CXLSeg Dataset: Chest X-ray with Lung Segmentation
2023 International Conference On Cyber Management And Engineering (CyMaEn)(2023)
Abstract
With the advancement of robust deep learning techniques, a significant number of applications pertaining to biomedical research and clinical practice can be noticed within the computer vision domain. Radiologists use Chest X-Ray (CXR) images, prominent among medical imaging, to diagnose and treat diseases. Proper anatomical segmentation of CXR images is increasingly valuable as a critical image pre-processing step as well as in interpreting the deep learning models since it isolates the necessary area by extracting the region of interest. This paper proposes a CXR-segmented dataset based on the MIMIC-CXR dataset. A total of 243,324 frontal views of CXR images with segmented masks for each image are available in the dataset. A U-Net model with spatial attention (SA-UNet) architecture is utilized for segmenting the CXR images after a comparative analysis of different U-Net variants. The SA-UNet model achieved a 96.80% in dice similarity coefficient and 91.97% in IoU for lung segmentation. This study has shown that the visual feature extraction process is optimized using segmented CXR radiographs of CXLSeg instead of original MIMIC-CXR images by a significant margin.
MoreTranslated text
Key words
medical image segmentation,MIMIC-CXR,U-NET,SA-UNet,Shenzhen,Montgomery
AI Read Science
Must-Reading Tree
Example
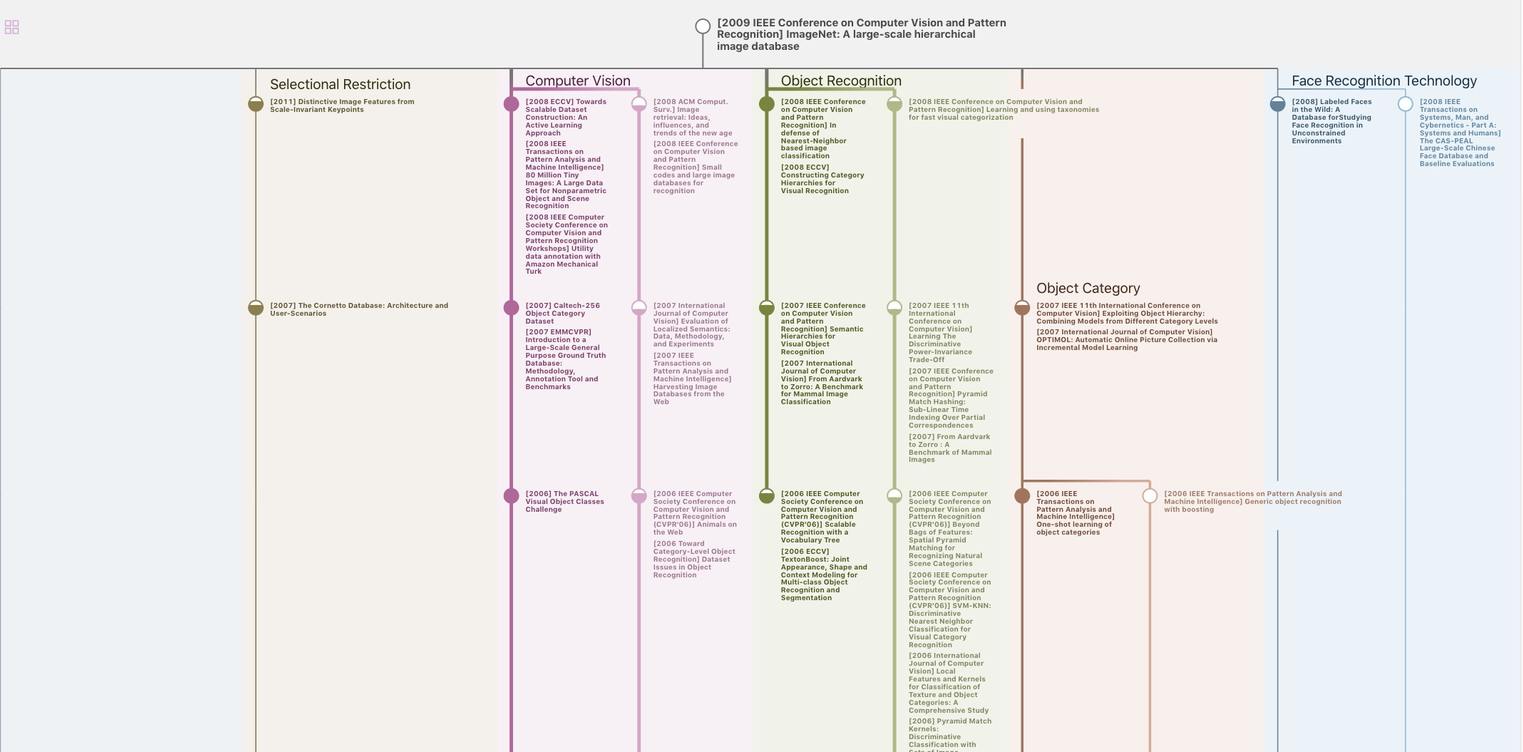
Generate MRT to find the research sequence of this paper
Chat Paper
Summary is being generated by the instructions you defined