Optimality-Based Discretization Methods for the Global Optimization of Nonconvex Semi-Infinite Programs
arxiv(2023)
摘要
We use sensitivity analysis to design $\textit{optimality-based}$ discretization (cutting-plane) methods for the global optimization of nonconvex semi-infinite programs (SIPs). We begin by formulating the optimal discretization of SIPs as a max-min problem and propose variants that are more computationally tractable. We then use parametric sensitivity theory to design an efficient method for solving these max-min problems to local optimality and argue this yields valid discretizations without sacrificing global optimality guarantees. Finally, we formulate optimality-based $\textit{generalized}$ discretization of SIPs as max-min problems and design efficient local optimization algorithms to solve them approximately. Numerical experiments on test instances from the literature demonstrate that our new optimality-based discretization methods can significantly reduce the number of iterations for convergence relative to the classical feasibility-based method.
更多查看译文
关键词
global optimization,discretization methods,optimality-based,semi-infinite
AI 理解论文
溯源树
样例
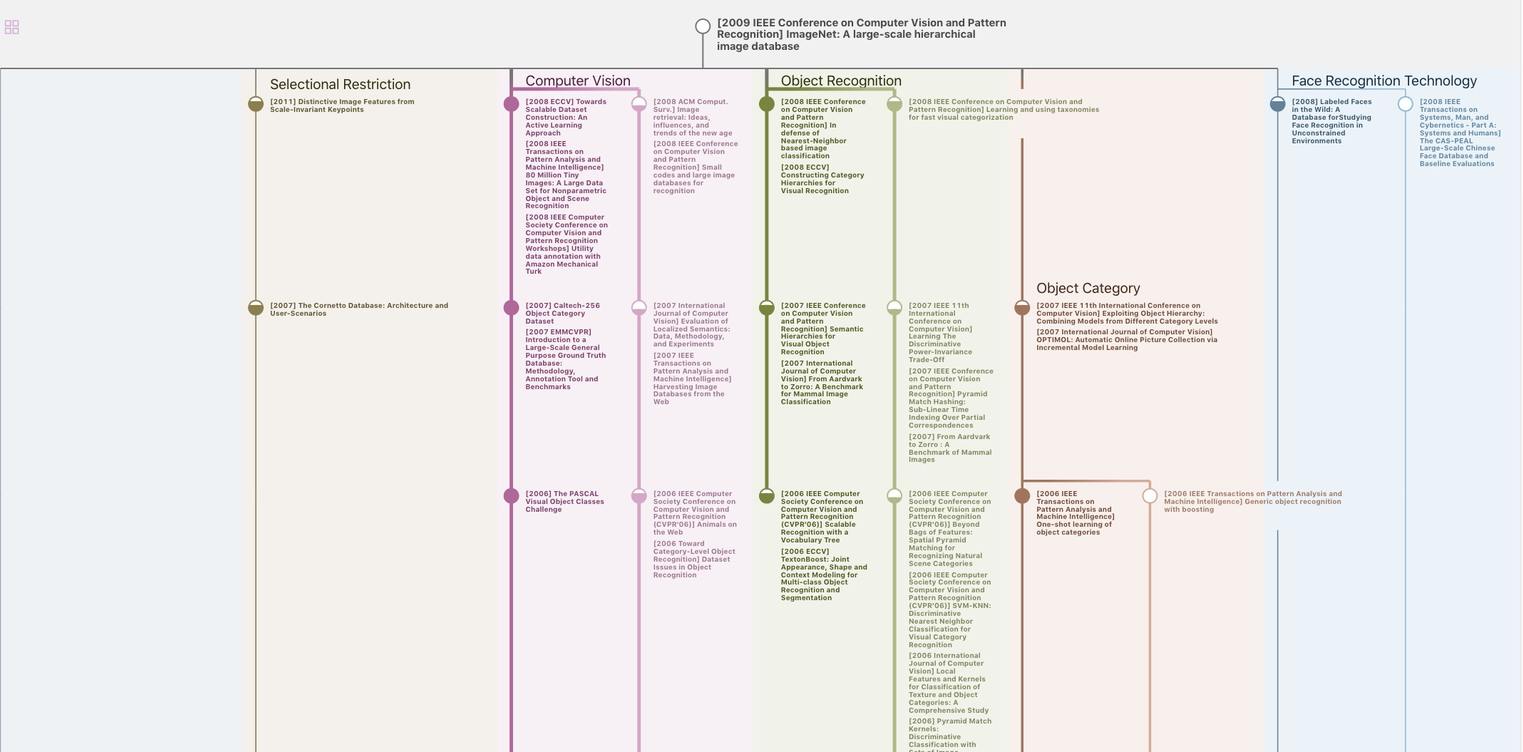
生成溯源树,研究论文发展脉络
Chat Paper
正在生成论文摘要