A Machine Learning Approach to Real-World Time to Treatment Discontinuation Prediction
ADVANCED INTELLIGENT SYSTEMS(2023)
摘要
Real-world time to treatment discontinuation (rwTTD) is an important endpoint measurement of drug efficacy evaluated using real-world observational data. rwTTD, represented as a set of metrics calculated from a population-wise curve, cannot be predicted by existing machine learning approaches. Herein, a methodology that enables predicting rwTTD is developed. First, the robust performance of the model in predicting rwTTD across populations of similar or distinct properties with simulated data using a variety of commonly used base learners in machine learning is demonstrated. Then, the robust performance of the approach both within-cohort and cross-disease using real-world observational data of pembrolizumab for advanced lung cancer and head neck cancer is demonstrated. This study establishes a generic pipeline for real-world time on treatment prediction, which can be extended to any base machine learners and drugs. Currently, there is no existing machine learning approach established for predicting population-wise rwTTD, despite that it is an essential metric to report real-world drug efficacy. Therefore, we believe our study opens a new investigation area of rwTTD prediction, and provides an innovative approach to probe this problem and other problems involving population-wise predictions. An interactive preprint version of the article can be found at: .
更多查看译文
关键词
drug effacacy,machine learning,rwToT,time-series prediction
AI 理解论文
溯源树
样例
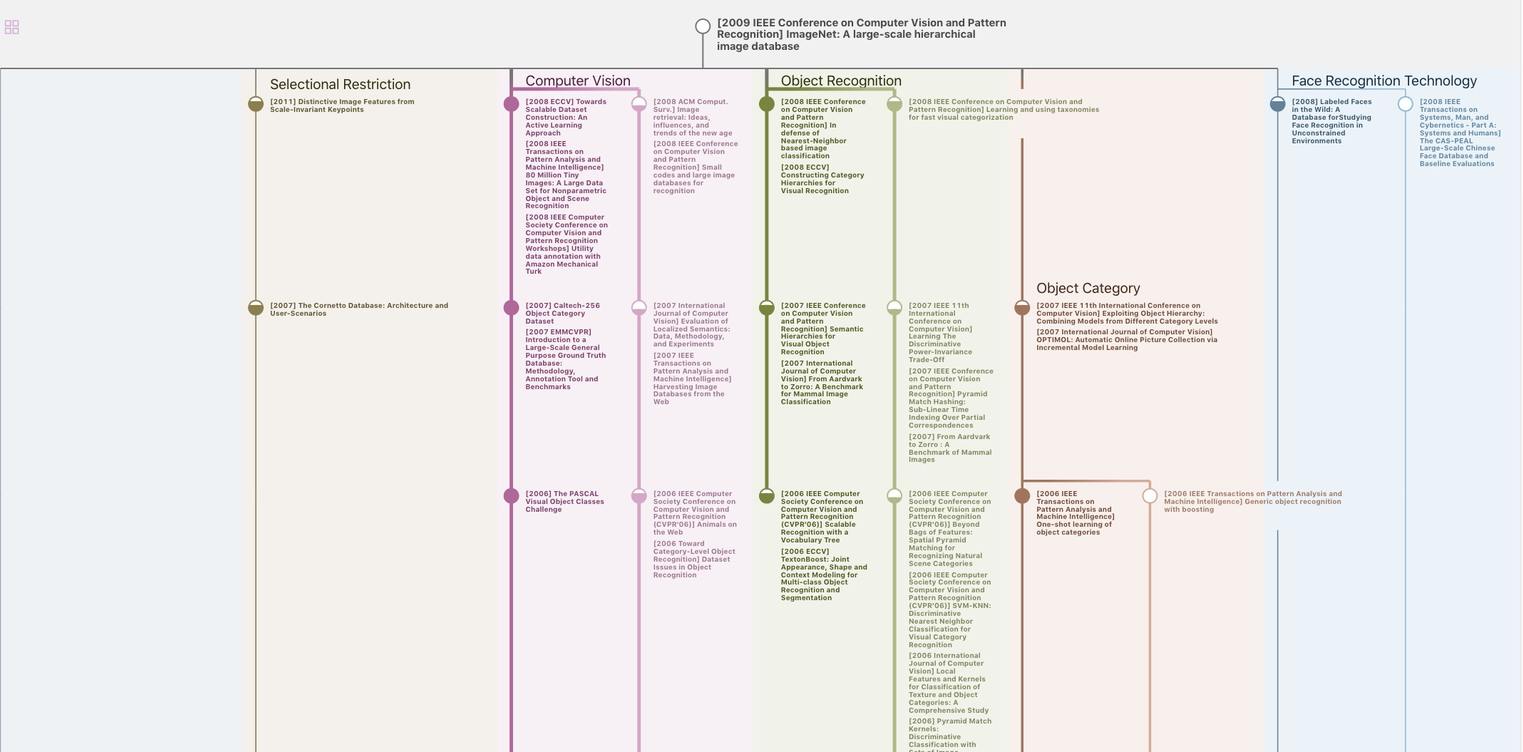
生成溯源树,研究论文发展脉络
Chat Paper
正在生成论文摘要