Domain Adaptation of Reinforcement Learning Agents based on Network Service Proximity
arxiv(2023)
摘要
The dynamic and evolutionary nature of service requirements in wireless networks has motivated the telecom industry to consider intelligent self-adapting Reinforcement Learning (RL) agents for controlling the growing portfolio of network services. Infusion of many new types of services is anticipated with future adoption of 6G networks, and sometimes these services will be defined by applications that are external to the network. An RL agent trained for managing the needs of a specific service type may not be ideal for managing a different service type without domain adaptation. We provide a simple heuristic for evaluating a measure of proximity between a new service and existing services, and show that the RL agent of the most proximal service rapidly adapts to the new service type through a well defined process of domain adaptation. Our approach enables a trained source policy to adapt to new situations with changed dynamics without retraining a new policy, thereby achieving significant computing and cost-effectiveness. Such domain adaptation techniques may soon provide a foundation for more generalized RL-based service management under the face of rapidly evolving service types.
更多查看译文
关键词
reinforcement learning agents,service proximity,adaptation
AI 理解论文
溯源树
样例
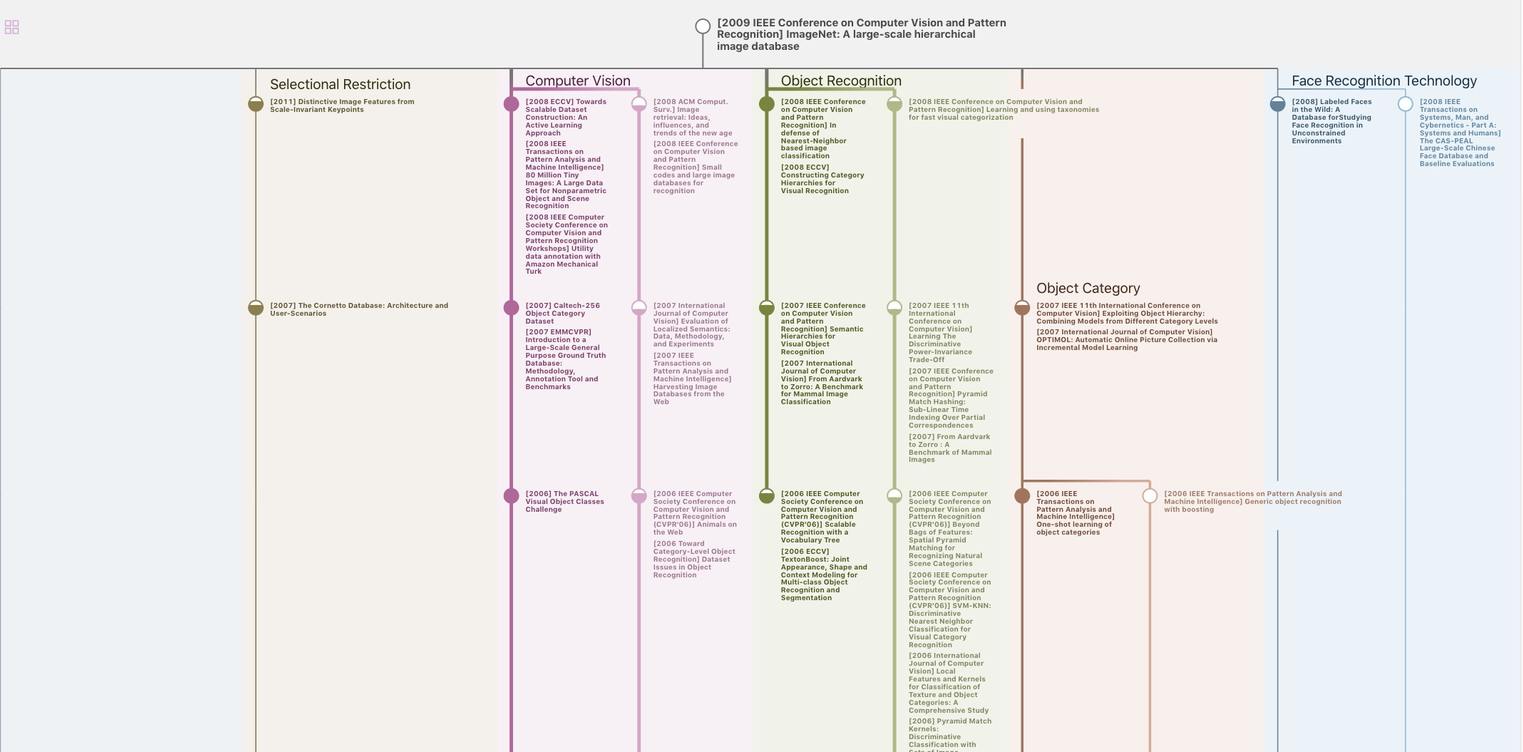
生成溯源树,研究论文发展脉络
Chat Paper
正在生成论文摘要