An adaptive medical image registration using hybridization of teaching learning-based optimization with affine and speeded up robust features with projective transformation
CLUSTER COMPUTING-THE JOURNAL OF NETWORKS SOFTWARE TOOLS AND APPLICATIONS(2024)
摘要
In the last several decades, application of affine transformation and optimization approaches have received enough attention in the domain of monomodal/multimodal image registration. A novel and robust image registration method is proposed which leverages teaching learning-based optimization (TLBO) to obtain the optimal value of rigid transformation parameters. Further, speeded up robust features (SURF) framework is employed to extract features along with Random sample consensus (RANSAC) algorithm. Afterwards, projective transformation is used to obtain more accurate registered image. To remove noise, gaussian filter is used during pre-processing and then normalization is carried out. TLBO is used to identify the optimal geometric transformation parameters by considering the mutual information (MI) as an objective function. This method detects keypoints (features) using SURF then K-nearest neighbour (KNN) is employed to match the detected features. Furthermore, RANSAC eliminates false matches. The registered image with optimal value of rigid transformation parameters is obtained and then enhanced by using SURF-RANSAC followed by projective transformation. Experiments are conducted on Whole Brain ATLAS and KAGGLE datasets. Robustness of proposed approach is verified by the improvement in evaluation metric, such as structure similarity index measure (SSIM) by 8%, MI by 12.71% on monomodal and root mean square error (RMSE) by 41.44% on multimodal images as compared to recent state-of-the-art methods.
更多查看译文
关键词
Affine transformation,Mutual information,SURF,RANSAC,Projective transformation,TLBO
AI 理解论文
溯源树
样例
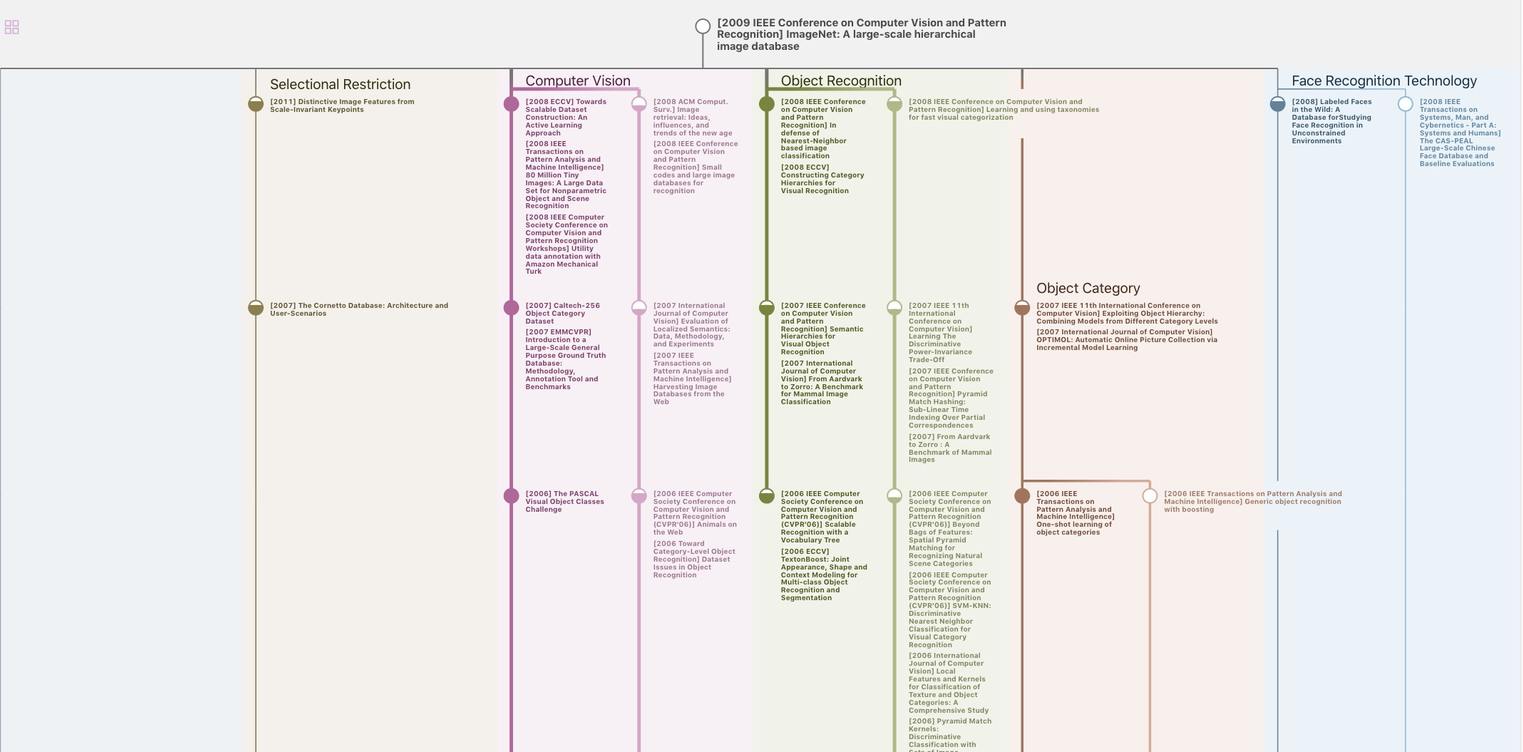
生成溯源树,研究论文发展脉络
Chat Paper
正在生成论文摘要