Monocular Depth Estimation using Diffusion Models
arxiv(2023)
摘要
We formulate monocular depth estimation using denoising diffusion models, inspired by their recent successes in high fidelity image generation. To that end, we introduce innovations to address problems arising due to noisy, incomplete depth maps in training data, including step-unrolled denoising diffusion, an $L_1$ loss, and depth infilling during training. To cope with the limited availability of data for supervised training, we leverage pre-training on self-supervised image-to-image translation tasks. Despite the simplicity of the approach, with a generic loss and architecture, our DepthGen model achieves SOTA performance on the indoor NYU dataset, and near SOTA results on the outdoor KITTI dataset. Further, with a multimodal posterior, DepthGen naturally represents depth ambiguity (e.g., from transparent surfaces), and its zero-shot performance combined with depth imputation, enable a simple but effective text-to-3D pipeline. Project page: https://depth-gen.github.io
更多查看译文
关键词
monocular depth estimation,diffusion
AI 理解论文
溯源树
样例
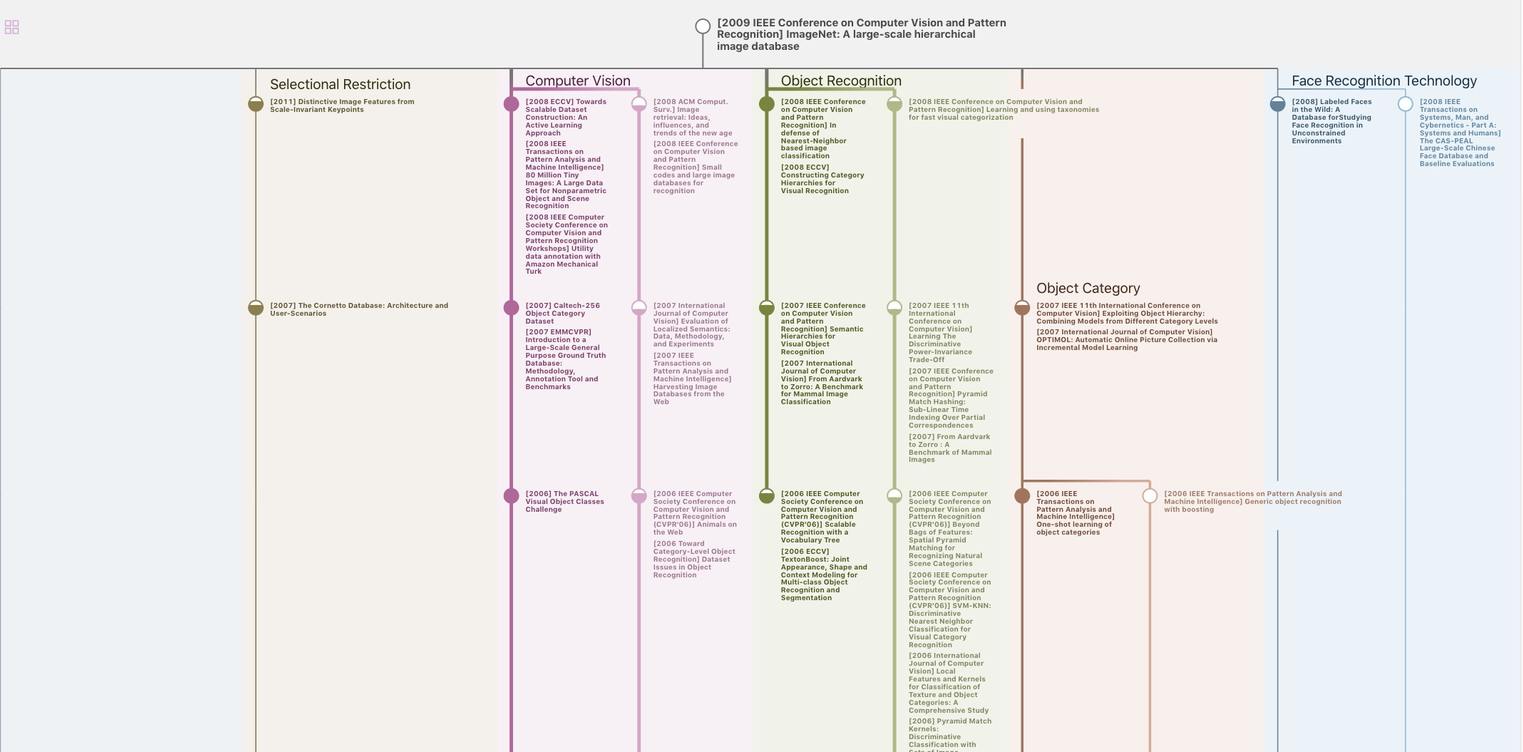
生成溯源树,研究论文发展脉络
Chat Paper
正在生成论文摘要