Effective Connectivity and Bias Entropy Improve Prediction of Dynamical Regime in Automata Networks
Entropy (Basel, Switzerland)(2023)
Abstract
Biomolecular network dynamics are thought to operate near the critical boundary between ordered and disordered regimes, where large perturbations to a small set of elements neither die out nor spread on average. A biomolecular automaton (e.g., gene, protein) typically has high regulatory redundancy, where small subsets of regulators determine activation via collective canalization. Previous work has shown that effective connectivity, a measure of collective canalization, leads to improved dynamical regime prediction for homogeneous automata networks. We expand this by (i) studying random Boolean networks (RBNs) with heterogeneous in-degree distributions, (ii) considering additional experimentally validated automata network models of biomolecular processes, and (iii) considering new measures of heterogeneity in automata network logic. We found that effective connectivity improves dynamical regime prediction in the models considered; in RBNs, combining effective connectivity with bias entropy further improves the prediction. Our work yields a new understanding of criticality in biomolecular networks that accounts for collective canalization, redundancy, and heterogeneity in the connectivity and logic of their automata models. The strong link we demonstrate between criticality and regulatory redundancy provides a means to modulate the dynamical regime of biochemical networks.
MoreTranslated text
Key words
random Boolean networks,criticality,perturbation spreading,biomolecular networks,Derrida coefficient
AI Read Science
Must-Reading Tree
Example
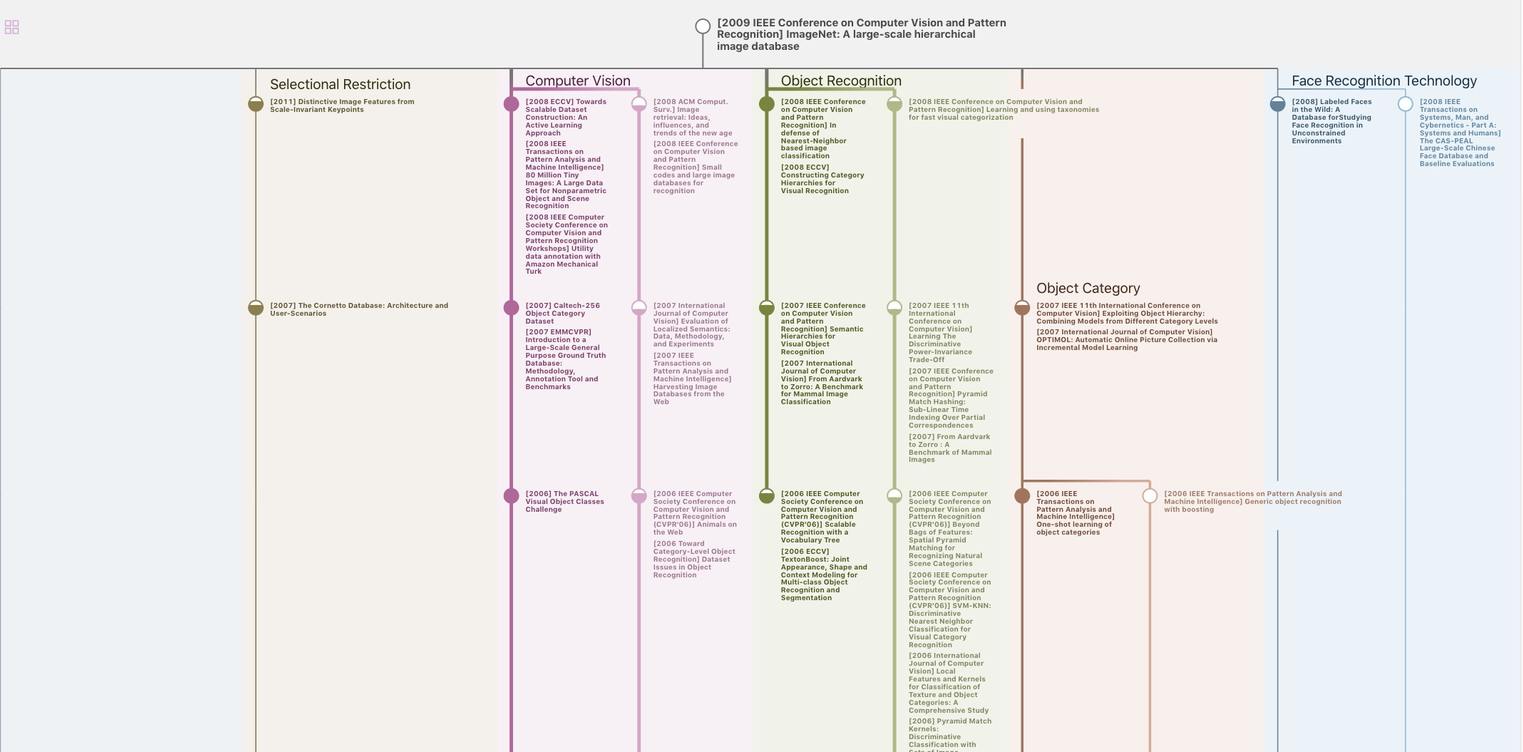
Generate MRT to find the research sequence of this paper
Chat Paper
Summary is being generated by the instructions you defined