Evaluating Attention in Convolutional Neural Networks for Blended Images
2022 IEEE 5th International Conference on Image Processing Applications and Systems (IPAS)(2022)
摘要
examine how attention mechanisms in the human brain work. They are particularly suited for this research area, as a subject needs to focus on particular features in an image to be able to classify superimposed objects. As Convolutional Neural Networks (CNNs) take some inspiration from the mammalian visual system - such as the hierarchical structure where different levels of abstraction are processed on different network layers we examine how CNNs perform on this task. More specifically, we evaluate the performance of four popular CNN architectures (ResNet18, ResNet50, CORnet-Z, and Inception V3) on the classification of objects in blended images. Since humans can rather easily solve this task by applying object-based attention, we also augment all architectures with a multi-headed self-attention mechanism to examine its effect on performance. Lastly, we analyse if there is a correlation between the similarity of a network architecture's structure to the human visual system and its ability to correctly classify objects in blended images. Our findings showed that adding a self-attention mechanism reliably increases the similarity to the V4 area of the human ventral stream, an area where attention has a large influence on the processing of visual stimuli.
更多查看译文
关键词
image classification,blended images,biological inspiration
AI 理解论文
溯源树
样例
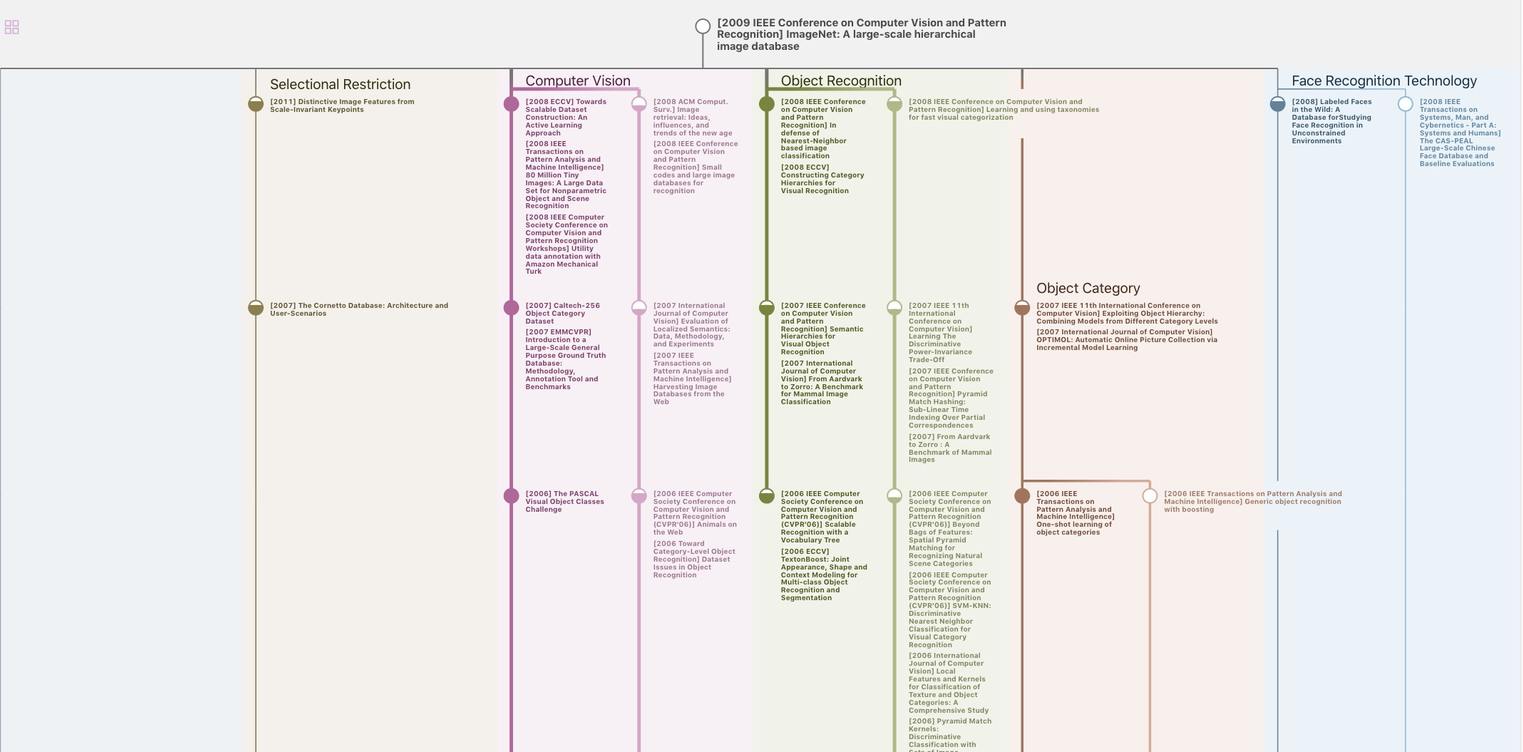
生成溯源树,研究论文发展脉络
Chat Paper
正在生成论文摘要