Single-Cell Multimodal Prediction via Transformers
PROCEEDINGS OF THE 32ND ACM INTERNATIONAL CONFERENCE ON INFORMATION AND KNOWLEDGE MANAGEMENT, CIKM 2023(2023)
摘要
The recent development of multimodal single-cell technology has made the possibility of acquiring multiple omics data from individual cells, thereby enabling a deeper understanding of cellular states and dynamics. Nevertheless, the proliferation of multimodal single-cell data also introduces tremendous challenges in modeling the complex interactions among different modalities. The recently advanced methods focus on constructing static interaction graphs and applying graph neural networks (GNNs) to learn from multimodal data. However, such static graphs can be suboptimal as they do not take advantage of the downstream task information; meanwhile GNNs also have some inherent limitations when deeply stacking GNN layers. To tackle these issues, in this work, we investigate how to leverage transformers for multimodal single-cell data in an end-to-end manner while exploiting downstream task information. In particular, we propose a scMoFormer framework which can readily incorporate external domain knowledge and model the interactions within each modality and cross modalities. Extensive experiments demonstrate that scMoFormer achieves superior performance on various benchmark datasets. Remarkably, scMoFormer won a Kaggle silver medal with the rank of 24/1221 (Top 2%) without ensemble in a NeurIPS 2022 competition(1). Our implementation is publicly available at Github(2).
更多查看译文
关键词
single-cell analysis,transformer,graph neural networks
AI 理解论文
溯源树
样例
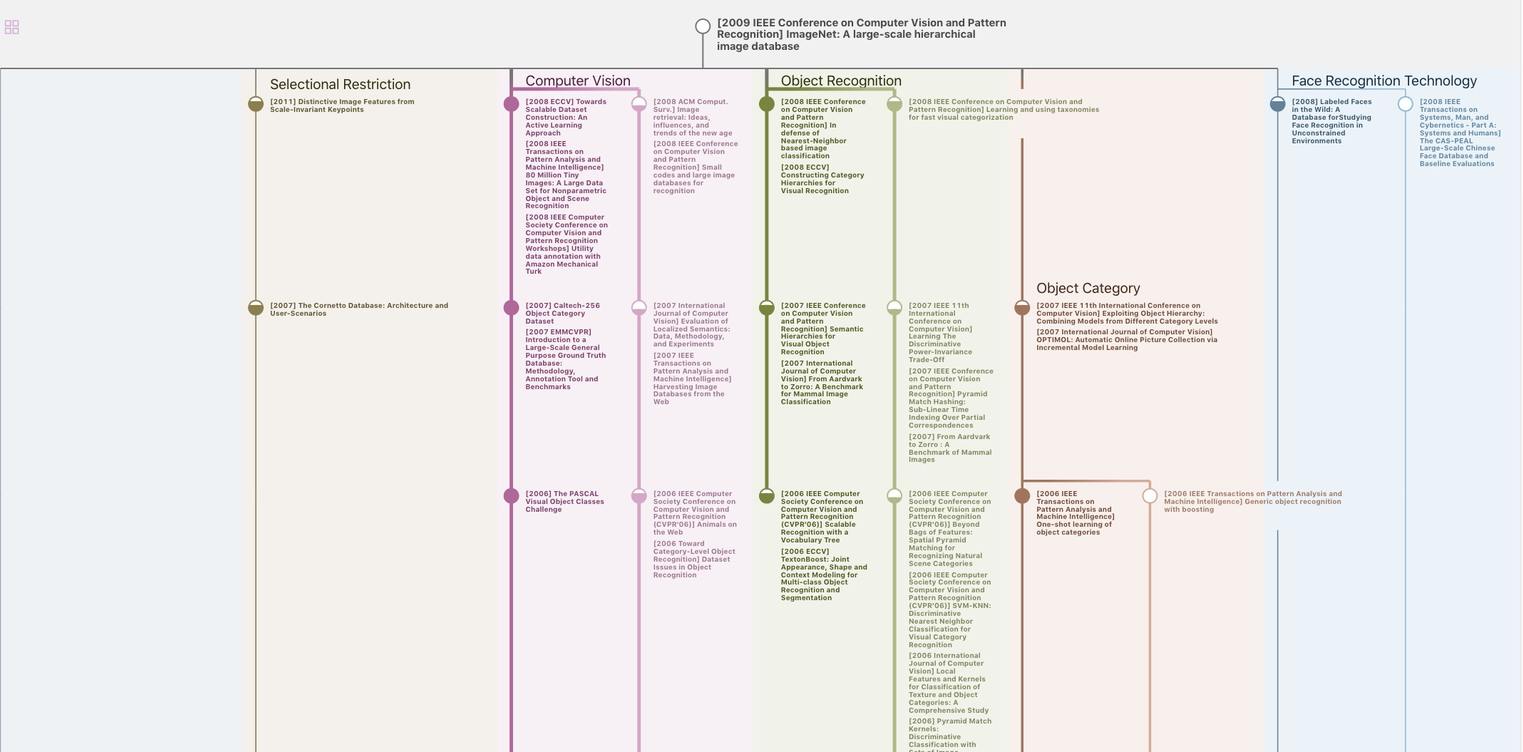
生成溯源树,研究论文发展脉络
Chat Paper
正在生成论文摘要