Learning curves for deep structured Gaussian feature models
NeurIPS(2023)
摘要
In recent years, significant attention in deep learning theory has been devoted to analyzing the generalization performance of models with multiple layers of Gaussian random features. However, few works have considered the effect of feature anisotropy; most assume that features are generated using independent and identically distributed Gaussian weights. Here, we derive learning curves for models with many layers of structured Gaussian features. We show that allowing correlations between the rows of the first layer of features can aid generalization, while structure in later layers is generally detrimental. Our results shed light on how weight structure affects generalization in a simple class of solvable models.
更多查看译文
关键词
feature models,curves,learning
AI 理解论文
溯源树
样例
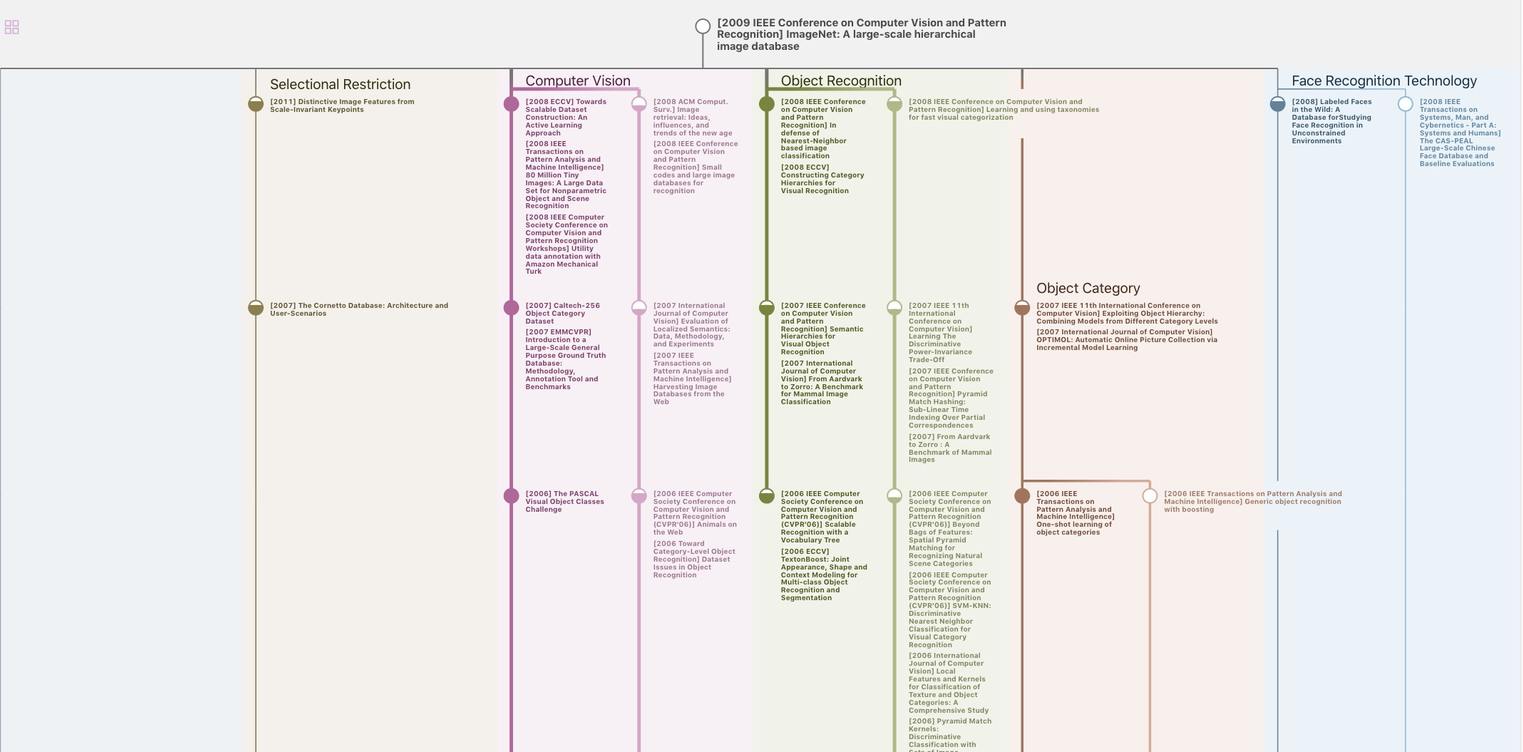
生成溯源树,研究论文发展脉络
Chat Paper
正在生成论文摘要