A novel hardware authentication primitive against modeling attacks
INTERNATIONAL JOURNAL OF CIRCUIT THEORY AND APPLICATIONS(2023)
摘要
Traditional hardware security primitives such as physical unclonable functions (PUFs) are quite vulnerable to machine learning (ML) attacks. The primary reason is that PUFs rely on process mismatches between two identically designed circuit blocks to generate deterministic math functions as the secret information sources. Unfortunately, ML algorithms are pretty efficient in modeling deterministic math functions. In order to resist against ML attacks, in this letter, a novel hardware security primitive named neural network (NN) chain is proposed by utilizing noise data to generate chaotic NNs for achieving authentication. In a NN chain, two independent batches of noise data are utilized as the input and output training data of NNs, respectively, to maximize the uncertainty within the NN chain. In contrast to a regular PUF, the proposed NN chain is capable of achieving over 20 times ML attackresistance and 100% reliability with less than 39% power and area overhead.
更多查看译文
关键词
deterministic math functions,machine learning (ML) attacks,neural network (NN) chain,physical unclonable functions (PUFs)
AI 理解论文
溯源树
样例
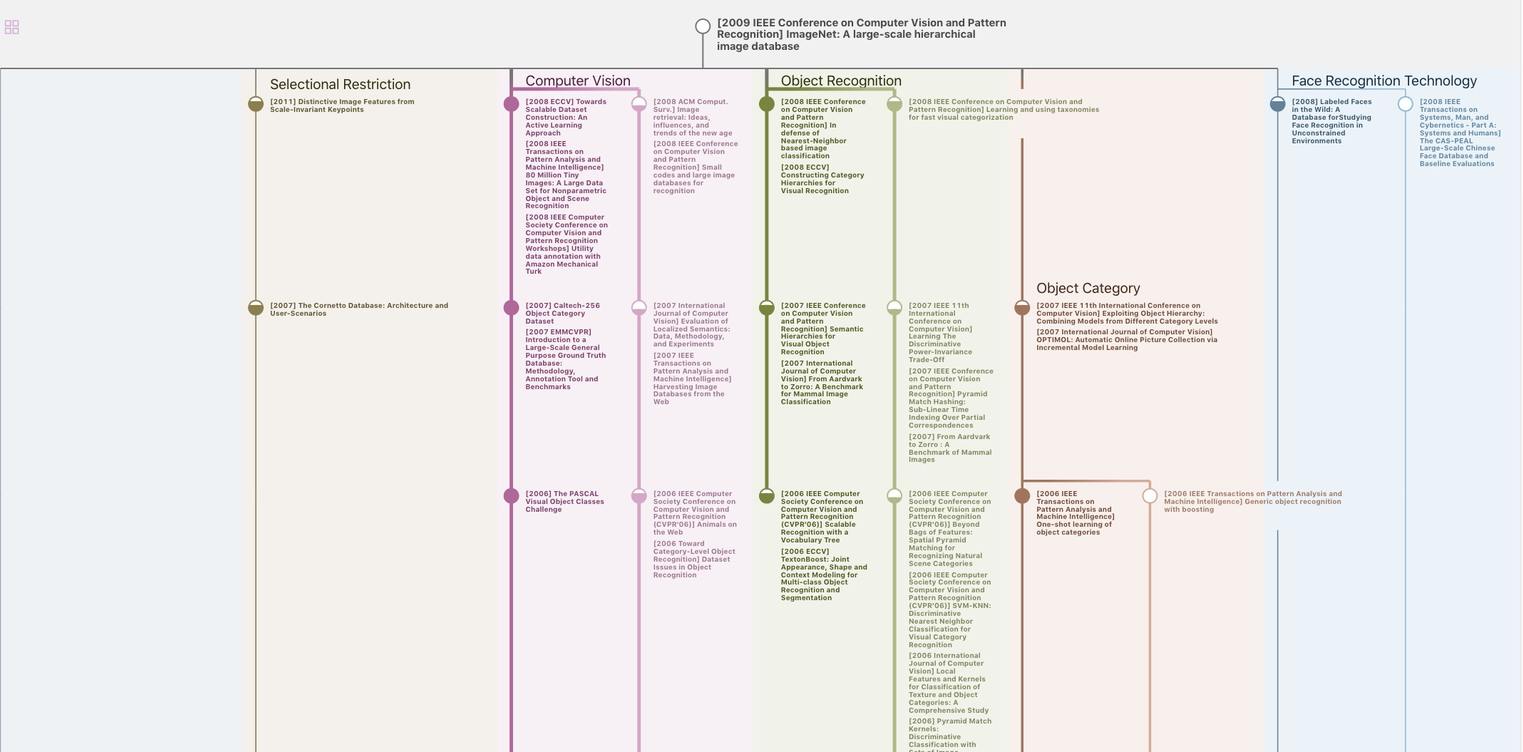
生成溯源树,研究论文发展脉络
Chat Paper
正在生成论文摘要