Self-Supervised Convolutional Visual Prompts
arxiv(2023)
摘要
Machine learning models often fail on out-of-distribution (OOD) samples. Visual prompts emerge as a light-weight adaptation method in input space for large-scale vision models. Existing vision prompts optimize a high-dimensional additive vector and require labeled data on training. However, we find this paradigm fails on test-time adaptation when labeled data is unavailable, where the high-dimensional visual prompt overfits to the self-supervised objective. We present convolutional visual prompts for test-time adaptation without labels. Our convolutional prompt is structured and requires fewer trainable parameters (less than 1 % parameters of standard visual prompts). Extensive experiments on a wide variety of OOD recognition tasks show that our approach is effective, improving robustness by up to 5.87 % over a number of large-scale model architectures.
更多查看译文
AI 理解论文
溯源树
样例
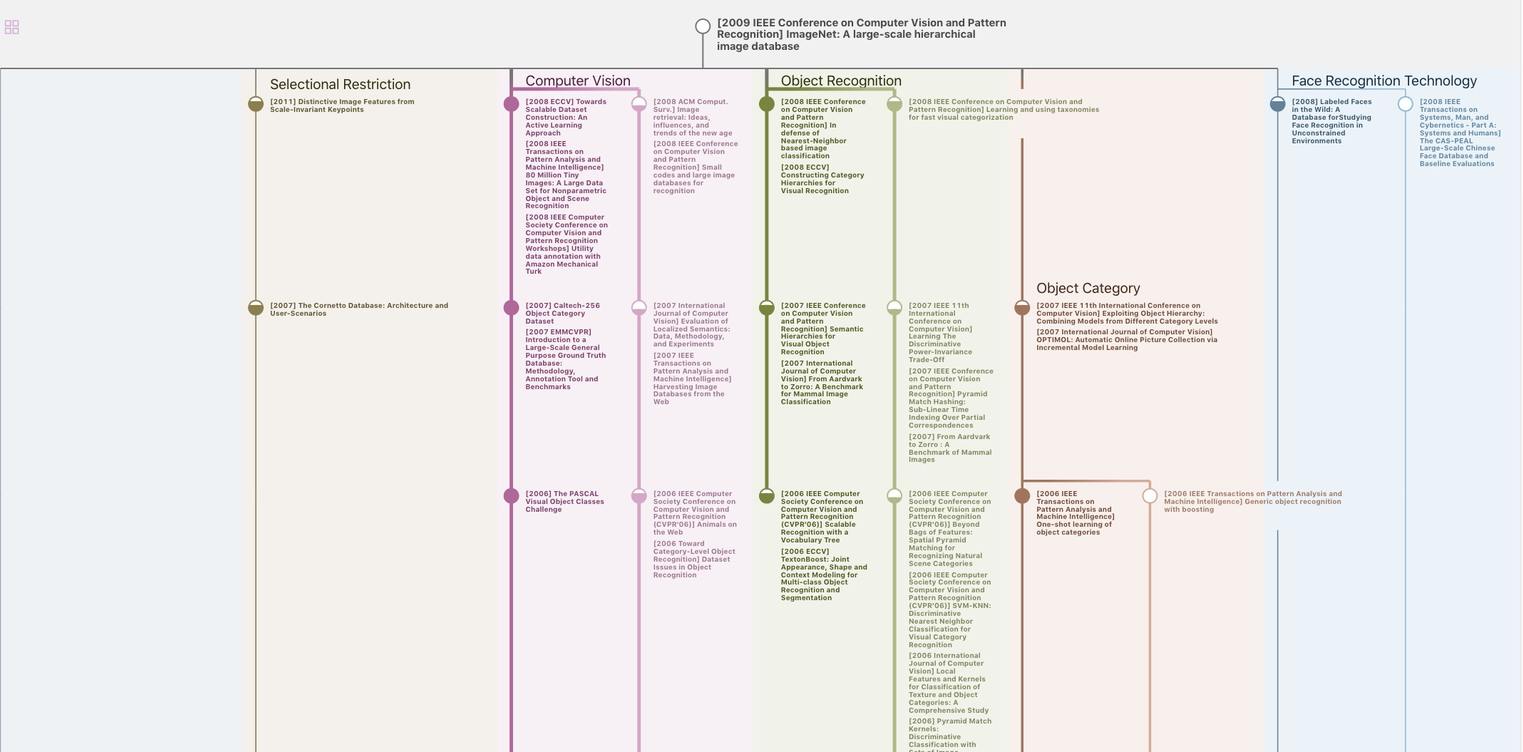
生成溯源树,研究论文发展脉络
Chat Paper
正在生成论文摘要