Approximately Optimal Domain Adaptation with Fisher's Linear Discriminant
MATHEMATICS(2024)
关键词
statistical learning,domain adaptation,transfer learning,physiological prediction,linear classifiers
AI 理解论文
溯源树
样例
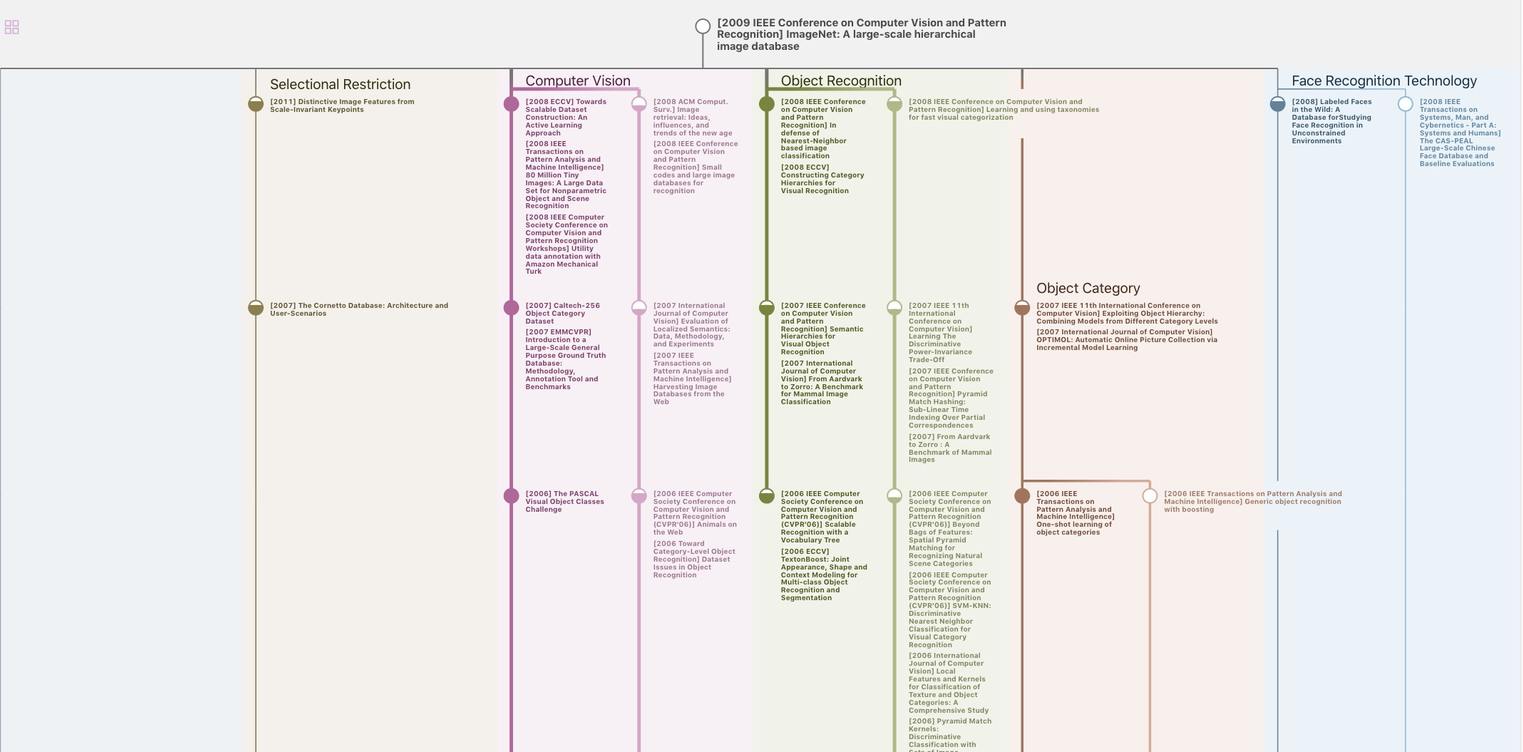
生成溯源树,研究论文发展脉络
Chat Paper
正在生成论文摘要
MATHEMATICS(2024)