TransAdapt: A Transformative Framework for Online Test Time Adaptive Semantic Segmentation
ICASSP 2023 - 2023 IEEE International Conference on Acoustics, Speech and Signal Processing (ICASSP)(2023)
Abstract
Test-time adaptive (TTA) semantic segmentation adapts a source pre-trained image semantic segmentation model to unlabeled batches of target domain test images, different from real-world, where samples arrive one-by-one in an online fashion. To tackle online settings, we propose TransAdapt, a framework that uses transformer and input transformations to improve segmentation performance. Specifically, we pre-train a transformer-based module on a segmentation network that transforms unsupervised segmentation output to a more reliable supervised output, without requiring test-time online training. To also facilitate test-time adaptation, we propose an unsupervised loss based on the transformed input that enforces the model to be invariant and equivariant to photometric and geometric perturbations, respectively. Overall, our framework produces higher quality segmentation masks with up to 17.6% and 2.8% mIOU improvement over no-adaptation and competitive baselines, respectively.
MoreTranslated text
Key words
Test Time Adaptation,Online Learning,Transformer,Consistency,Semantic Segmentation
AI Read Science
Must-Reading Tree
Example
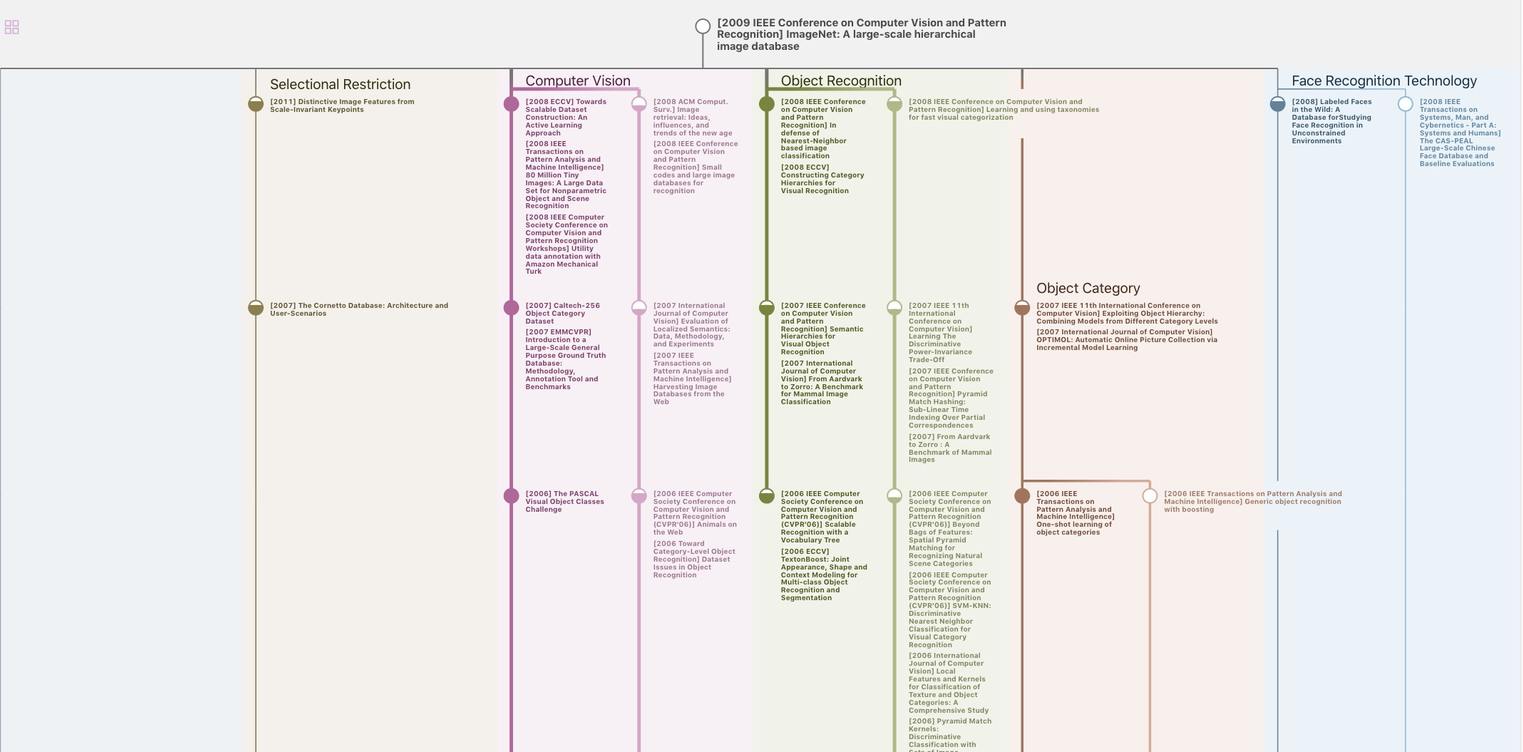
Generate MRT to find the research sequence of this paper
Chat Paper
Summary is being generated by the instructions you defined