Soft labelling for semantic segmentation: Bringing coherence to label down-sampling
arXiv (Cornell University)(2023)
摘要
In semantic segmentation, training data down-sampling is commonly performed
due to limited resources, the need to adapt image size to the model input, or
improve data augmentation. This down-sampling typically employs different
strategies for the image data and the annotated labels. Such discrepancy leads
to mismatches between the down-sampled color and label images. Hence, the
training performance significantly decreases as the down-sampling factor
increases. In this paper, we bring together the down-sampling strategies for
the image data and the training labels. To that aim, we propose a novel
framework for label down-sampling via soft-labeling that better conserves label
information after down-sampling. Therefore, fully aligning soft-labels with
image data to keep the distribution of the sampled pixels. This proposal also
produces reliable annotations for under-represented semantic classes.
Altogether, it allows training competitive models at lower resolutions.
Experiments show that the proposal outperforms other down-sampling strategies.
Moreover, state-of-the-art performance is achieved for reference benchmarks,
but employing significantly less computational resources than foremost
approaches. This proposal enables competitive research for semantic
segmentation under resource constraints.
更多查看译文
关键词
semantic segmentation,soft labelling,coherence,down-sampling
AI 理解论文
溯源树
样例
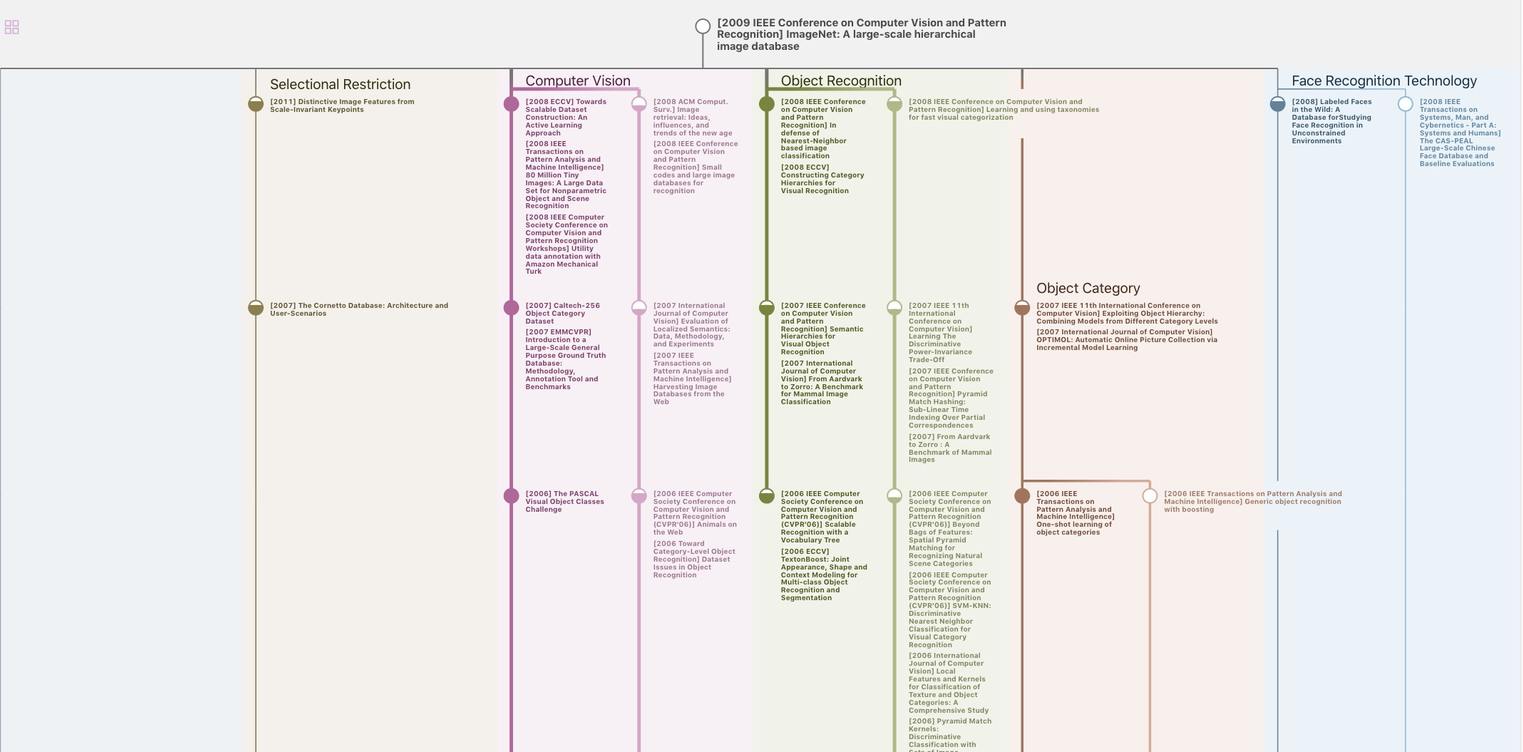
生成溯源树,研究论文发展脉络
Chat Paper
正在生成论文摘要