Machine Learning-Based Prediction of Conversion Coefficients for I-123 Metaiodobenzylguanidine Heart-to-mediastinum Ratio
Journal of Nuclear Cardiology(2023)
关键词
123I-MIBG,heart-to-mediastinum ratio,Monte Carlo simulation,collimator
AI 理解论文
溯源树
样例
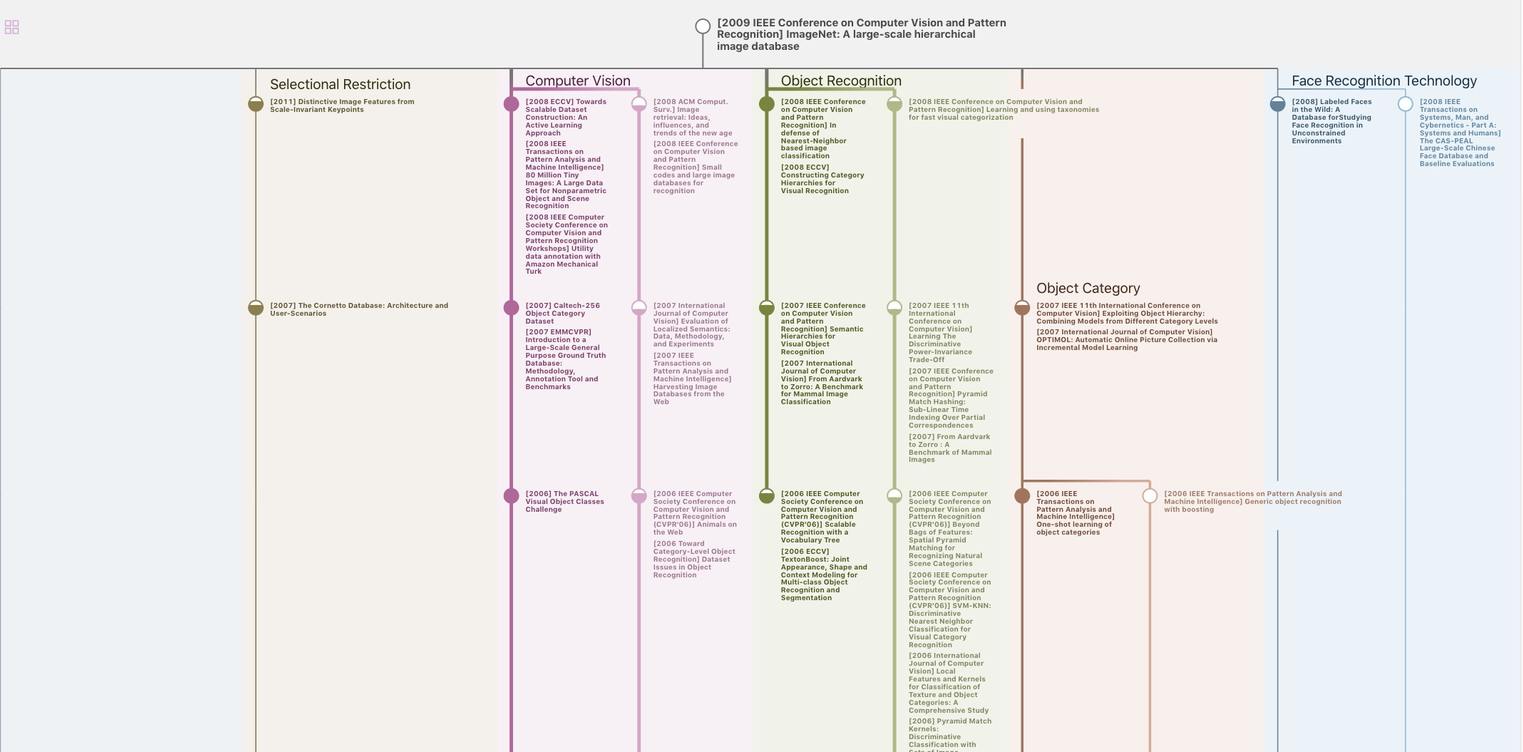
生成溯源树,研究论文发展脉络
Chat Paper
正在生成论文摘要