Sampled Transformer for Point Sets
ICLR 2023(2023)
摘要
The sparse transformer can reduce the computational complexity of the self-attention layers to $O(n)$, whilst still being a universal approximator of continuous sequence-to-sequence functions. However, this permutation variant operation is not appropriate for direct application to sets. In this paper, we proposed an $O(n)$ complexity sampled transformer that can process point set elements directly without any additional inductive bias. Our sampled transformer introduces random element sampling, which randomly splits point sets into subsets, followed by applying a shared Hamiltonian self-attention mechanism to each subset. The overall attention mechanism can be viewed as a Hamiltonian cycle in the complete attention graph, and the permutation of point set elements is equivalent to randomly sampling Hamiltonian cycles. This mechanism implements a Monte Carlo simulation of the $O(n^2)$ dense attention connections. We show that it is a universal approximator for continuous set-to-set functions. Experimental results on point-clouds show comparable or better accuracy with significantly reduced computational complexity compared to the dense transformer or alternative sparse attention schemes.
更多查看译文
关键词
transformer
AI 理解论文
溯源树
样例
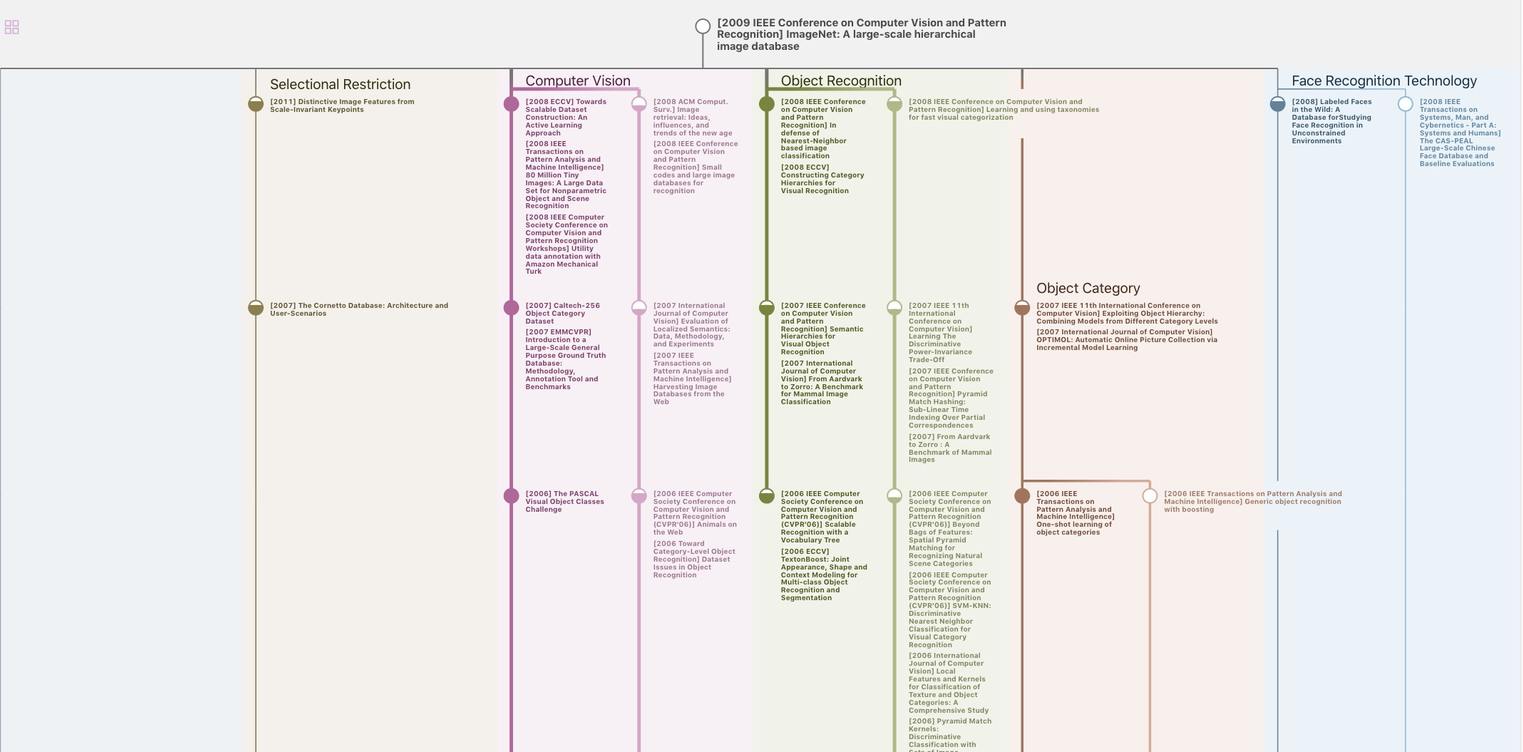
生成溯源树,研究论文发展脉络
Chat Paper
正在生成论文摘要