A Dataset for Learning Graph Representations to Predict Customer Returns in Fashion Retail
RECOMMENDER SYSTEMS IN FASHION AND RETAIL(2023)
摘要
We present a novel dataset collected by ASOS (a major online fashion retailer) to address the challenge of predicting customer returns in a fashion retail ecosystem. With the release of this substantial dataset, we hope to motivate further collaboration between research communities and the fashion industry. We first explore the structure of this dataset with a focus on the application of Graph Representation Learning in order to exploit the natural data structure and provide statistical insights into particular features within the data. In addition to this, we show examples of a return prediction classification task with a selection of baseline models (i.e. with no intermediate representation learning step) and a graph representation based model. We show that in a downstream return prediction classification task, an F1-score of 0.792 can be found using a Graph Neural Network (GNN), improving upon other models discussed in this work. Alongside this increased F1-score, we also present a lower cross-entropy loss by recasting the data into a graph structure, indicating more robust predictions from a GNN-based solution. These results provide evidence that GNNs could provide more impactful and usable classifications than other baseline models on the presented dataset, and with this motivation, we hope to encourage further research into graph-based approaches using the ASOS GraphReturns dataset.
更多查看译文
关键词
Graph representation learning,Neural message passing,Edge classification,Fashion retail dataset,Customer return prediction
AI 理解论文
溯源树
样例
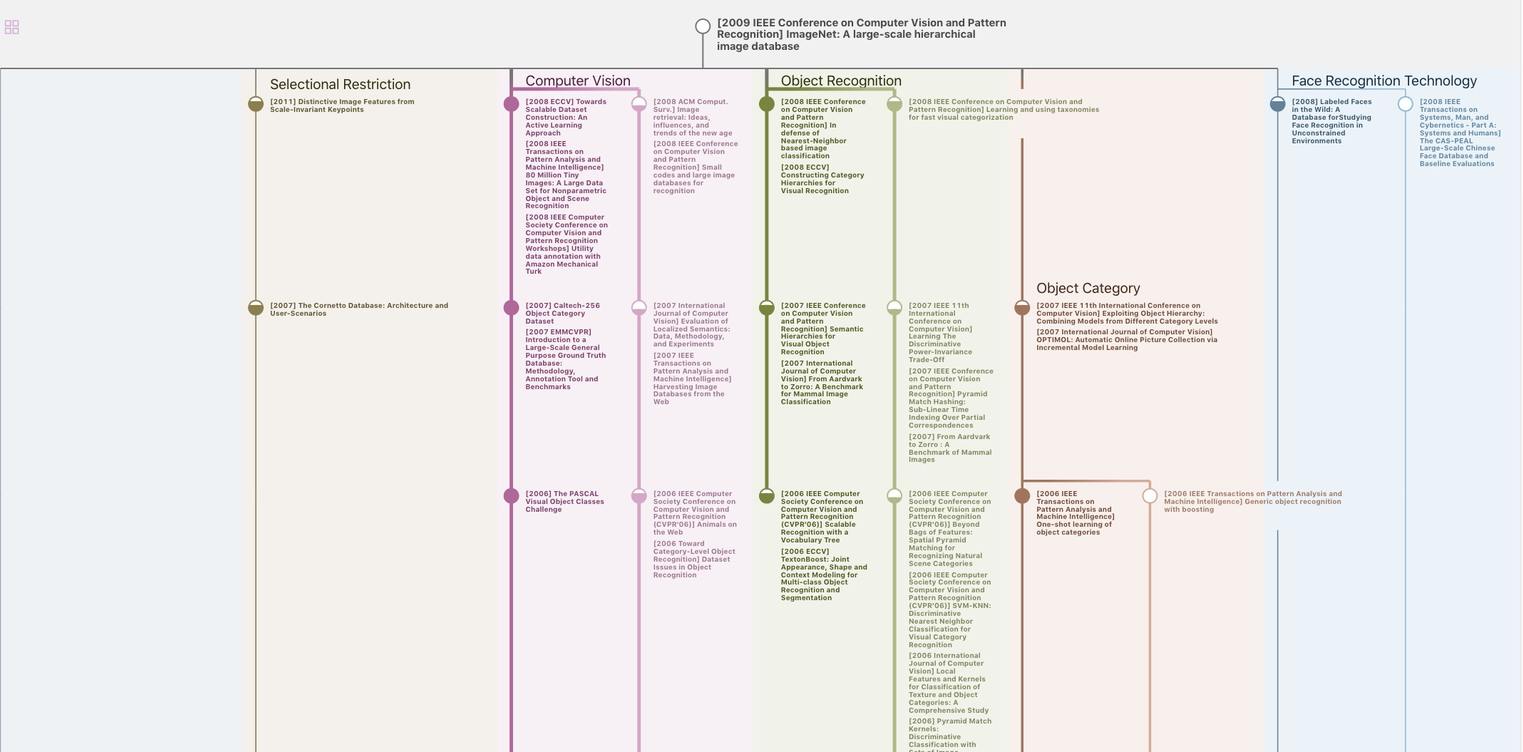
生成溯源树,研究论文发展脉络
Chat Paper
正在生成论文摘要