Free form deformation and symmetry constraint-based multi-modal brain image registration using generative adversarial nets
CAAI TRANSACTIONS ON INTELLIGENCE TECHNOLOGY(2023)
摘要
Multi-modal brain image registration has been widely applied to functional localisation, neurosurgery and computational anatomy. The existing registration methods based on the dense deformation fields involve too many parameters, which is not conducive to the exploration of correct spatial correspondence between the float and reference images. Meanwhile, the unidirectional registration may involve the deformation folding, which will result in the change of topology during registration. To address these issues, this work has presented an unsupervised image registration method using the free form deformation (FFD) and the symmetry constraint-based generative adversarial networks (FSGAN). The FSGAN utilises the principle component analysis network-based structural representations of the reference and float images as the inputs and uses the generator to learn the FFD model parameters, thereby producing two deformation fields. Meanwhile, the FSGAN uses two discriminators to decide whether the bilateral registration have been realised simultaneously. Besides, the symmetry constraint is utilised to construct the loss function, thereby avoiding the deformation folding. Experiments on BrainWeb, high grade gliomas, IXI and LPBA40 show that compared with state-of-the-art methods, the FSGAN provides superior performance in terms of visual comparisons and such quantitative indexes as dice value, target registration error and computational efficiency.
更多查看译文
关键词
Free-form deformation,Generative adversarial nets,Multi-modal brain image registration,Structural representation,Symmetry constraint
AI 理解论文
溯源树
样例
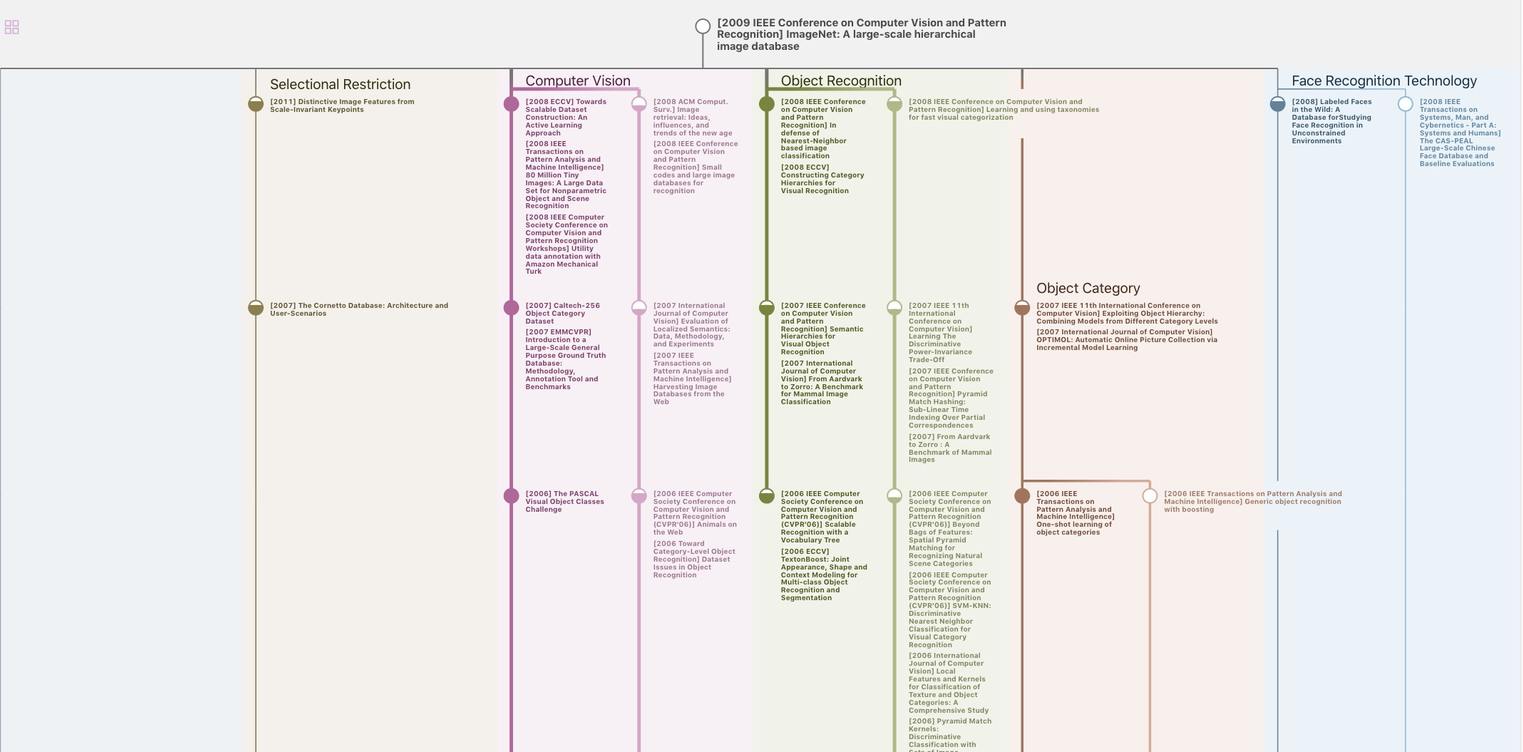
生成溯源树,研究论文发展脉络
Chat Paper
正在生成论文摘要