Achieving Better Category Separability for Hyperspectral Image Classification: A Spatial-Spectral Approach.
IEEE transactions on neural networks and learning systems(2023)
摘要
The task of hyperspectral image (HSI) classification has attracted extensive attention. The rich spectral information in HSIs not only provides more detailed information but also brings a lot of redundant information. Redundant information makes spectral curves of different categories have similar trends, which leads to poor category separability. In this article, we achieve better category separability from the perspective of increasing the difference between categories and reducing the variation within category, thus improving the classification accuracy. Specifically, we propose the template spectrum-based processing module from spectral perspective, which can effectively expose the unique characteristics of different categories and reduce the difficulty of model mining key features. Second, we design an adaptive dual attention network from spatial perspective, where the target pixel can adaptively aggregate high-level features by evaluating the confidence of effective information in different receptive fields. Compared with the single adjacency scheme, the adaptive dual attention mechanism makes the ability of target pixel to combine spatial information to reduce variation more stable. Finally, we designed a dispersion loss from the classifier's perspective. By supervising the learnable parameters of the final classification layer, the loss makes the category standard eigenvectors learned by the model more dispersed, which improves the category separability and reduces the rate of misclassification. Experiments on three common datasets show that our proposed method is superior to the comparison method.
更多查看译文
关键词
Feature extraction,Iron,Market research,Hyperspectral imaging,Standards,Dispersion,Convolutional neural networks,Category separability,dispersion loss,dual attentive mechanism,hyperspectral image classification (HSIC),template spectrum
AI 理解论文
溯源树
样例
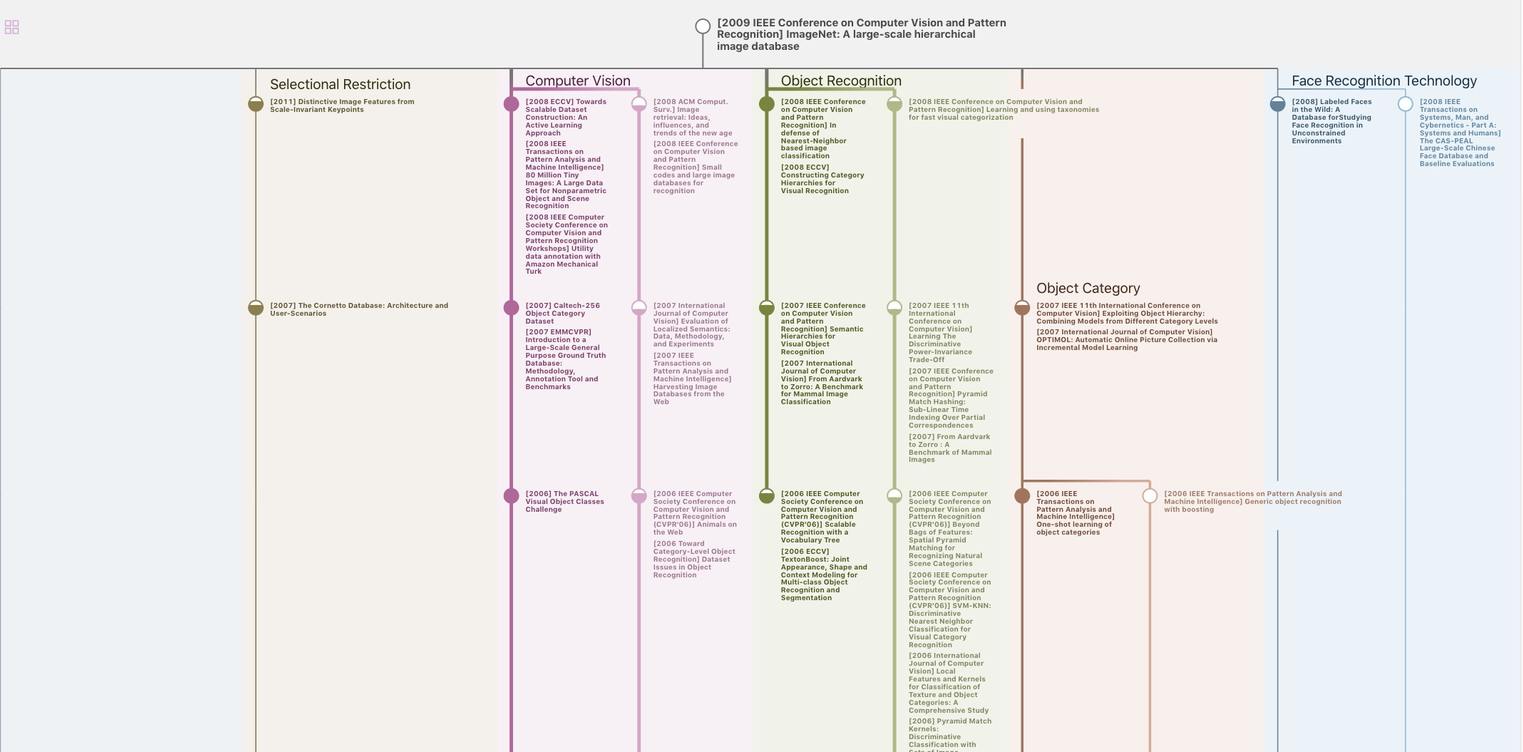
生成溯源树,研究论文发展脉络
Chat Paper
正在生成论文摘要