A Variational Approach to Mutual Information-Based Coordination for Multi-Agent Reinforcement Learning
arxiv(2023)
摘要
In this paper, we propose a new mutual information framework for multi-agent reinforcement learning to enable multiple agents to learn coordinated behaviors by regularizing the accumulated return with the simultaneous mutual information between multi-agent actions. By introducing a latent variable to induce nonzero mutual information between multi-agent actions and applying a variational bound, we derive a tractable lower bound on the considered MMI-regularized objective function. The derived tractable objective can be interpreted as maximum entropy reinforcement learning combined with uncertainty reduction of other agents actions. Applying policy iteration to maximize the derived lower bound, we propose a practical algorithm named variational maximum mutual information multi-agent actor-critic, which follows centralized learning with decentralized execution. We evaluated VM3-AC for several games requiring coordination, and numerical results show that VM3-AC outperforms other MARL algorithms in multi-agent tasks requiring high-quality coordination.
更多查看译文
关键词
reinforcement learning,coordination,variational approach,information-based,multi-agent
AI 理解论文
溯源树
样例
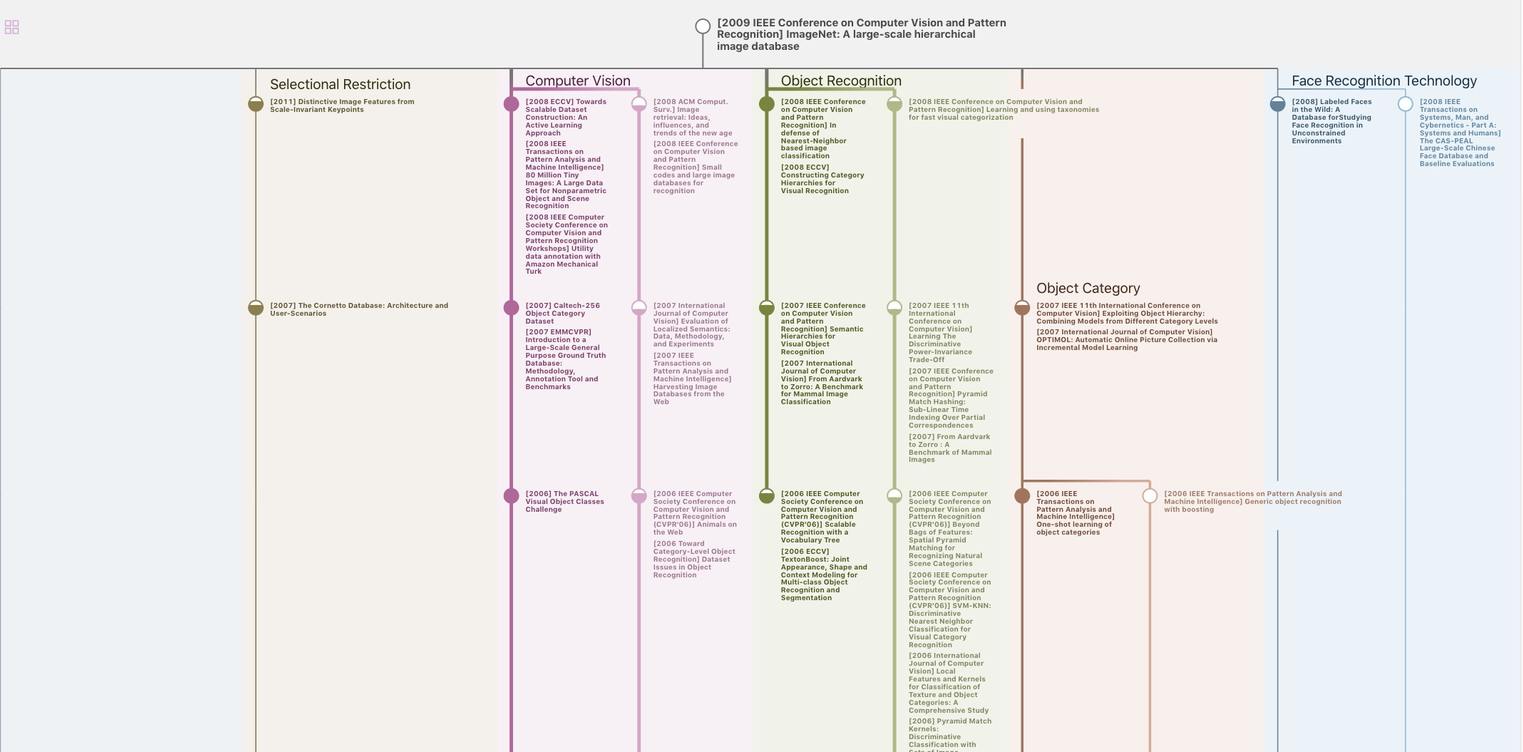
生成溯源树,研究论文发展脉络
Chat Paper
正在生成论文摘要