods for data with a cross-classified structure, where each individual is clustered within more than one group, but the groups are not hierarchically organized. Through a Monte Carlo simulation study, we compared sequential cluster matching (SCM), preferential within cluster matching (PWCM), greedy matching (GM), and optimal full matching (OFM), using propensity scores from four different models. The results indicated that the four matching methods performed well when PSs were estimated with logistic regression containing both level-1 and level-2 covariates. When the level-2 covariates were omitted in the logistic regression PS model, matching methods resulted in biased treatment effect estimates. However, omission of level-2 covariates did not result in biased estimates when the PS model was a logistic cross-classified random effects model (CCREM). SCM and PWCM outperformed GM and OFM with a logistic CCREM that included level-1 and level-2 covariates.
更多查看译文
关键词
Cross-classified data,cross-classified random effects model,one-to-many nearest neighbor matching,propensity scores,sequential cluster matching
AI 理解论文
溯源树
样例
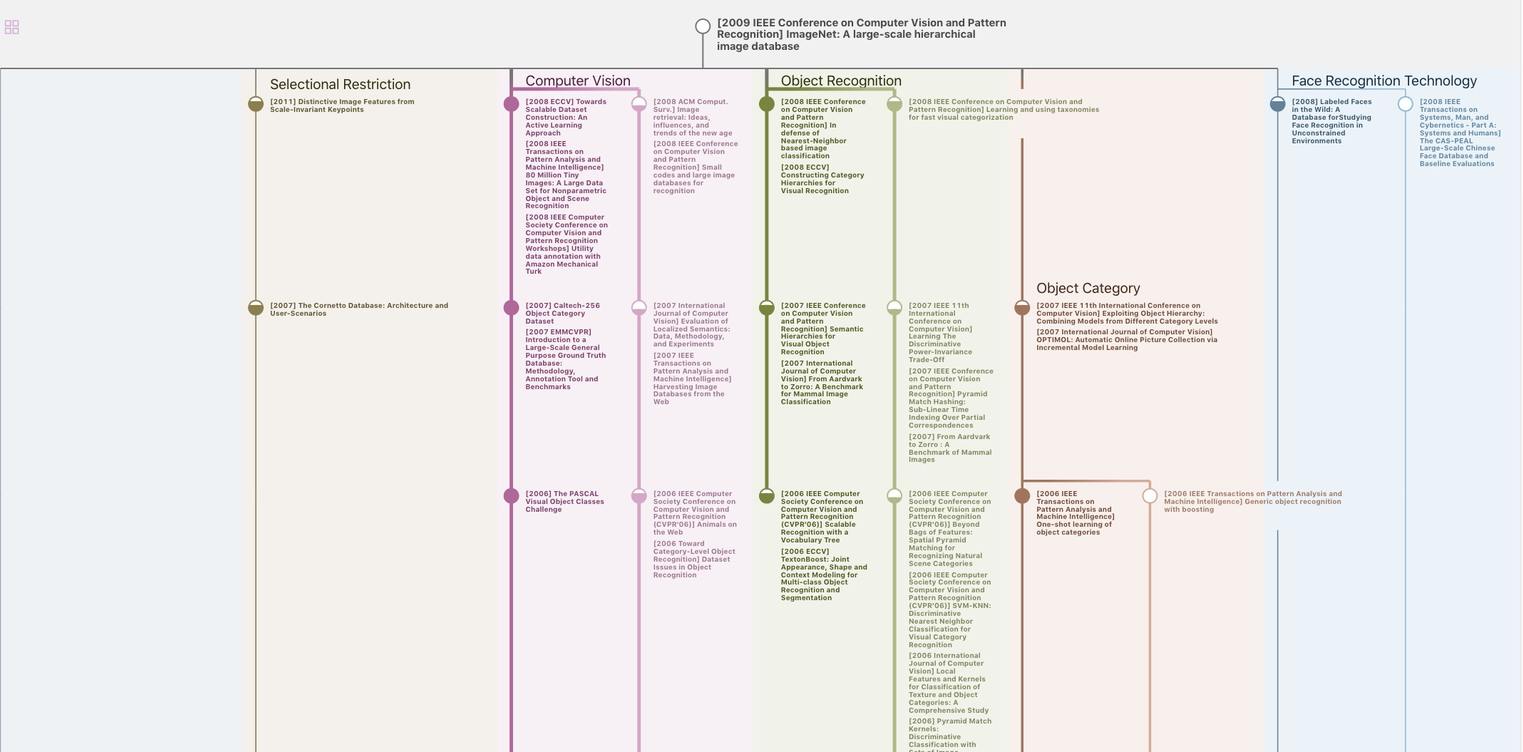
生成溯源树,研究论文发展脉络
Chat Paper
正在生成论文摘要