LSTM-ACB-Based RA for IoT Mixed Traffic
arxiv(2023)
摘要
A novel random access (RA) scheme for mixed URLLC-mMTC traffic scenario is proposed using realistic statistical models, with the use mode presenting long-term traffic regularity. The traffic is predicted by a long short-term memory neural network, which enables a traffic-aware resource slicing aided by contention access class barring-based procedure. The method combines a grant-free (GF) RA scheme with an intermediate step to congestion alleviation. The protocol trade-off is a small overhead while enabling a higher number of decoded received packets thanks to the intermediate step. Numerical results evaluate the system performance for each procedure and combined solution. A comparison with GF benchmark reveals substantial improvement in system performance.
更多查看译文
关键词
Random access protocols,IoT networks,network slicing,long-short term memory (LSTM)
AI 理解论文
溯源树
样例
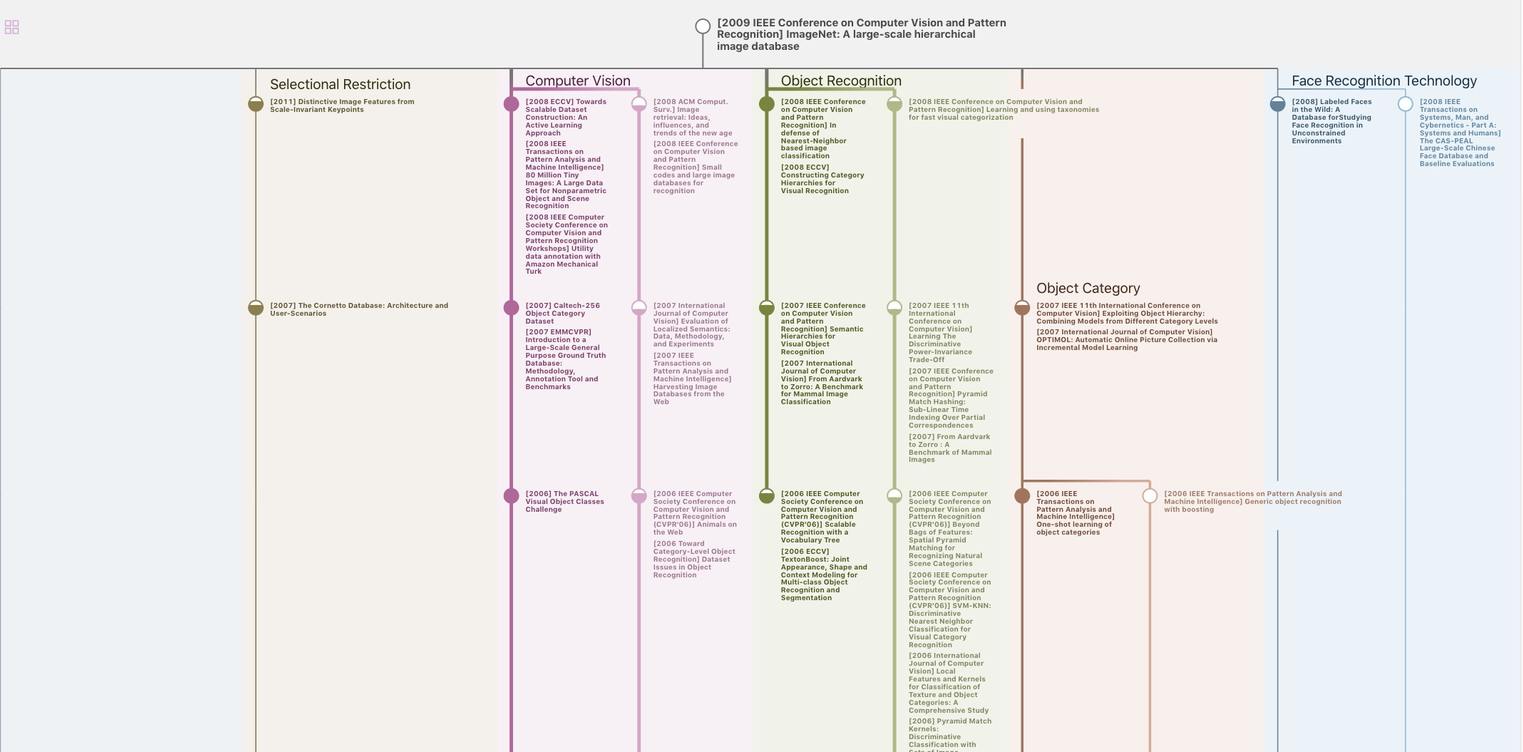
生成溯源树,研究论文发展脉络
Chat Paper
正在生成论文摘要