Extraction of clinical phenotypes for Alzheimer's disease dementia from clinical notes using natural language processing
JAMIA open(2023)
摘要
Lay Summary There is much interest in understanding risk factors and predicting the clinical trajectory of Alzheimer disease (AD) dementia, for which there is substantial variability in the rate of clinical decline. Electronic health record data collected over the course of routine medical care contains vast amounts of patient data that could be useful for this purpose. In our dataset, we found that the richest source of AD-relevant information is the clinical notes. However, the unstructured nature of the clinical note poses a significant challenge to extracting information in a format useful for predictive analyses. Natural language processing was used to extract information from clinical notes relevant to the clinical care of an AD patient, and the success of this method was determined by comparing the accuracy of the information extracted to the information manually annotated by 2 AD clinical experts. The 2 clinical experts generally agreed, and our method performed well compared to their annotations. Accurate information retrieval from unstructured clinical notes will improve understanding of a patient's medical history and overall health, and thus the ability to predict AD risk and progression. Objectives There is much interest in utilizing clinical data for developing prediction models for Alzheimer's disease (AD) risk, progression, and outcomes. Existing studies have mostly utilized curated research registries, image analysis, and structured electronic health record (EHR) data. However, much critical information resides in relatively inaccessible unstructured clinical notes within the EHR. Materials and Methods We developed a natural language processing (NLP)-based pipeline to extract AD-related clinical phenotypes, documenting strategies for success and assessing the utility of mining unstructured clinical notes. We evaluated the pipeline against gold-standard manual annotations performed by 2 clinical dementia experts for AD-related clinical phenotypes including medical comorbidities, biomarkers, neurobehavioral test scores, behavioral indicators of cognitive decline, family history, and neuroimaging findings. Results Documentation rates for each phenotype varied in the structured versus unstructured EHR. Interannotator agreement was high (Cohen's kappa = 0.72-1) and positively correlated with the NLP-based phenotype extraction pipeline's performance (average F1-score = 0.65-0.99) for each phenotype. Discussion We developed an automated NLP-based pipeline to extract informative phenotypes that may improve the performance of eventual machine learning predictive models for AD. In the process, we examined documentation practices for each phenotype relevant to the care of AD patients and identified factors for success. Conclusion Success of our NLP-based phenotype extraction pipeline depended on domain-specific knowledge and focus on a specific clinical domain instead of maximizing generalizability.
更多查看译文
关键词
natural language processing,Alzheimer's disease,electronic health records,routinely collected health data,information retrieval
AI 理解论文
溯源树
样例
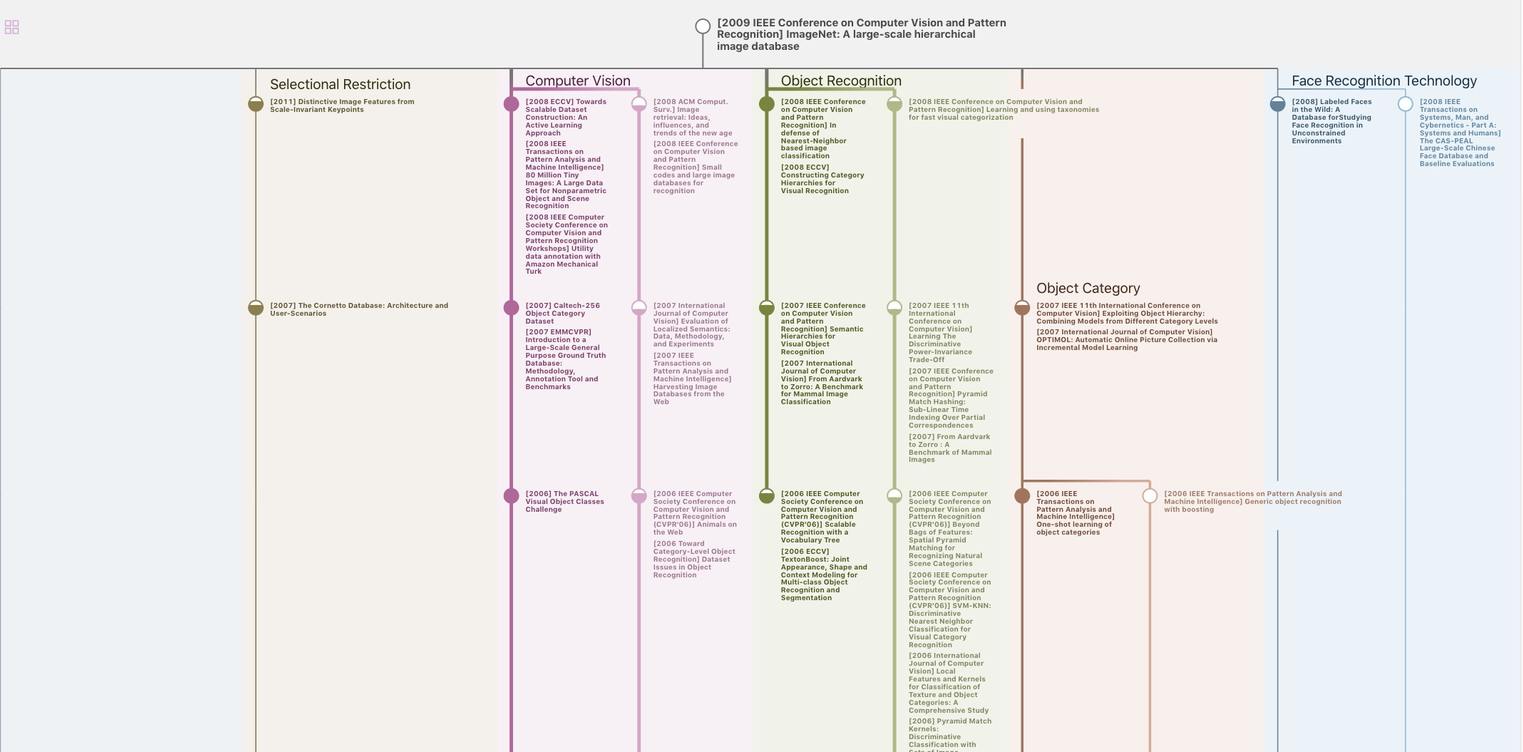
生成溯源树,研究论文发展脉络
Chat Paper
正在生成论文摘要