Time-Varying Sequence Model
MATHEMATICS(2023)
摘要
Traditional machine learning sequence models, such as RNN and LSTM, can solve sequential data problems with the use of internal memory states. However, the neuron units and weights are shared at each time step to reduce computational costs, limiting their ability to learn time-varying relationships between model inputs and outputs. In this context, this paper proposes two methods to characterize the dynamic relationships in real-world sequential data, namely, the internal time-varying sequence model (ITV model) and the external time-varying sequence model (ETV model). Our methods were designed with an automated basis expansion module to adapt internal or external parameters at each time step without requiring high computational complexity. Extensive experiments performed on synthetic and real-world data demonstrated superior prediction and classification results to conventional sequence models. Our proposed ETV model is particularly effective at handling long sequence data.
更多查看译文
关键词
sequence model,basis expansion,dynamic weight update,neural networks
AI 理解论文
溯源树
样例
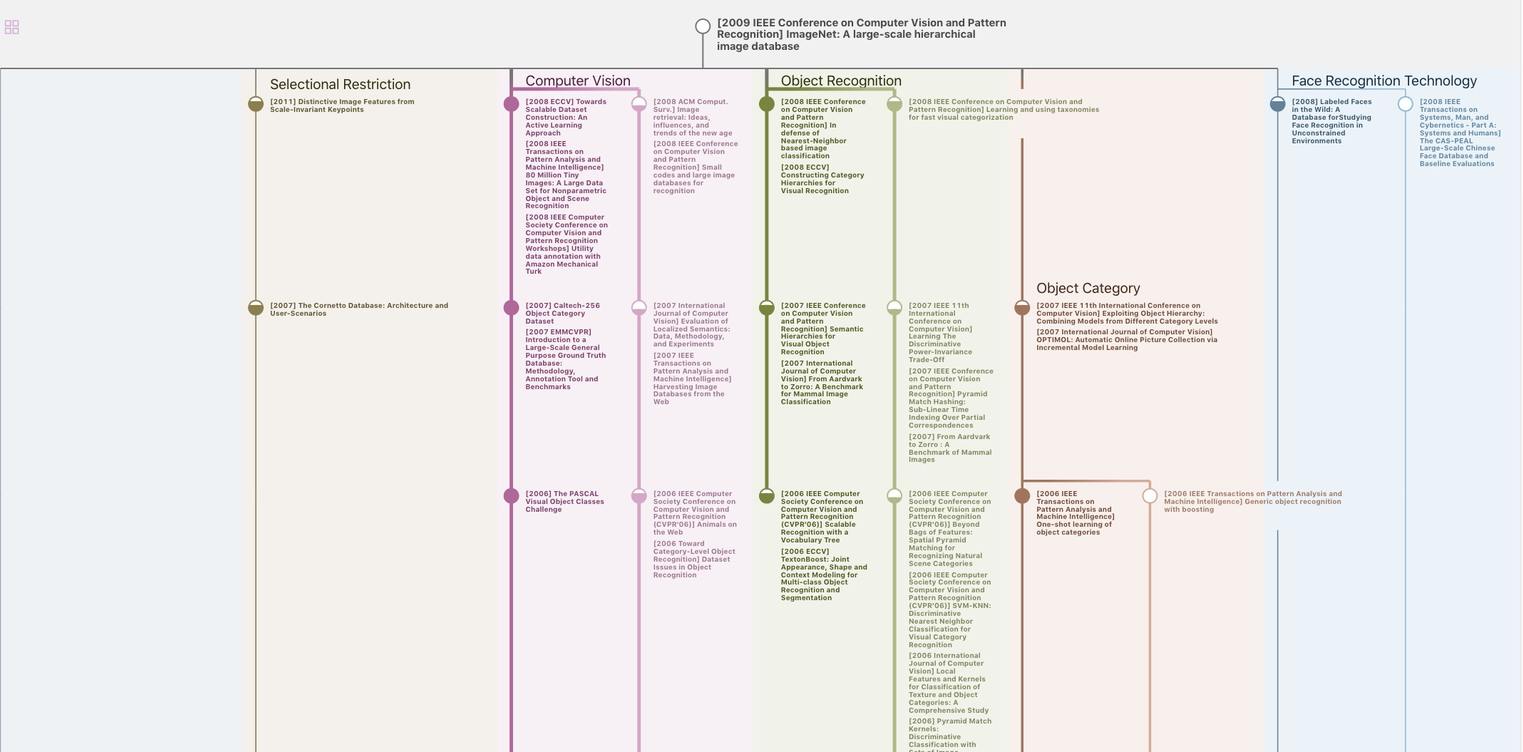
生成溯源树,研究论文发展脉络
Chat Paper
正在生成论文摘要